DSNet: A Superior Real-Time Semantic Segmentation Model for Road Scenes
2023 9th International Conference on Computer and Communications (ICCC)(2023)
摘要
A series of semantic segmentation models have achieved remarkable accuracy, but their high computational cost limits their practical applications in areas such as autonomous driving and robotics. Recently, some multi-branch real-time semantic segmentation models have been proposed to improve segmentation accuracy by introducing detail branches. However, there is a lack of auxiliary guidance in the design of detail branches, and there is a high computational cost problem in designing multi-branch information fusion modules. Therefore, to address these issues, in this paper, we propose a real-time semantic segmentation model called DSNet, which achieves a good balance between speed and accuracy. DSNet consists of two branches: the detail branch and the semantic branch. The detail branch effectively extracts detail information by introducing edge loss as auxiliary supervision, while the semantic branch extracts deeper-level semantic information by increasing network depth and channel count. Finally, the fusion of detail and semantic information serves as the feature for predicting the segmentation map. To fuse the detail and semantic information, we design a novel feature fusion module called LSAF, which efficiently integrates features from different branches with low computational costs. Our method achieves a speed of 152.3 FPS with an accuracy of 72.8% on the CityScapes test dataset.
更多查看译文
AI 理解论文
溯源树
样例
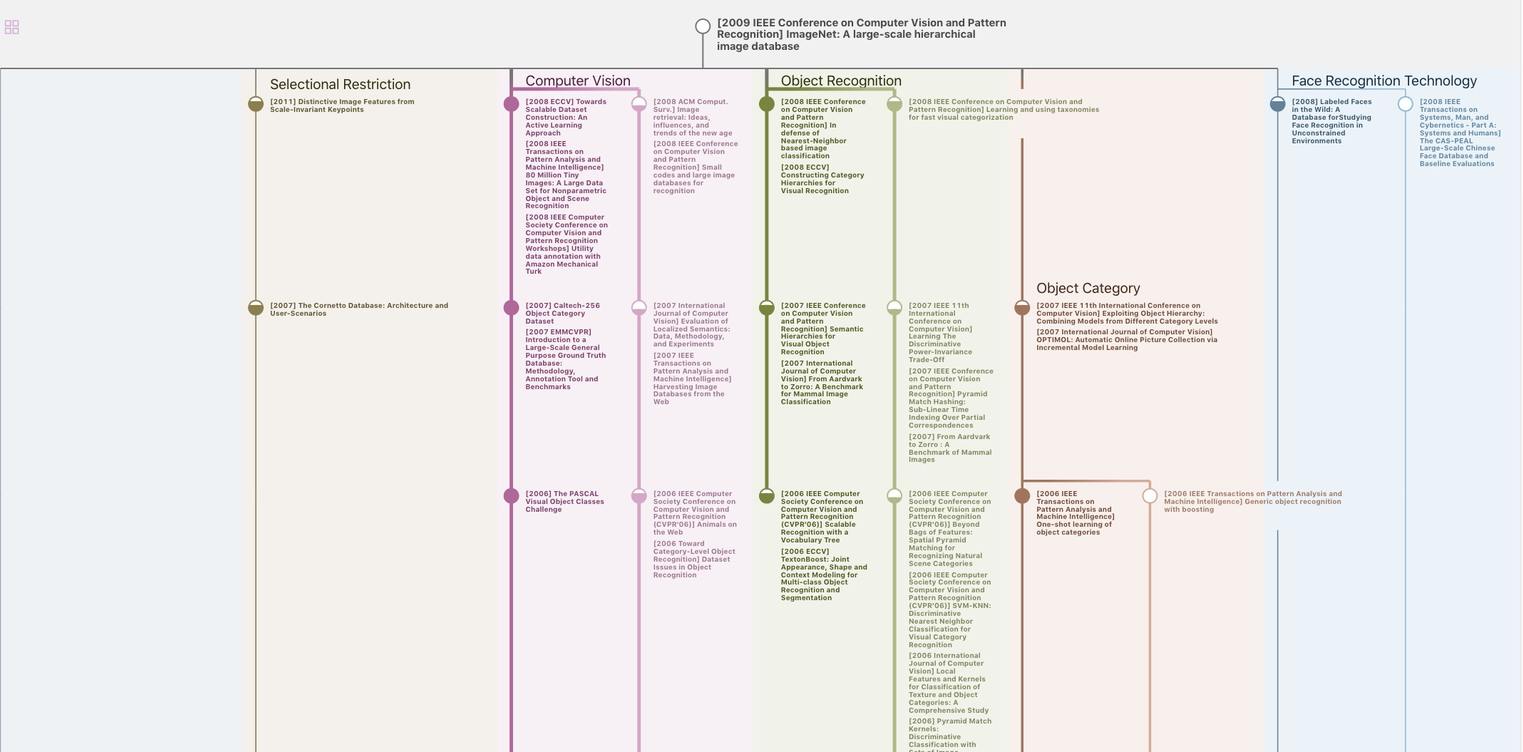
生成溯源树,研究论文发展脉络
Chat Paper
正在生成论文摘要