Sampled-Data-Based Iterative Cost-Learning Model Predictive Control for T–S Fuzzy Systems
IEEE Transactions on Systems, Man, and Cybernetics: Systems(2024)
摘要
In this article, an iterative cost-learning model predictive control (ICLMPC) is proposed for nonlinear networked control systems (NCSs) in the presence of aperiodic sampling. The proposed ICLMPC is useful not only to guarantee asymptotic stability of the closed-loop system with aperiodic sampling but also to improve control performance in the case of performing an iterative task. In the proposed method, the nonlinear system of NCSs is mathematically represented as an aperiodic sampled-data Takagi–Sugeno (T–S) fuzzy system. Based on this representation, the ICLMPC design is formulated in terms of a finite-horizon optimal control problem in which a new terminal cost function is considered. The terminal cost function is constructed by a Lyapunov function with a looped-functional and an iteratively minimized function (IMF). From the Lyapunov function with the looped-functional, it is possible to guarantee that the ICLMPC asymptotically stabilizes the aperiodic sampled-data T–S fuzzy system. To obtain an iteratively improved control performance, the IMF takes the minimized value among the integrals of the collected data at each iteration. The validity and effectiveness of the proposed method are illustrated by two practical examples in the simulation section.
更多查看译文
关键词
Iterative cost learning,model predictive control (MPC),sampled-data control systems,Takagi–Sugeno (T–S) fuzzy systems
AI 理解论文
溯源树
样例
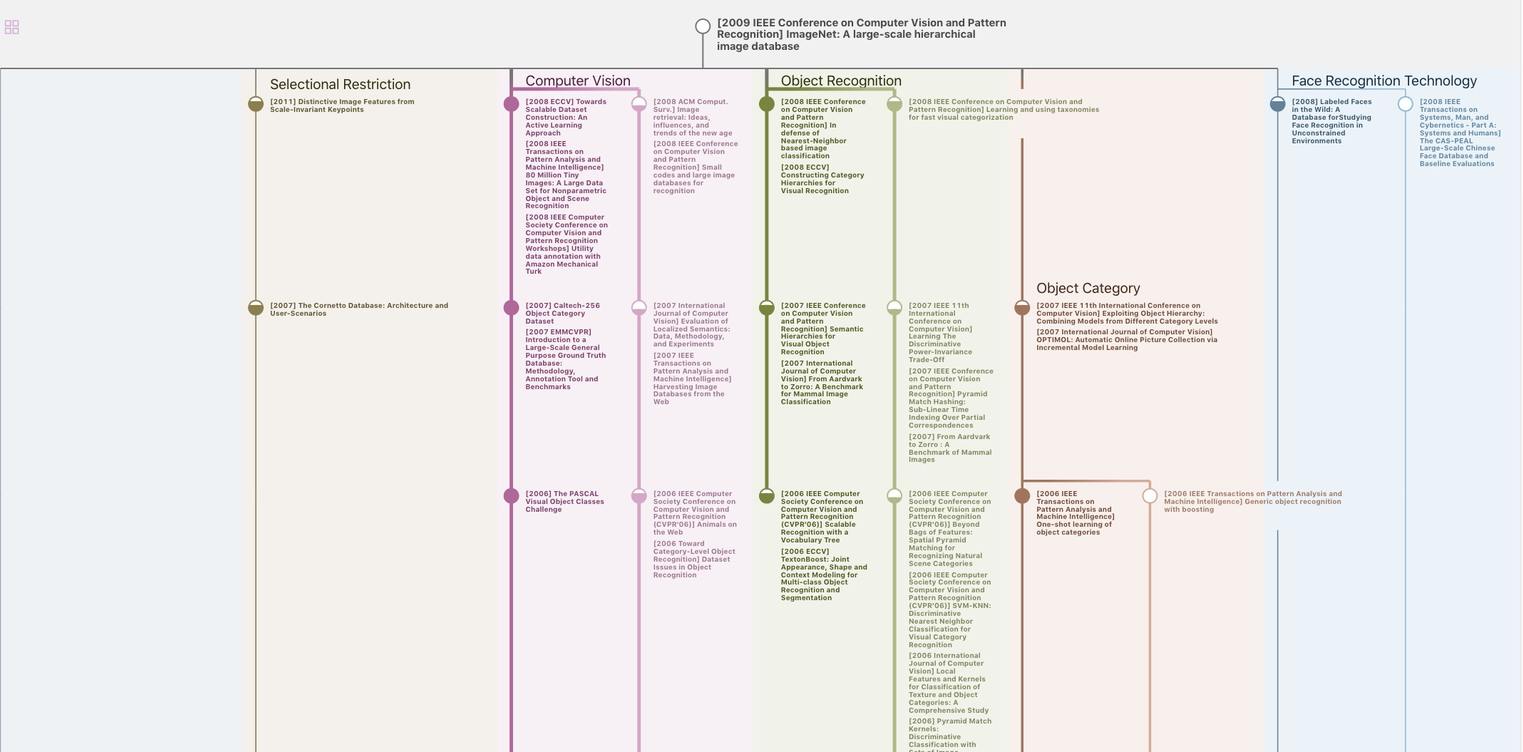
生成溯源树,研究论文发展脉络
Chat Paper
正在生成论文摘要