Drug repositioning based on tripartite cross-network embedding and graph convolutional network
Expert Systems with Applications(2024)
摘要
Drug-disease association prediction is an important part of drug discovery, which can help researchers uncover potential drug candidates and disease targets more accurately to deal with the drawbacks of inefficiency and high cost of traditional methods. In contrast, past drug-disease prediction processes often overlooked the associations between drugs and side effects and the impact of proteins on diseases. These issues hindered models from fully learning the potential information within the network. Hence, a method capable of comprehensively learning from multi-source heterogeneous information and extracting high-level features of biological associations is needed. To overcome these challenges, proposes a drug repositioning method based on a tripartite cross-network embedding graph convolutional network, called TGCNDR, to predict the associations between drugs and diseases. Firstly, we construct a tripartite cross-network of drug-disease associations, drug-protein associations, and drug-side effect associations. TGCNDR employs graph convolutional networks to capture information from different nodes to learn drug embedding. Subsequently, TGCNDR uses anchor links for knowledge transfer, and different networks use the Self-attention mechanism to represent drug importance. Experiments on benchmark datasets demonstrate that TGCNDR exhibits good performance(the average AUROC is 0.922, the average AUPR is 0.916, and the average F1-score is 0.860) compared with state-of-the-art methods. In the case study, we perform molecular docking experiments on the predicted drug candidates and identify newly approved drugs for osteosarcoma, which suggests that TGCNDR is an effective tool for drug repositioning.
更多查看译文
关键词
Drug repositioning,Tripartite cross-network embedding,Drug-disease association prediction,Graph convolutional network
AI 理解论文
溯源树
样例
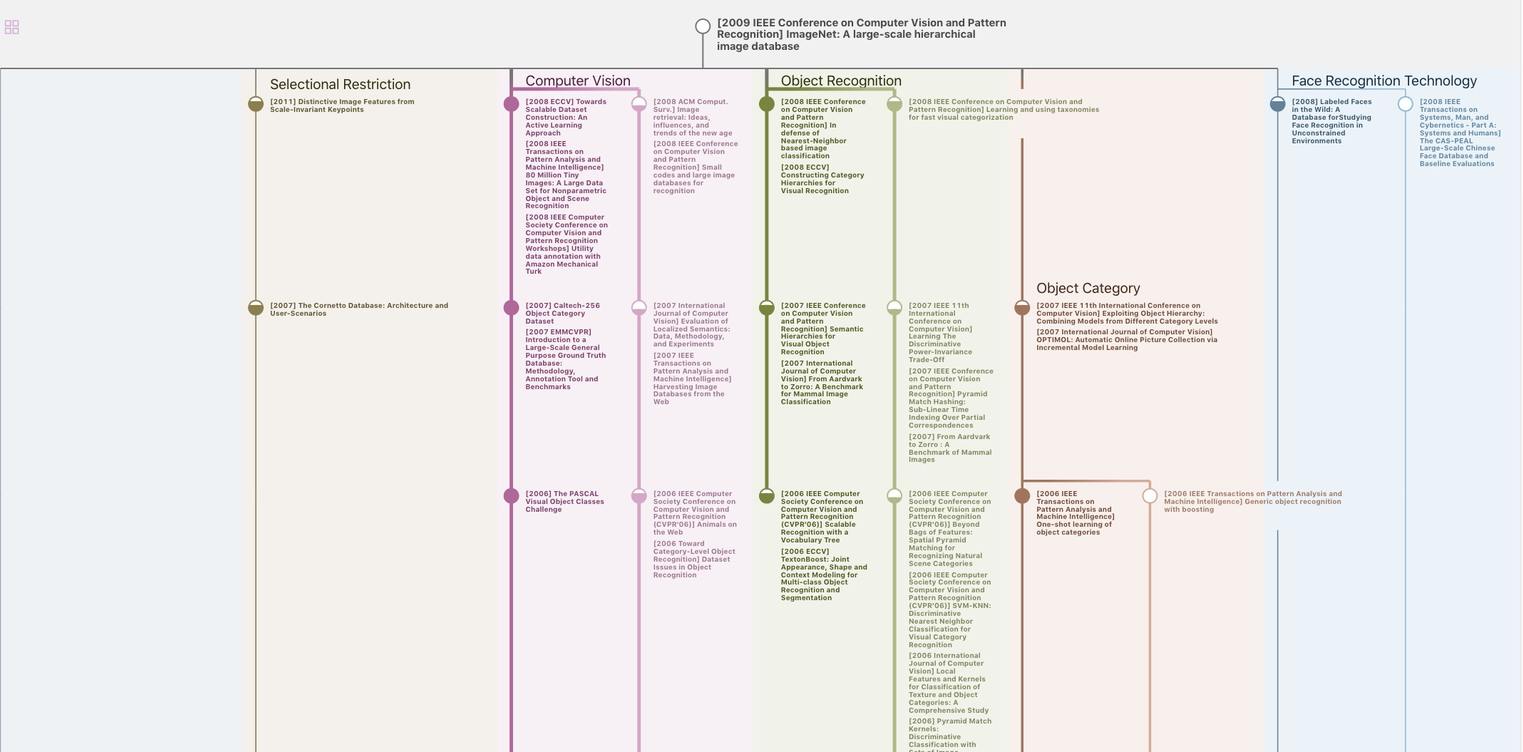
生成溯源树,研究论文发展脉络
Chat Paper
正在生成论文摘要