Abstract PO5-07-03: Integrating mechanism-based and data-driven modeling to predict the response of triple negative breast cancer to therapy
Cancer Research(2024)
摘要
Abstract Introduction Neoadjuvant chemotherapy (NAC) is the standard-of-care (SOC) for patients with locally advanced triple-negative breast cancer (TNBC) [1]. Unfortunately, only about half of TNBC patients achieve a pathological complete response (pCR) at the completion of NAC [2]. With the recent FDA approval of immunotherapy, the response rate improved by about 8% [3]. To try to further improve NAC response rates, we build upon methods that predict treatment response to optimize interventions and outcomes. Our previous studies have demonstrated that excellent predictive accuracy of TNBC response to NAC was achieved through calibrating mechanism-based models to both pre- and on- treatment imaging data [4]. However, by requiring on-treatment imaging data, therapy optimization can only occur after a patient begins NAC. Here, our goal is to relax the requirement of on-treatment imaging data such that we can predict, and potentially optimize, a patient’s response to NAC before initiating it. We will accomplish this through combining data-driven approaches with mechanism-based modeling to obtain spatiotemporal predictions of tumor response prior to NAC. More specifically, we integrate a reaction-diffusion equation based mathematical model with a U-Net based convolutional neural network (CNN). Methods The reaction-diffusion equation models the development of tumor cellularity over time as the sum of tumor cell diffusion, controlled by a fixed diffusivity, and tumor cell proliferation, controlled by a calibrated net proliferation rate. The net proliferation rate implicitly accounts for both proliferative and drug induced death effects. We calibrated the net proliferation rates in this model on a patient-specific basis for 128 patients from the ARTEMIS trial (NCT02276443) using three imaging timepoints – pre- (V1), post two cycles (V2), and post four cycles (V3) of A/C. We then employed a CNN to characterize the relationship between the calibrated net proliferation rates and the pre-treatment imaging data. The CNN takes pretreatment imaging inputs of the apparent diffusion coefficient map, percent enhancement maps from the dynamic contrast enhanced MRI timecourse, and precontrast T1 map. The CNN outputs an estimate of the calibrated net proliferation rate which can be used to run the mathematical model forward in time to predict cellularity maps at V2/3. In training the CNN, our loss considers both the concordance correlation coefficient (CCC) and the normalized root mean square error (NRMSE). We calculated loss on the net proliferation rate predictions as NRMSE with a CCC penalty to aid in characterizing the non-Gaussian distribution of the net proliferation rate. We calculated loss on the cellularity predictions as the CCC between predicted and measured voxel-wise cellularity. The CNN was trained using the Adam optimizer and a fivefold cross validation with a withheld test set of 26 patients. Results Using the CNN predictions to forward run the mechanism-based model in time to V3 we calculated change in total tumor cellularity and volume from V1 to V3. In the test cohort, we obtained CCC values between the predicted and measured changes of 0.95 and 0.91 for cellularity and volume, respectively. Discussion and summary Through integrating mechanistic modeling and deep learning, we can accurately predict the spatiotemporal development of TNBC response to NAC using only pretreatment imaging data. We will build upon these results by expanding our model to include separate proliferation and drug induced death terms to allow for therapy intervention and optimization. Additionally, we will incorporate other pretreatment data types including genetic data. [1] Liedtke et al., J of Clin Oncol, 2008. [2] Spring et al., Clin Cancer Res, 2020. [3] Schmid et al., NEJM, 2020. [4] Wu et al., Cancer Res, 2022. Citation Format: Casey Stowers, Chengyue Wu, Sidharth Kumar, Zhan Xu, Clinton Yam, Jong Bum Son, Jingfei Ma, Jonathan Tamir, Gaiane Rauch, Thomas Yankeelov. Integrating mechanism-based and data-driven modeling to predict the response of triple negative breast cancer to therapy [abstract]. In: Proceedings of the 2023 San Antonio Breast Cancer Symposium; 2023 Dec 5-9; San Antonio, TX. Philadelphia (PA): AACR; Cancer Res 2024;84(9 Suppl):Abstract nr PO5-07-03.
更多查看译文
AI 理解论文
溯源树
样例
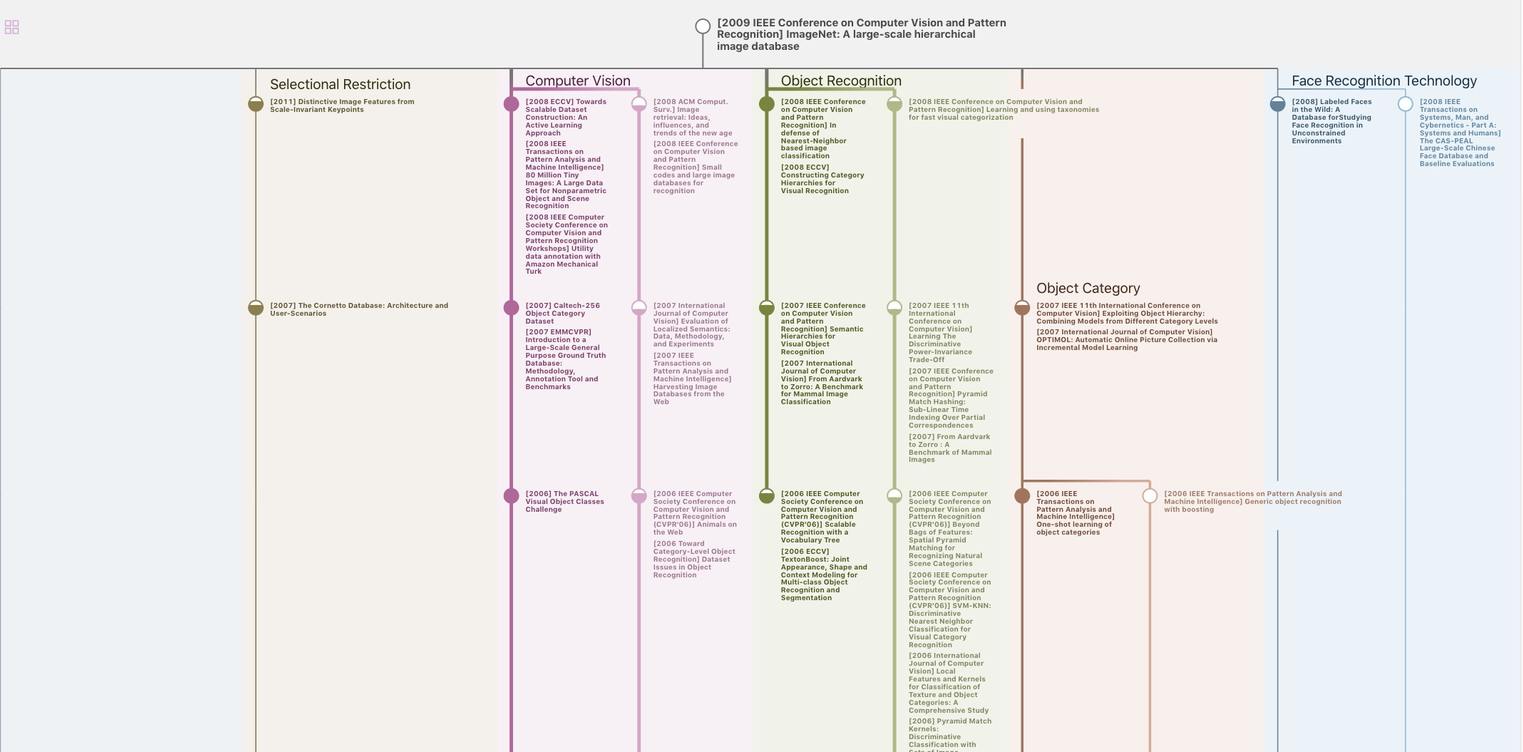
生成溯源树,研究论文发展脉络
Chat Paper
正在生成论文摘要