Understanding Multimodal Contrastive Learning Through Pointwise Mutual Information
arxiv(2024)
摘要
Multimodal representation learning to integrate different modalities, such as
text, vision, and audio is important for real-world applications. The symmetric
InfoNCE loss proposed in CLIP is a key concept in multimodal representation
learning. In this work, we provide a theoretical understanding of the symmetric
InfoNCE loss through the lens of the pointwise mutual information and show that
encoders that achieve the optimal similarity in the pretraining provide a good
representation for downstream classification tasks under mild assumptions.
Based on our theoretical results, we also propose a new similarity metric for
multimodal contrastive learning by utilizing a nonlinear kernel to enrich the
capability. To verify the effectiveness of the proposed method, we demonstrate
pretraining of multimodal representation models on the Conceptual Caption
datasets and evaluate zero-shot classification and linear classification on
common benchmark datasets.
更多查看译文
AI 理解论文
溯源树
样例
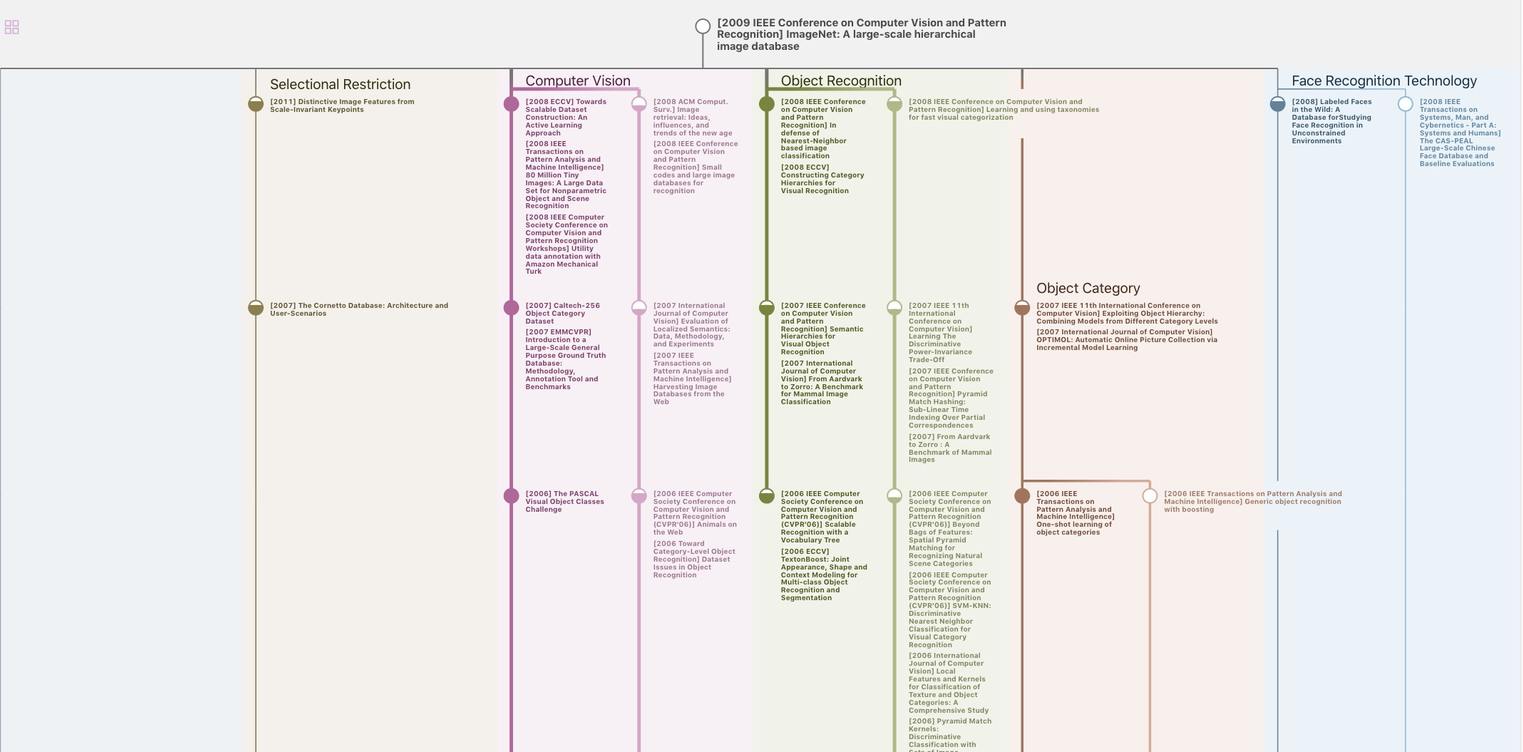
生成溯源树,研究论文发展脉络
Chat Paper
正在生成论文摘要