Enhanced network inference from sparse incomplete time series through automatically adapted L_1 regularization
Applied Network Science(2024)
摘要
Reconstructing dynamics of complex systems from sparse, incomplete time series data is a challenging problem with applications in various domains. Here, we develop an iterative heuristic method to infer the underlying network structure and parameters governed by Ising dynamics from incomplete spin configurations based on sparse and small-sized samples. Our method iterates between imputing missing spin states given current coupling strengths and re-estimating couplings from completed spin state data. Central to our approach is the novel application of adaptive l_1 regularization on updating coupling strengths, which features an automatic adjustment of the regularization strength throughout the iterative inference process. By doing so, we aim at preventing over-fitting and enforcing the sparsity of couplings without access to ground truth parameters. We demonstrate that this approach accurately recovers parameters and imputes missing spins even with substantial missing data and short time series, providing improvements in the inference of Ising model parameters even for relatively small sample sizes.
更多查看译文
关键词
Network inference,Inverse ising model,Complex networks
AI 理解论文
溯源树
样例
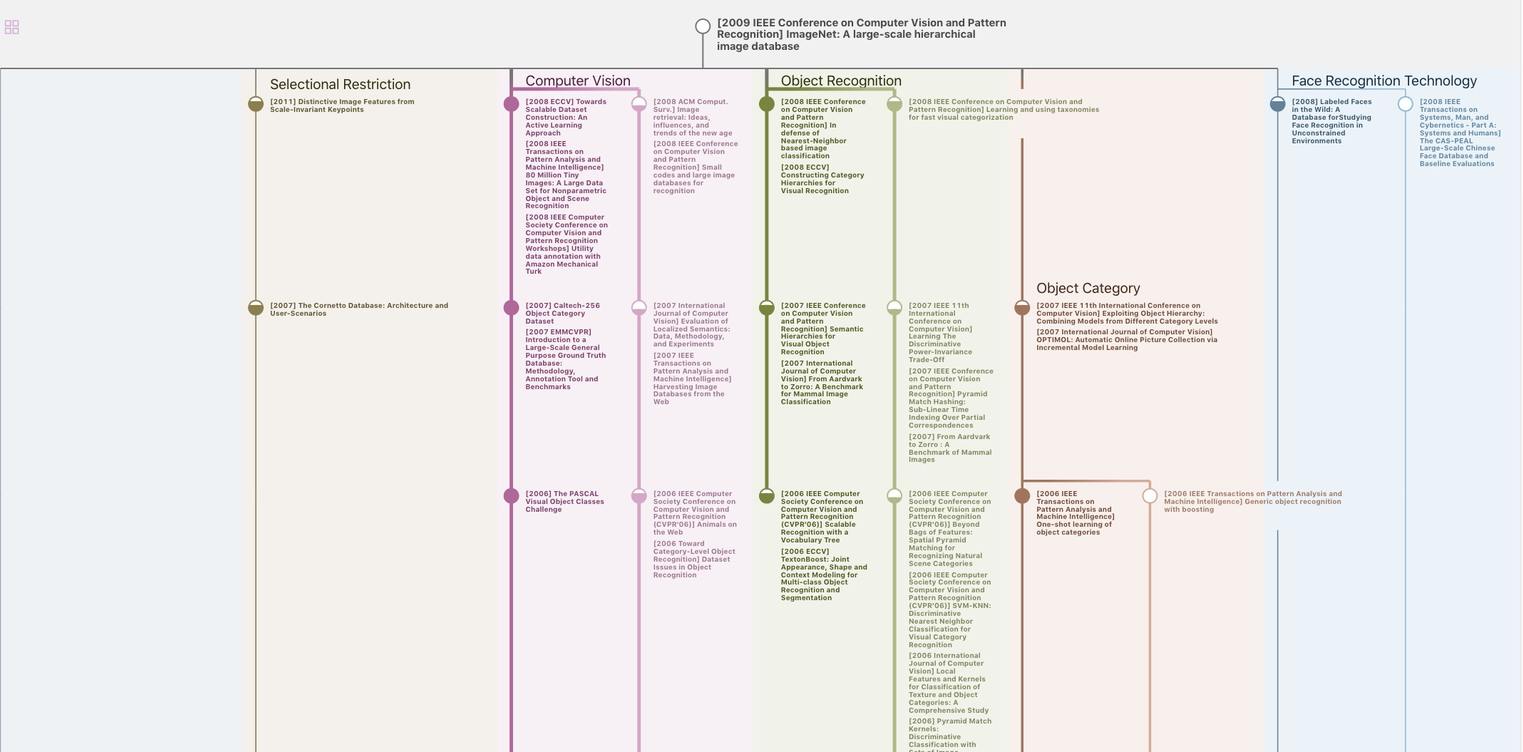
生成溯源树,研究论文发展脉络
Chat Paper
正在生成论文摘要