MF-OML: Online Mean-Field Reinforcement Learning with Occupation Measures for Large Population Games
arxiv(2024)
摘要
Reinforcement learning for multi-agent games has attracted lots of attention
recently. However, given the challenge of solving Nash equilibria for large
population games, existing works with guaranteed polynomial complexities either
focus on variants of zero-sum and potential games, or aim at solving (coarse)
correlated equilibria, or require access to simulators, or rely on certain
assumptions that are hard to verify. This work proposes MF-OML (Mean-Field
Occupation-Measure Learning), an online mean-field reinforcement learning
algorithm for computing approximate Nash equilibria of large population
sequential symmetric games. MF-OML is the first fully polynomial multi-agent
reinforcement learning algorithm for provably solving Nash equilibria (up to
mean-field approximation gaps that vanish as the number of players N goes to
infinity) beyond variants of zero-sum and potential games. When evaluated by
the cumulative deviation from Nash equilibria, the algorithm is shown to
achieve a high probability regret bound of Õ(M^3/4+N^-1/2M) for
games with the strong Lasry-Lions monotonicity condition, and a regret bound of
Õ(M^11/12+N^- 1/6M) for games with only the Lasry-Lions
monotonicity condition, where M is the total number of episodes and N is
the number of agents of the game. As a byproduct, we also obtain the first
tractable globally convergent computational algorithm for computing approximate
Nash equilibria of monotone mean-field games.
更多查看译文
AI 理解论文
溯源树
样例
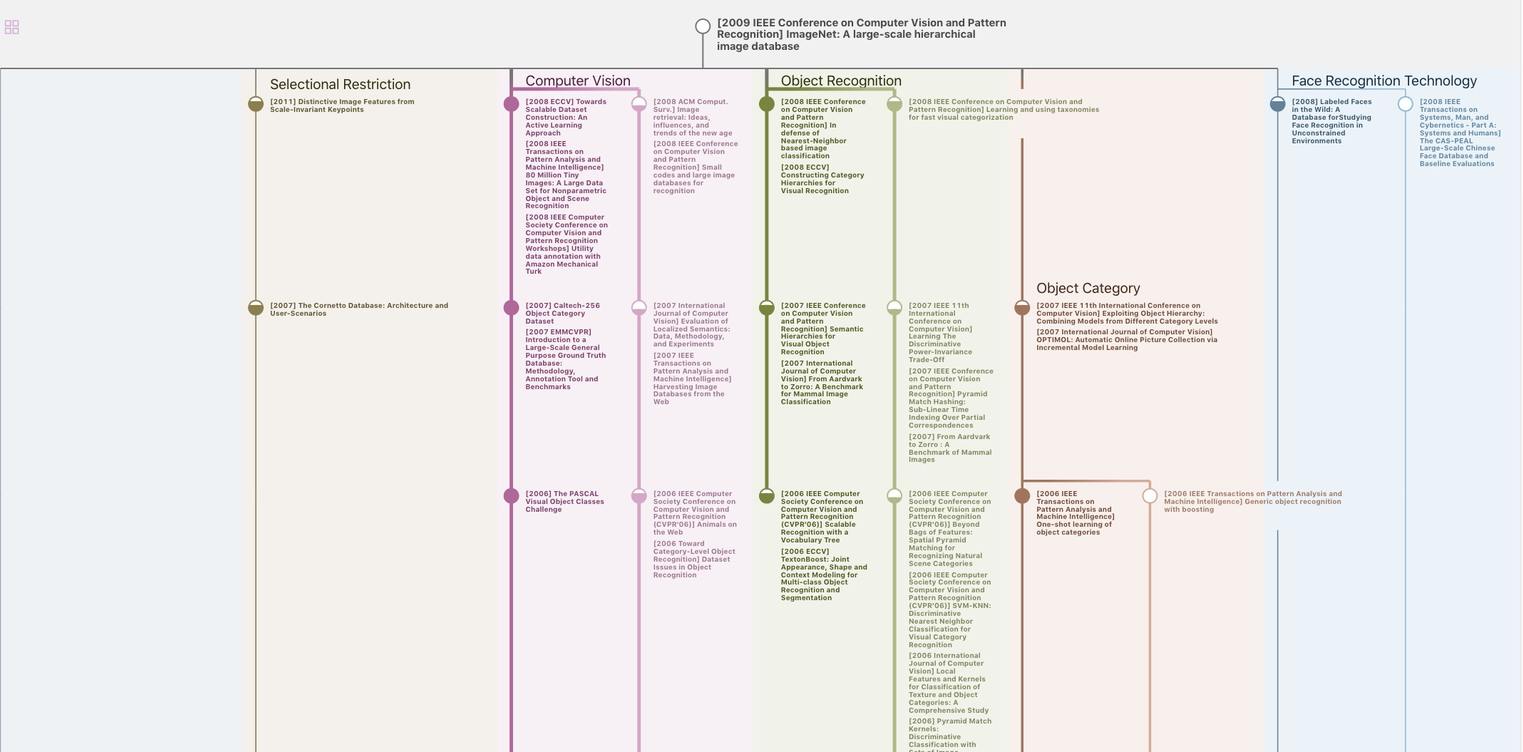
生成溯源树,研究论文发展脉络
Chat Paper
正在生成论文摘要