Ghost Reservoir: A Memory-Efficient Low-Power and Real-Time Neuromorphic Processor of Liquid State Machine With On-Chip Learning
IEEE Transactions on Circuits and Systems II: Express Briefs(2024)
摘要
Neuromorphic processors commonly execute spiking neural networks (SNN) models to obtain high energy efficiency. Compared to standard SNNs, liquid state machine (LSM), the spiking variant of reservoir computing, exhibits advantages in image classification, and are especially more promising in speech recognition. However, LSM-based neuromorphic processors suffer from weight storage overhead in resource-constrained edge applications. To address this, we propose ghost reservoir: a memory-efficient LSM based neuromorphic processor enabling on-chip spike-time-dependent plasticity-based backpropagation (BP-STDP) learning. For the LSM reservoir layer, we adopt a stateless-neuron model and an on-the-fly weight re-generation scheme to avoid the storages for both membrane potentials and weights. For the readout learning layer, a stochastic weight update approach is implemented to reduce the memory bit-width. These techniques contribute to an aggregate of a 482.5 KB on-chip memory reduction on the FPGA prototype. Implemented on the very-low-cost Xilinx Zynq-7010 device, our prototype achieved real-time processing, demonstrated comparably high on-chip learning accuracies of 94.93%, 84.65%, and 92% on the MNIST, N-MNIST, and FSDD datasets, respectively. These experimental results indicate that our LSM-based lightweight neuromorphic design is quite suitable for speech and visual recognition tasks in many resource-constrained edge intelligent applications.
更多查看译文
关键词
neuromorphic hardware,spiking neural network,reservoir computing,liquid state machine,on-chip learning
AI 理解论文
溯源树
样例
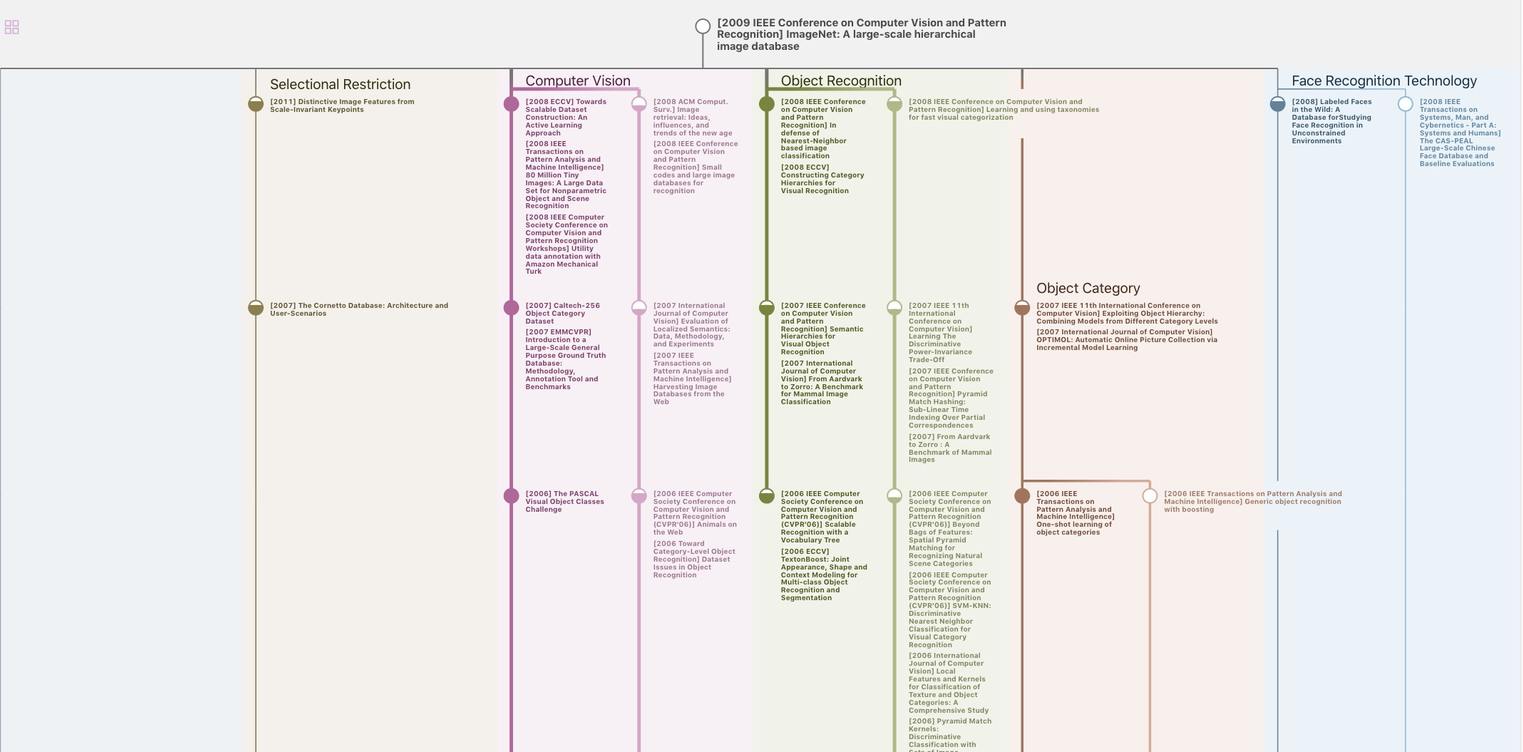
生成溯源树,研究论文发展脉络
Chat Paper
正在生成论文摘要