Development and validation of machine learning models to predict the need for haemostatic therapy in acute upper gastrointestinal bleeding.
Therapeutic advances in gastrointestinal endoscopy(2024)
摘要
Background:Acute upper gastrointestinal bleeding (AUGIB) is a major cause of morbidity and mortality. This presentation however is not universally high risk as only 20-30% of bleeds require urgent haemostatic therapy. Nevertheless, the current standard of care is for all patients admitted to an inpatient bed to undergo endoscopy within 24 h for risk stratification which is invasive, costly and difficult to achieve in routine clinical practice.
Objectives:To develop novel non-endoscopic machine learning models for AUGIB to predict the need for haemostatic therapy by endoscopic, radiological or surgical intervention.
Design:A retrospective cohort study.
Method:We analysed data from patients admitted with AUGIB to hospitals from 2015 to 2020 (n = 970). Machine learning models were internally validated to predict the need for haemostatic therapy. The performance of the models was compared to the Glasgow-Blatchford score (GBS) using the area under receiver operating characteristic (AUROC) curves.
Results:The random forest classifier [AUROC 0.84 (0.80-0.87)] had the best performance and was superior to the GBS [AUROC 0.75 (0.72-0.78), p < 0.001] in predicting the need for haemostatic therapy in patients with AUGIB. A GBS cut-off of ⩾12 was associated with an accuracy, sensitivity, specificity, positive predictive value (PPV) and negative predictive value (NPV) of 0.74, 0.49, 0.81, 0.41 and 0.85, respectively. The Random Forrest model performed better with an accuracy, sensitivity, specificity, PPV and NPV of 0.82, 0.54, 0.90, 0.60 and 0.88, respectively.
Conclusion:We developed and validated a machine learning algorithm with high accuracy and specificity in predicting the need for haemostatic therapy in AUGIB. This could be used to risk stratify high-risk patients to urgent endoscopy.
更多查看译文
AI 理解论文
溯源树
样例
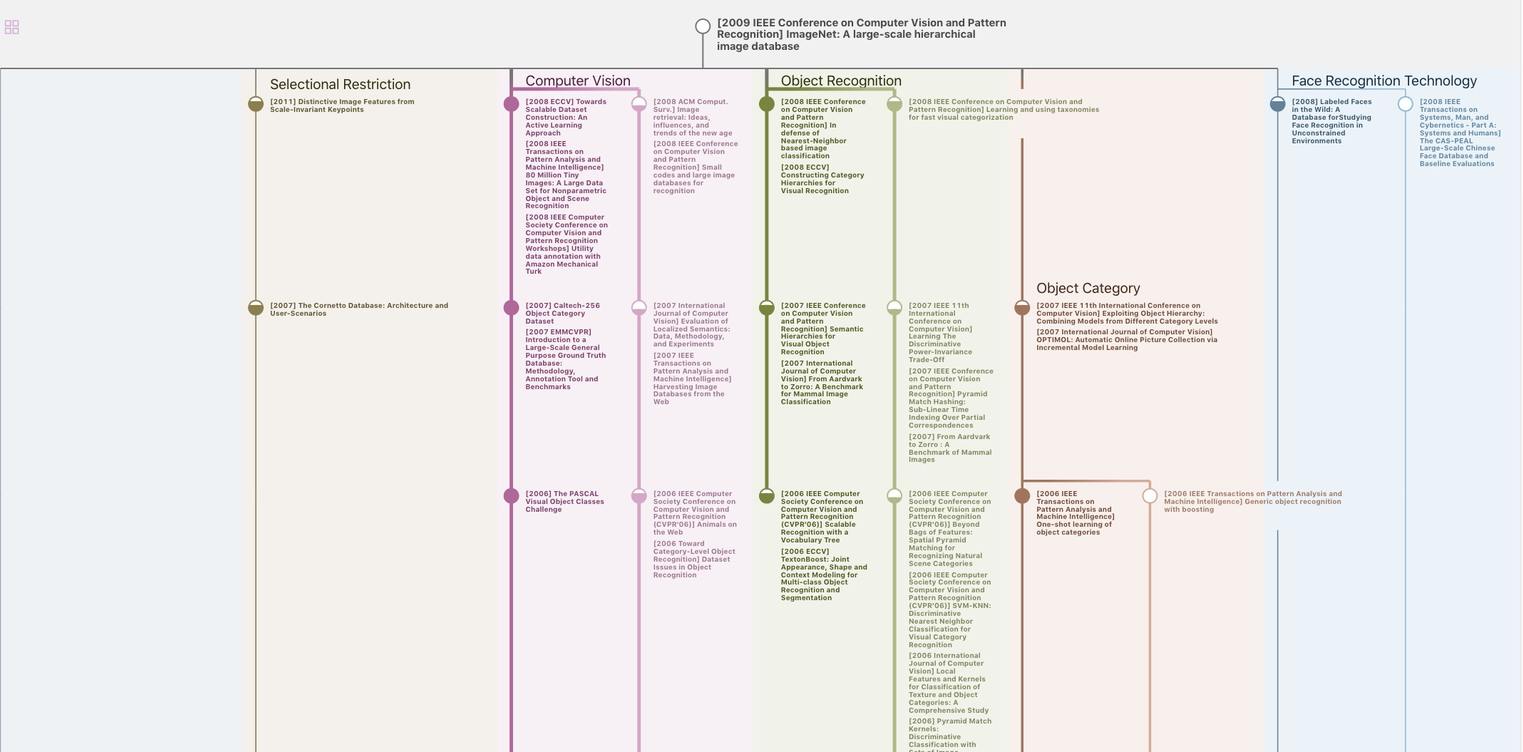
生成溯源树,研究论文发展脉络
Chat Paper
正在生成论文摘要