Residual feature learning with hierarchical calibration for gaze estimation
Machine Vision and Applications(2024)
摘要
Gaze estimation aims to predict accurate gaze direction from natural eye images, which is an extreme challenging task due to both random variations in head pose and person-specific biases. Existing works often independently learn features from binocular images and directly concatenate them for gaze estimation. In this paper, we propose a simple yet effective two-stage framework for gaze estimation, in which both residual feature learning (RFL) and hierarchical gaze calibration (HGC) networks are designed to consistently improve the performance of gaze estimation. Specifically, the RFL network extracts informative features by jointly exploring the symmetric and asymmetric factors between left and right eyes, which can produce accurate initial predictions as much as possible. Besides, the HGC network cascades a personal-specific transform module to further transform the distribution of gaze point from coarse to fine, which can effectively compensate the subjective bias in initial predictions. Extensive experiments on both EVE and MPIIGaze datasets show that our method outperforms the state-of-the-art approaches.
更多查看译文
关键词
Gaze estimation,Residual feature learning,Hierarchical gaze calibration
AI 理解论文
溯源树
样例
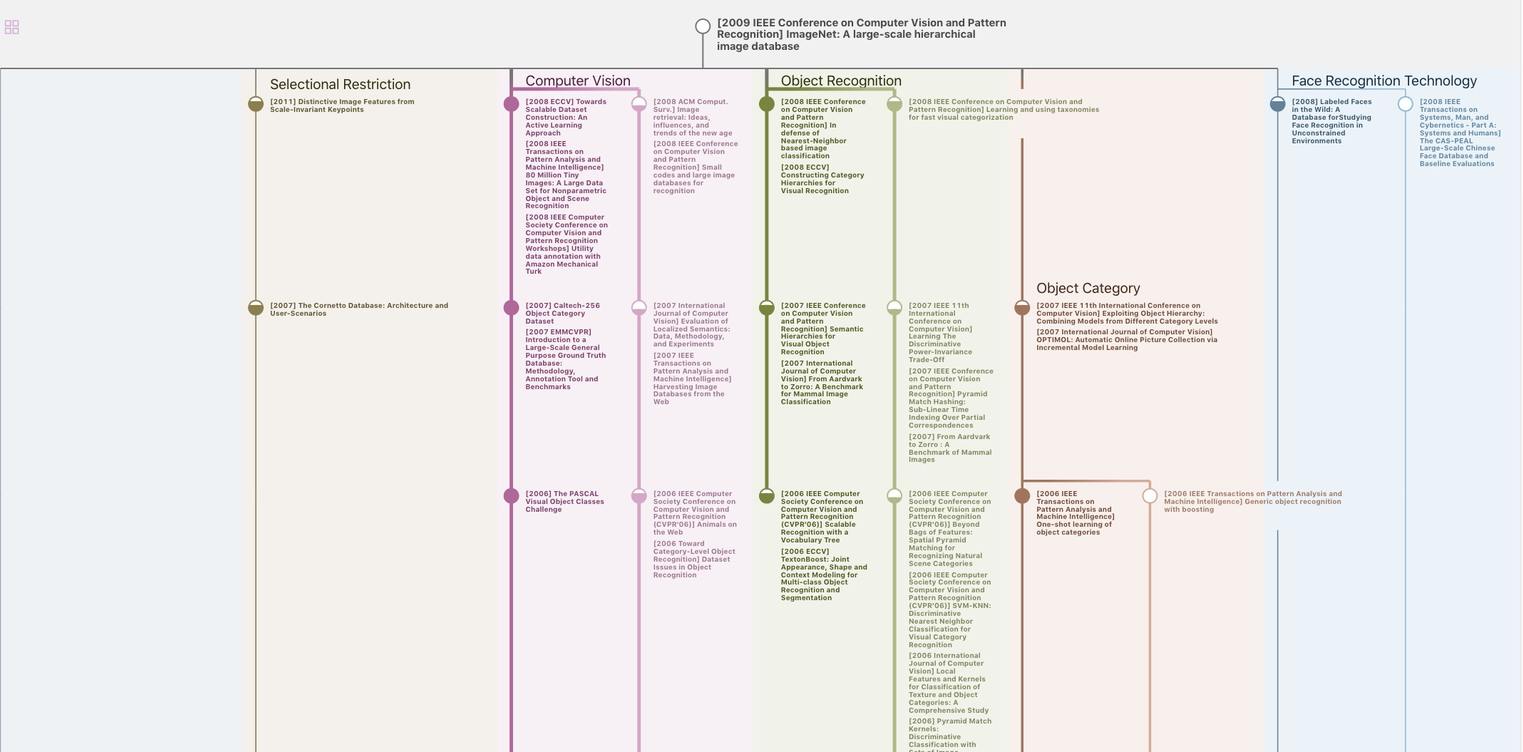
生成溯源树,研究论文发展脉络
Chat Paper
正在生成论文摘要