Urban flood susceptibility mapping using remote sensing, social sensing and an ensemble machine learning model
Sustainable Cities and Society(2024)
摘要
Flood susceptibility mapping is crucial for urban disaster management. However, the heterogeneity of urban land use and the complexity of terrain pose challenges to the accuracy and generalizability of flood models. In this paper, we propose a framework named the EMF model for urban flood mapping. Specifically, the social sensing and remote sensing were used for flooding information collection. The XGBoost, Support Vector Classifier (SVC), Multilayer Perceptron (MLP), and Multimodal Deep Learning (MDL), were used as the predictive models, and their results were ensembled using a Random Forest model to produce the final outcome. Results show that the EMF model outperforms the standalone models, with an accuracy of 0.942 on the training set and 0.940 on the testing set. The accuracy of the five models is ranked as EMF > MDL > XGBoost > SVC > MLP. The flood map indicates that flooding has a greater impact on the outskirts and suburbs of the city compared to its central urban areas. Farmland is the most affected land type, making up 54.8% of the flooded area. Overall, the proposed framework enables rapid and accurate identification of flood-prone areas, providing technical support for managers in formulating effective flood prevention strategies.
更多查看译文
关键词
Urban flood,Ensemble model,Machine learning,Remote sensing,Social sensing
AI 理解论文
溯源树
样例
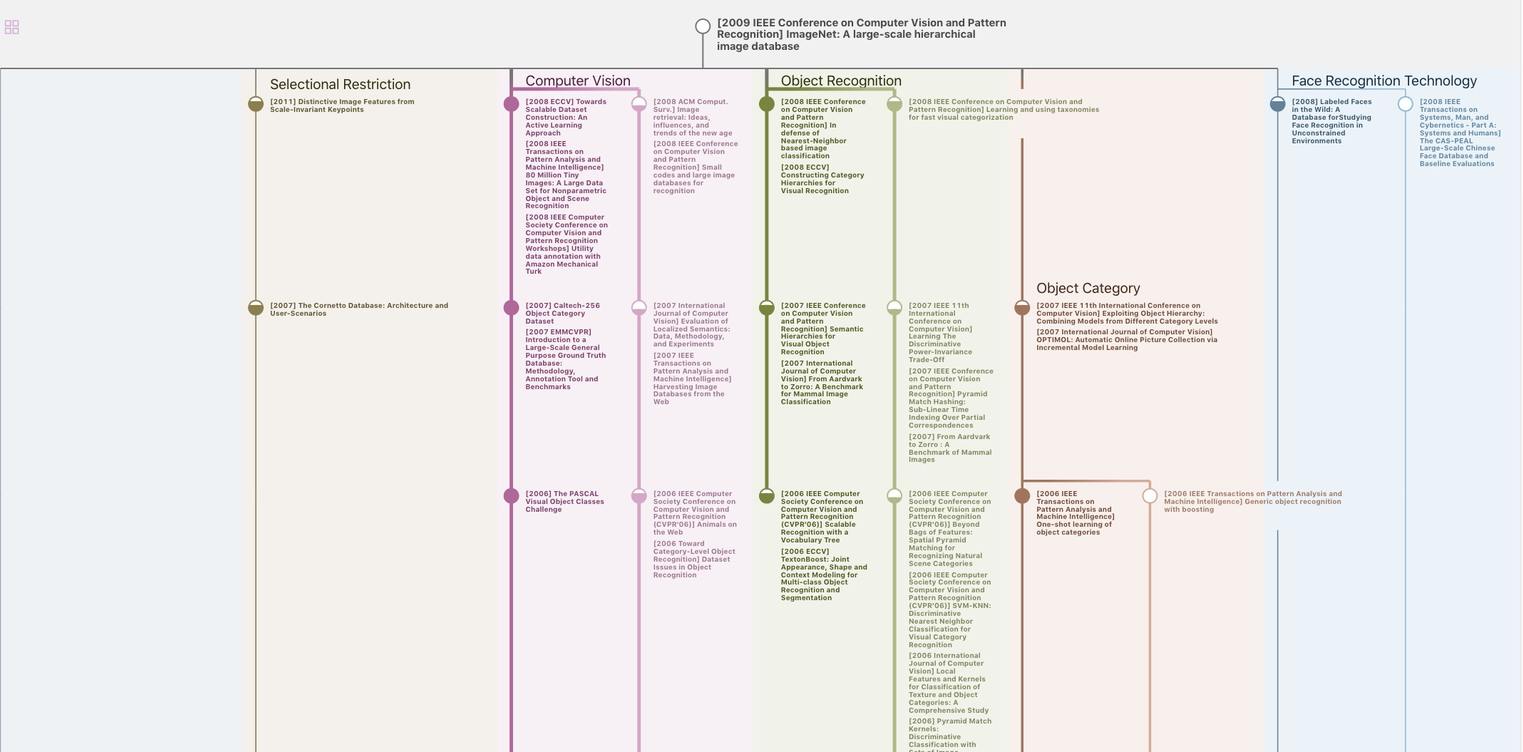
生成溯源树,研究论文发展脉络
Chat Paper
正在生成论文摘要