An operational framework to track individual farmland trees over time at national scales using PlanetScope imagery
crossref(2024)
摘要
Abstract Trees outside forests, in particular on croplands, play a crucial role for food security and climate resilience in the Global South, but are threatened by increasing climatic change and human pressures. The dynamics of agroforestry systems and national cropland tree stocks are largely unknown, as currently no robust monitoring system exists to remotely detect single field trees and track changes at national scales. Here we present a framework to track cropland trees at the single tree level across multiple years, using a combination of satellite imagery, deep learning, and object-based change classification. The approach matches annual tree centre predictions to detect changes, such as individual tree losses from logging or tree mortality events. The slope between annual tree prediction confidence heatmaps is also used to detect areas of gains, with possible applications for monitoring plantation and restoration areas. The framework is designed for PlanetScope nano-satellite imagery, which offers unprecedented opportunities for detailed tree monitoring given the combined high spatial and temporal resolution. PlanetScope imagery, however, also come with a range of challenges, which are discussed and for which solutions are proposed. We demonstrate the framework by applying it to a national-scale case study of cropland trees in Tanzania from 2018 to 2022.
更多查看译文
AI 理解论文
溯源树
样例
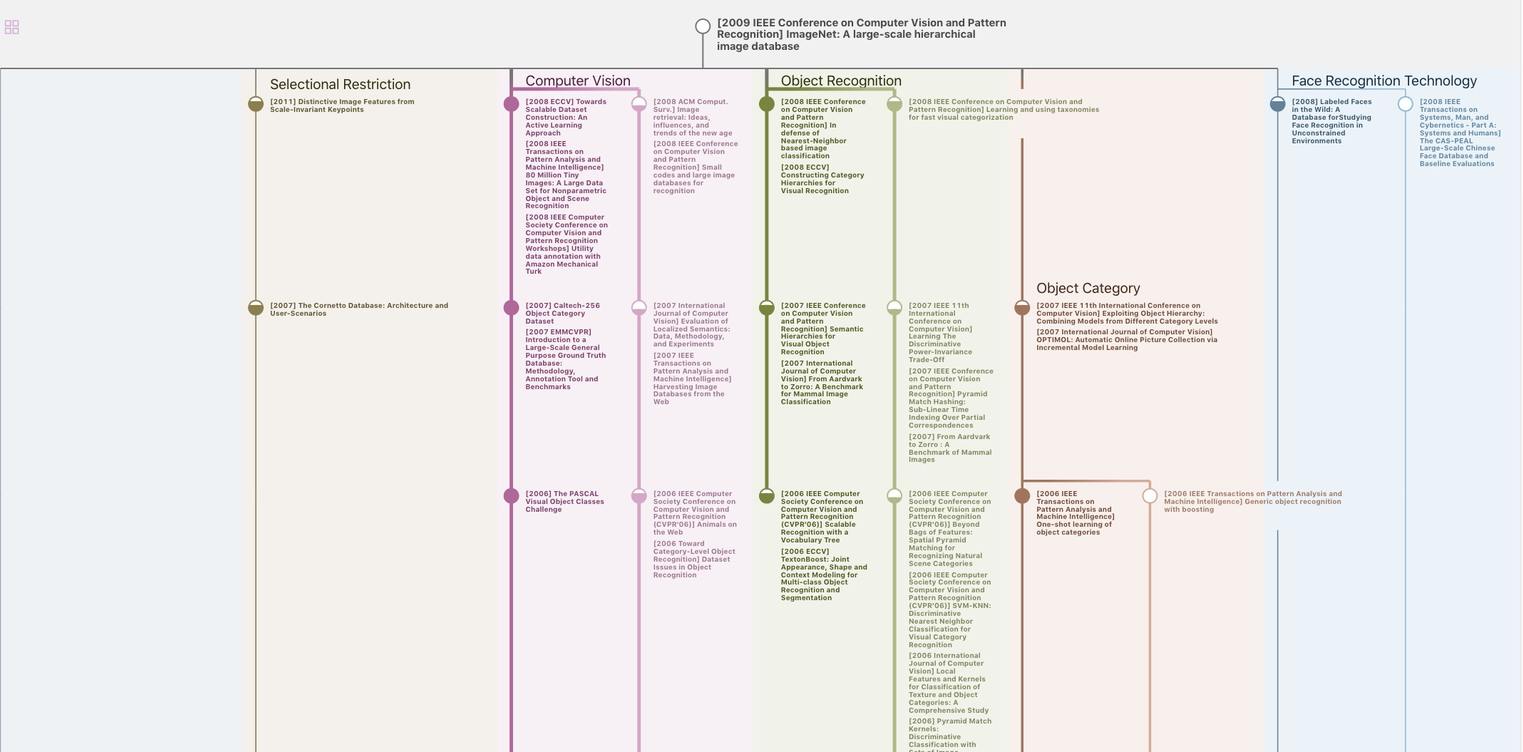
生成溯源树,研究论文发展脉络
Chat Paper
正在生成论文摘要