Fault Diagnosis of Harmonic Drives based on Markov Field Graph Information
IEEE Sensors Journal(2024)
摘要
Fault rate of harmonic drives (HDs) is high due to their complex structures, especially under heavy working conditions, and an efficient fault diagnosis method is highly desired. In this paper, a method based on Markov field graph information (MFGI) and graph cardinality preserved attention network with residual connections (GCPAR) was proposed to classify fault states of the HDs. Firstly, vibration signals of the HDs were transformed into graphs through the MFGI and then the GCPAR was applied for extraction and classification. Finally, the proposed method was validated using experimental data from real industrial HD health-monitoring platforms, wherein fault diagnosis accuracy was up to 98.72% and 92.12% for single and multi-speed datasets, respectively, significantly higher than those using other comparative methods. To the best of our knowledge, it was the first time that the MFGI and the GCPAR methods were jointly used for HDs fault diagnosis, wherein superior visibility, interpretability and accuracy were obtained, showing its potential to be applied in real industrial scenarios.
更多查看译文
关键词
Harmonic drives,Fault diagnosis,Markov field graph information,Graph neural network (GNN)
AI 理解论文
溯源树
样例
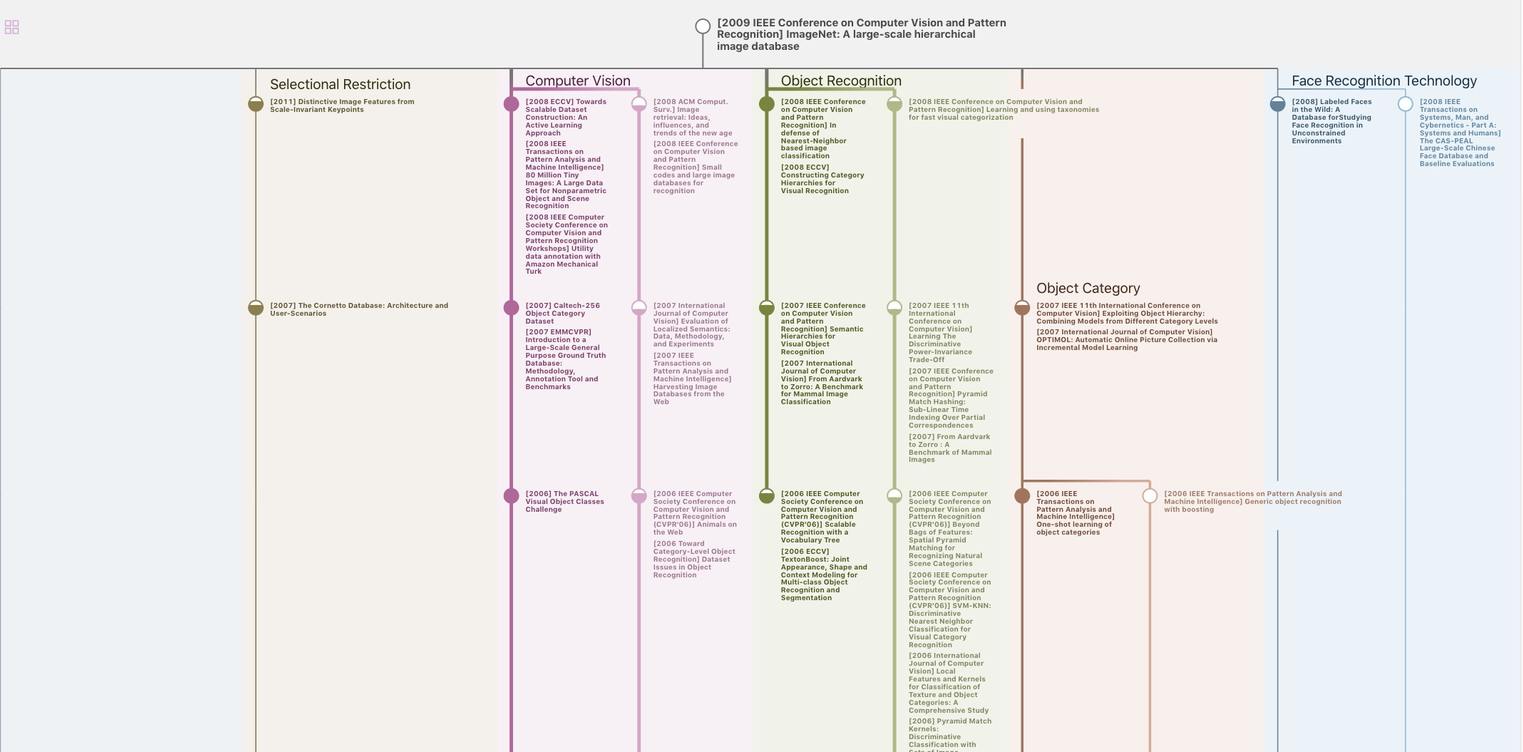
生成溯源树,研究论文发展脉络
Chat Paper
正在生成论文摘要