Class Balancing in a Cardiovascular Disease Domain.
Mexican International Conference on Computer Science(2023)
摘要
The imbalanced data problem is a significant issue in medical data sets, and dealing with it can be challenging for performance results. Resampling techniques do not only generate or remove synthetic instances. They also generate noisy instances, misclassify instances at boundaries, or resample instances from outliers. For these reasons, there are a variety of techniques for resolving imbalanced data. This paper focuses on the use of different resampling techniques: Synthetic Minority Over-sampling Technique, Adaptive Synthetic, Borderline-Synthetic Minority Oversampling Technique, Support Vector Machine-Synthetic Minority Oversampling Technique, Synthetic Minority Oversampling Technique+Edited Nearest Neighbor, and Synthetic Minority Oversampling Technique+Tomek Links, to deal with imbalanced data and the selection of the best resampling technique. We also show the performance differences between the classifiers XGBoost, k-Nearest Neighbor, and Support Vector Machine, the hyperparameter configuration, and the accuracy, precision, recall, F1 score, Area Under the Curve, F2 score, and Area Under the Precision-Recall Curve evaluation metrics. We conclude that the best classifier with hyperparameter configuration based on the accuracy metric was k-Nearest Neighbor with the hybrid technique Synthetic Minority Oversampling Technique+Edited Nearest Neighbor. All the evaluation metrics for this classifier were in the range between 97% and 100%.
更多查看译文
AI 理解论文
溯源树
样例
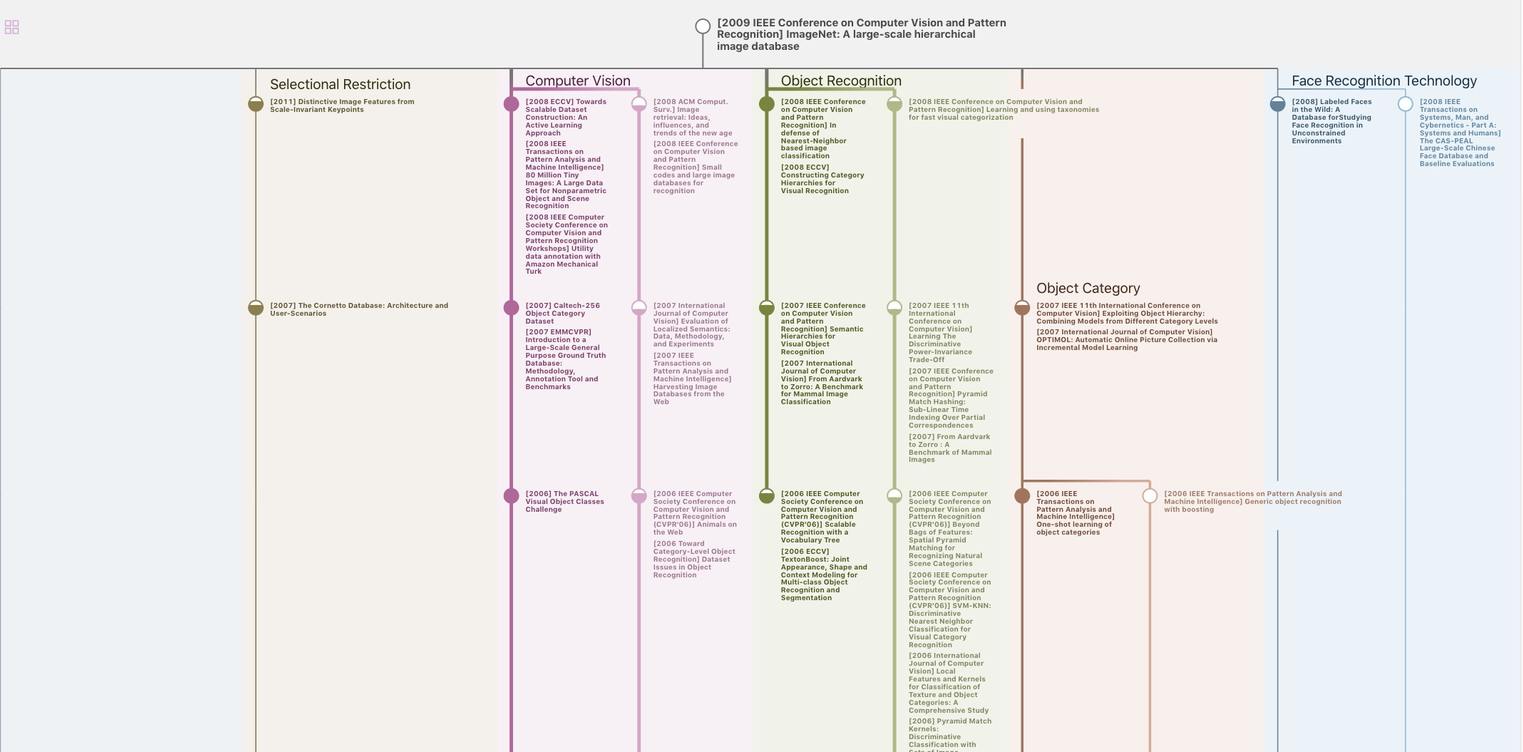
生成溯源树,研究论文发展脉络
Chat Paper
正在生成论文摘要