Longitudinally Consistent Registration and Parcellation of Cortical Surfaces Using Semi-Supervised Learning
Medical Image Analysis(2024)
摘要
Temporally consistent and accurate registration and parcellation of longitudinal cortical surfaces is of great importance in studying longitudinal morphological and functional changes of human brains. However, most existing methods are developed for registration or parcellation of a single cortical surface. When applying to longitudinal studies, these methods independently register/parcellate each surface from longitudinal scans, thus often generating longitudinally inconsistent and inaccurate results, especially in small or ambiguous cortical regions. Essentially, longitudinal cortical surface registration and parcellation are highly correlated tasks with inherently shared constraints on both spatial and temporal feature representations, which are unfortunately ignored in existing methods. To this end, we unprecedentedly propose a novel semi-supervised learning framework to exploit these inherent relationships from limited labeled data and extensive unlabeled data for more robust and consistent registration and parcellation of longitudinal cortical surfaces. Our method utilizes the spherical topology characteristic of cortical surfaces. It employs a spherical network to function as an encoder, which extracts high-level cortical features. Subsequently, we build two specialized decoders dedicated to the tasks of registration and parcellation, respectively. To extract more meaningful spatial features, we design a novel parcellation map similarity loss to utilize the relationship between registration and parcellation tasks, i.e., the parcellation map warped by the deformation field in registration should match the atlas parcellation map, thereby providing extra supervision for the registration task and augmented data for parcellation task by warping the atlas parcellation map to unlabeled surfaces. To enable temporally more consistent feature representation, we additionally enforce longitudinal consistency among longitudinal surfaces after registering them together using their concatenated features. Experiments on two longitudinal datasets of infants and adults have shown that our method achieves significant improvements on both registration/parcellation accuracy and longitudinal consistency compared to existing methods, especially in small and challenging cortical regions.
更多查看译文
关键词
Cortical Surface Registration,Parcellation,Spherical Network,Longitudinal Analysis
AI 理解论文
溯源树
样例
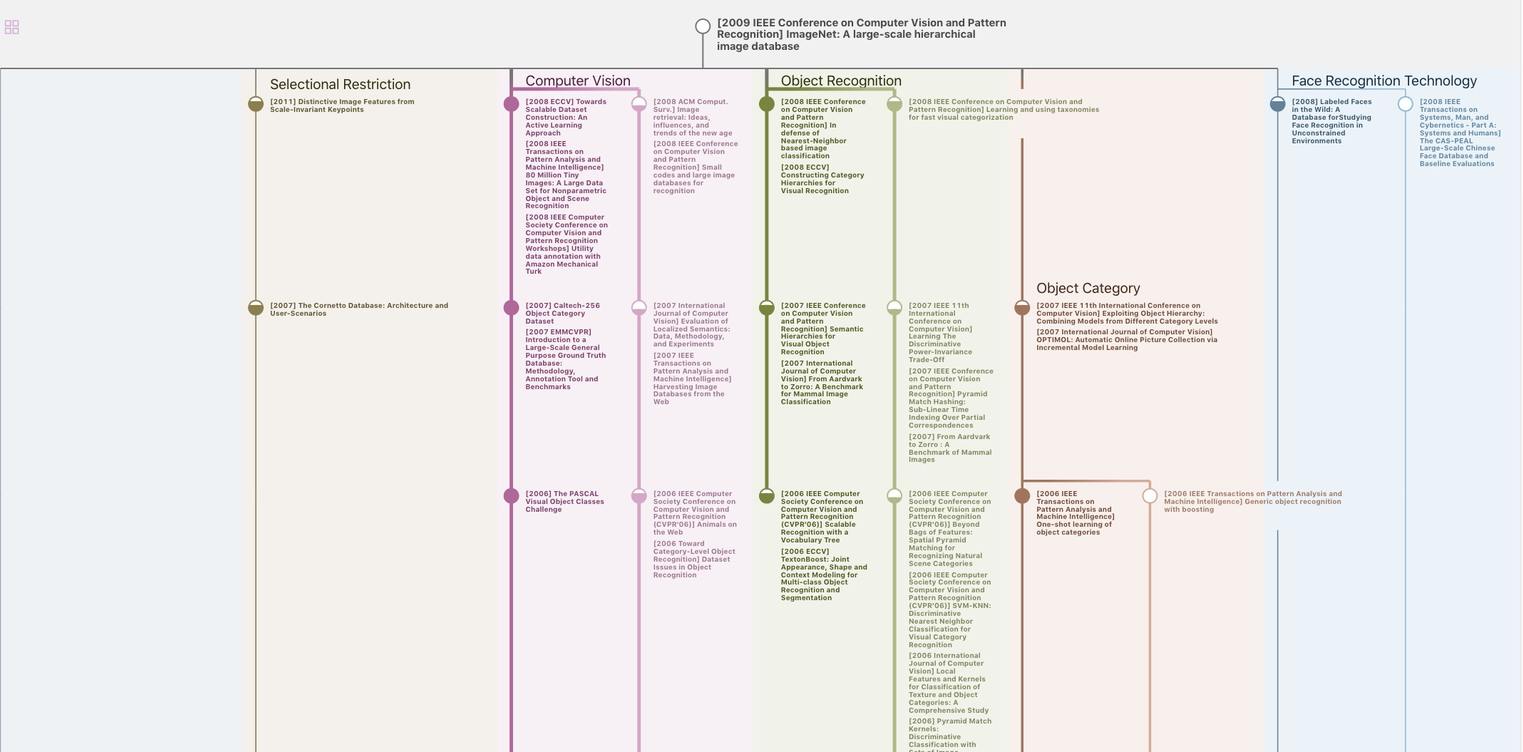
生成溯源树,研究论文发展脉络
Chat Paper
正在生成论文摘要