Exploring unseen 3D scenarios of physics variables using machine learning-based synthetic data: An application to wave energy converters
Environmental Modelling & Software(2024)
摘要
This work uses machine learning to produce synthetic data of wave energy converters from time-expensive 3D simulations based on computational fluid dynamics models. The simulations to analyse the response of these systems to incoming waves are lengthy and computationally expensive to obtain. Here, we explore the use of a beta-VAE and a Principal Components-based adversarial autoencoder for generating new synthetic data. The compression plus the generation of synthetic data introduces an exceptionally fast surrogate model of the original simulation and delivers more samples of either dynamic viscosity and velocity fields, enlarging the design space. The new generated synthetic samples can have a speed up from 5 to 6 orders of magnitude. The new design space can be used to improve the prediction of dynamic viscosity given the velocity fields. The generative model has the potential to capture the transition and the new physical phenomena under extreme initial conditions.
更多查看译文
关键词
Generative models,Synthetic data,Wave energy converters,Surrogate model
AI 理解论文
溯源树
样例
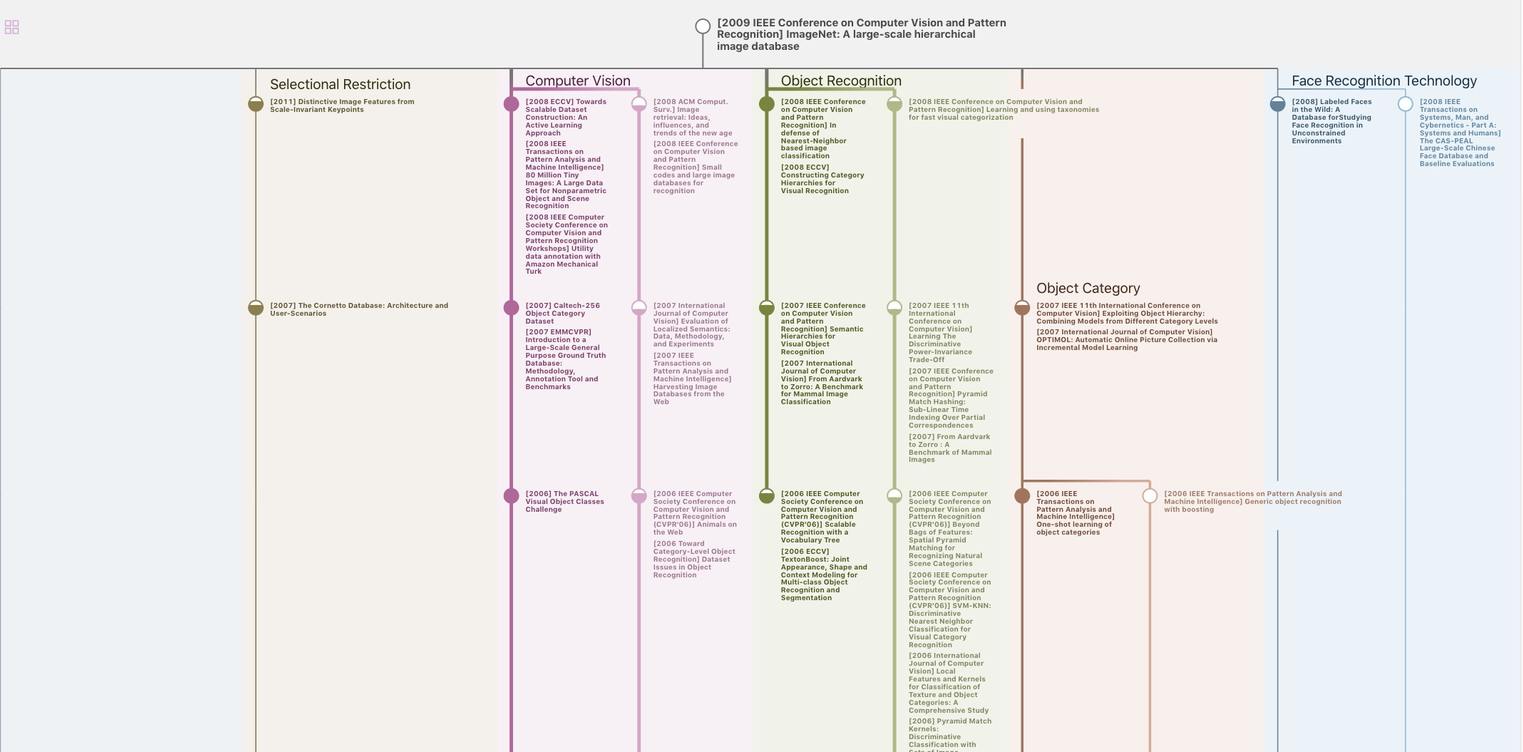
生成溯源树,研究论文发展脉络
Chat Paper
正在生成论文摘要