Explainable Deep Learning for sEMG-Based Similar Gesture Recognition: A Shapley-Value-Based Solution
Information Sciences(2024)
摘要
Surface electromyography (sEMG) based gesture recognition shows promise in enhancing human-robot interaction. However, accurately recognizing similar gestures is a challenging task, and the underlying mechanisms of gesture recognition are not well understood. To address these issues, we developed a new solution called the Shapley-value-based similar gesture recognition (SV-SGR) method. Our solution combines deep learning and game theory to achieve high recognition accuracy and interpretability. First, we devised a data preprocessing method that converts sEMG signals into sEMG color images, which can be more effectively utilized by deep learning techniques. Next, we established a deep-neural-network-based model for gesture recognition using the processed sEMG color images. Then, we designed a global explanation approach based on Shapley values to quantify the contribution of each channel to recognizing similar gestures. Finally, we carried out an explanation analysis, which provides feedback on the recognition model to enhance the precision of gesture recognition. Extensive comparisons and interpretable analyses have been conducted on real-world datasets, and the results demonstrate that the SV-SGR method outperforms other baselines under various experimental conditions. The interpretable analysis method based on Shapley values effectively enhances the performance of recognizing similar gestures and provides valuable insights into the decision-making process of recognition models.
更多查看译文
关键词
Explainable Artificial Intelligence,Deep Learning,Similar Gesture Recognition,Shapley Value
AI 理解论文
溯源树
样例
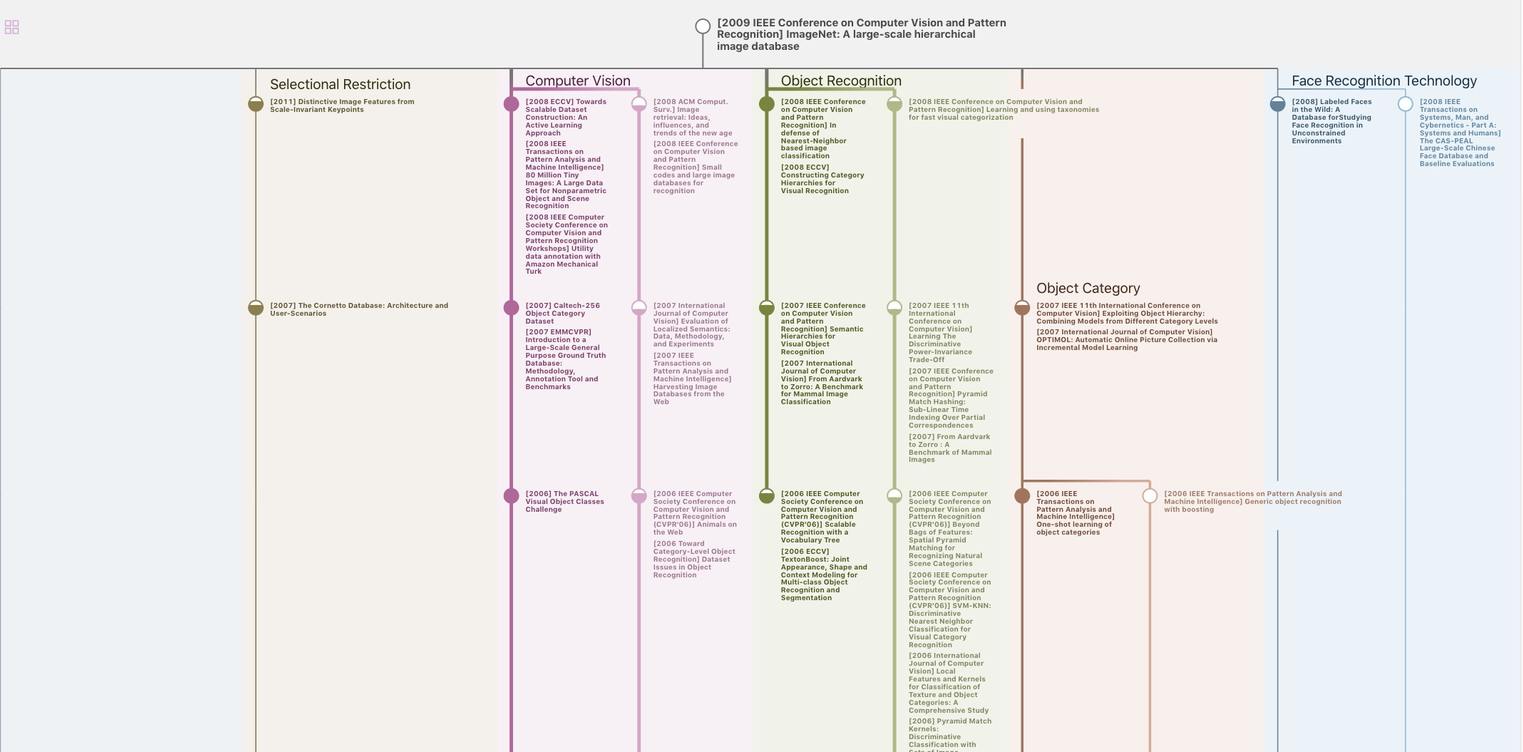
生成溯源树,研究论文发展脉络
Chat Paper
正在生成论文摘要