ContinuousSensing: a task allocation algorithm for human–robot collaborative mobile crowdsensing with task migration
CCF Transactions on Pervasive Computing and Interaction(2024)
摘要
Mobile crowdsensing (MCS) represents a novel and innovative sensing paradigm. With the advancement of the Internet of Things (IoT) and communication technologies, an increasing number of robots are also equipped with a plethora of sensors, which makes it possible for them to participate in MCS. However, robotic participants exhibit distinct characteristics from human participants. Therefore, tailoring the allocation of sensing tasks based on the characteristics of different types of participants is crucial to improving the efficiency of MCS. Furthermore, abandonment of tasks by participants due to various reasons is a common occurrence in real-world environments. Managing the migration of unfinished tasks and achieving continuity of sensing is thus a challenging issue. Therefore, we propose a novel MCS framework called ContinuousSensing. This framework establishes a human–robot collaborative crowdsensing model, which facilitates cooperation and coordination between different types of robots and humans. It also features a task migration model that transfers tasks from failed participants to other available participants. Experimental results show that the crowdsensing task allocation framework proposed by us has demonstrated the ability to significantly improve the quality of crowdsensing task completion while maintaining relatively low task execution costs.
更多查看译文
关键词
Mobile Crowdsensing,Human–robot collaboration,Ability prediction,Task allocation
AI 理解论文
溯源树
样例
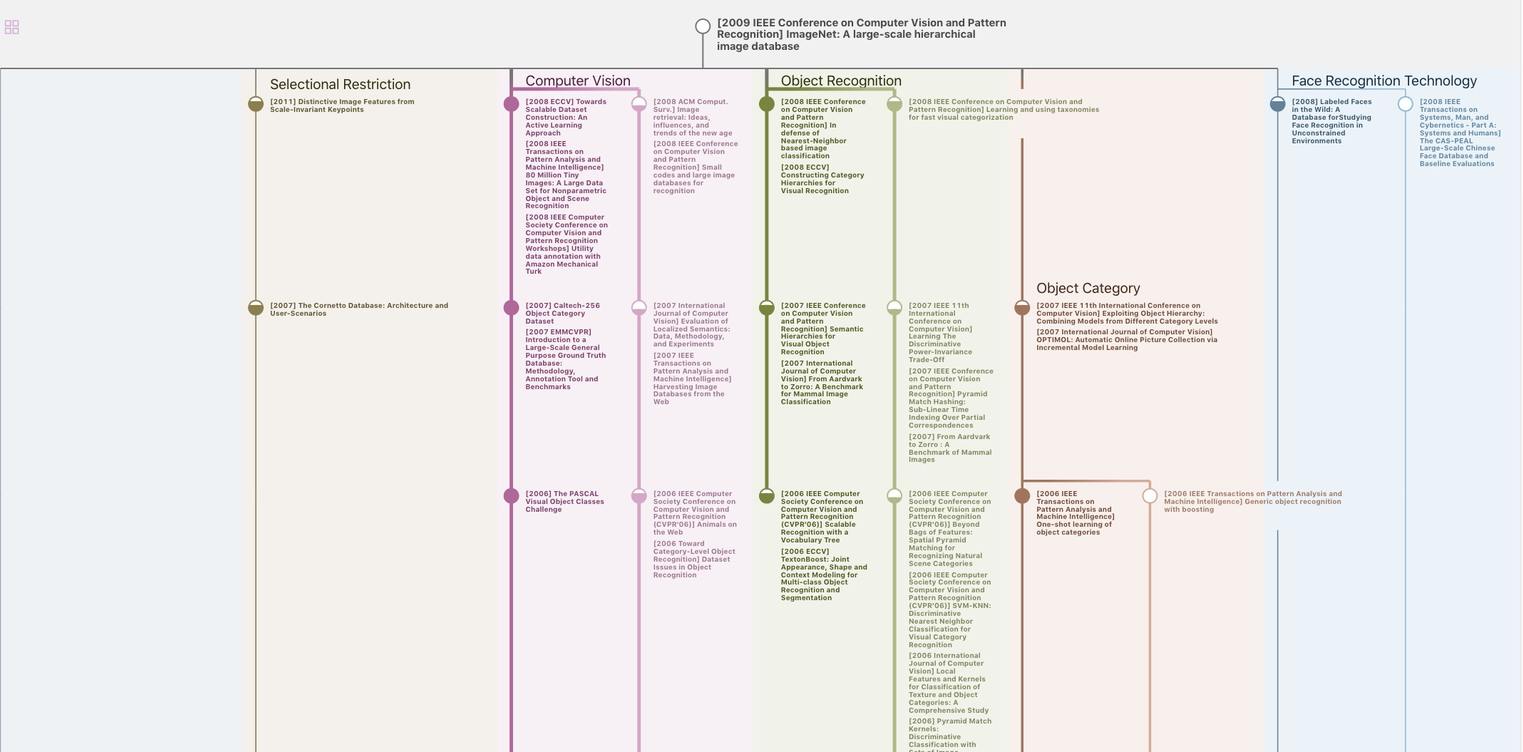
生成溯源树,研究论文发展脉络
Chat Paper
正在生成论文摘要