Forecasting personal heat strain under extremely hot environments: Utilizing feature importance in machine learning
Engineering Applications of Artificial Intelligence(2024)
摘要
Since the frequency and intensity of heatwaves are expected to increase due to global warming, it is crucial to establish a simplified approach to preventing personal heat-related illnesses as occupational hazard under extremely hot environments, by taking into account individual differences in heat strain. In light of this, this study proposed a simplified forecast model for personal heat strain under extremely hot environments by utilizing feature importance in machine learning, focused on enhancing its field applicability. Using the 1417 records gathered from the experiments under extremely hot conditions, the simplified model was developed by utilizing four types of machine learning algorithms (i.e., random forest, extreme gradient boosting, support vector regression, and multi-layer perceptron). As a result, the proposed simplified models with four principal features (i.e., dry-bulb temperature, radiant temperature, relative humidity, and percentage body fat) were found to be the most reliable, resulting in a small difference of 0.047 °C compared to the reference model. Since the principal biometric features can be easily measured by a simple and low-cost body composition test (but not with the physical measurement of personal core body temperature) before they are put into the field, the proposed approach will aid in providing more systematic and efficient management system for proactively preventing personal heat-related illnesses. Furthermore, it is expected that the field applicability of the proposed approach can be continuously enhanced by utilizing the accumulated big data gathered from the field-based living-lab projects over a lengthy period.
更多查看译文
关键词
Personal heat strain,Extremely hot environments,Forecasting model,Principal biometric features,Machine learning algorithm,Field applicability
AI 理解论文
溯源树
样例
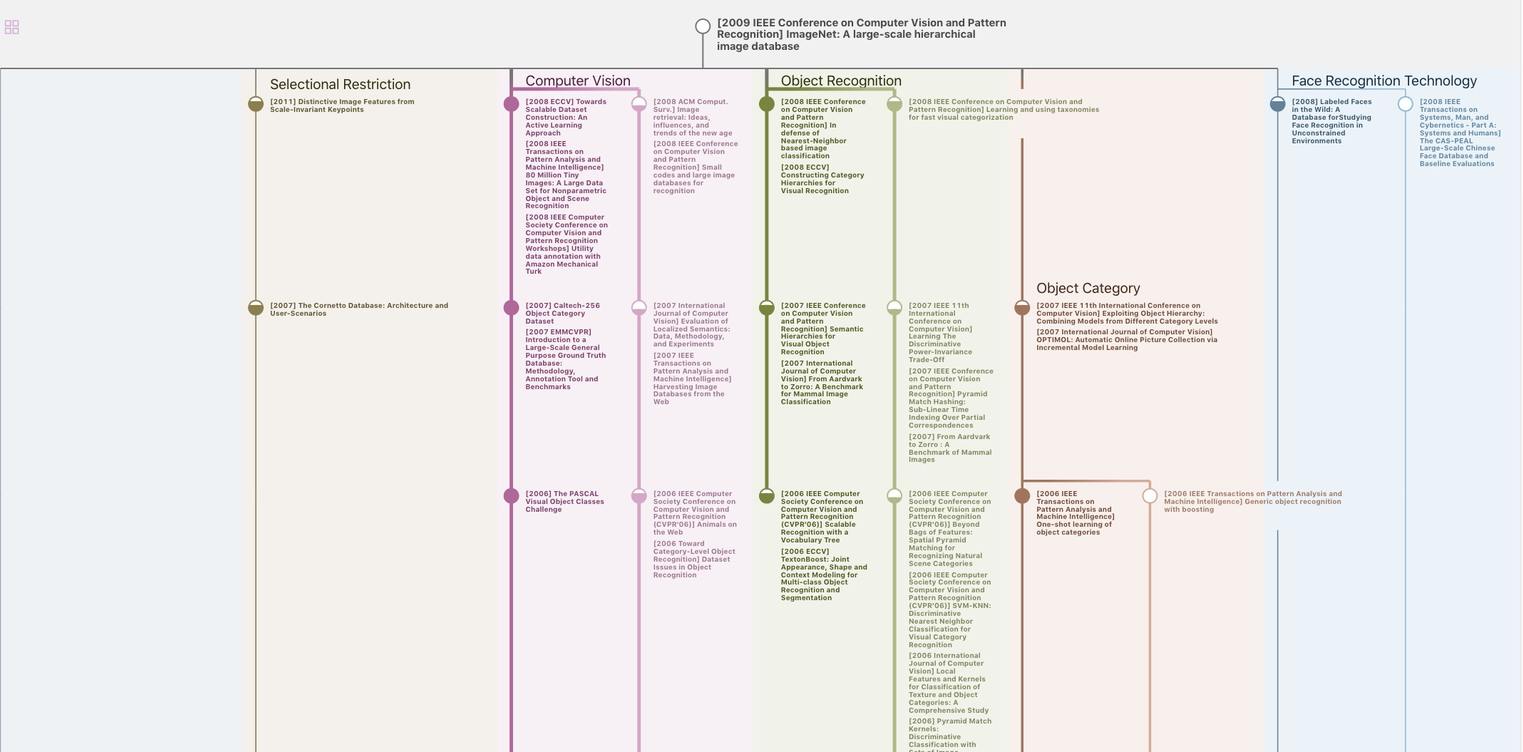
生成溯源树,研究论文发展脉络
Chat Paper
正在生成论文摘要