Re-decoupling the classification branch in object detectors for few-class scenes
Pattern Recognition(2024)
摘要
Few-class object detection is a critical task in numerous scenes, such as autonomous driving and intelligent surveillance. The current researches mainly focus on the correlation or decoupling between classification and regression subtasks in object detection. However, they rarely take advantage of the potential of re-decoupling the classification subtask. In this paper, we propose to re-decouple the classification branch in object detection for few-class scenes by reducing multi-classification features to multiple binary-classification features. Since multi-classification losses cannot supervise the network to learn decoupled binary-classification features, we introduce a single-class loss to supervise decoupled multiple binary-classification branches. In particular, we propose a basic feature degradation head (FD-Head) structure that decouples the classification branches and applies binary-classification loss to encourage each branch to learn only the degraded single-class features. In addition, based on the mutual exclusion between classes, we propose a mutual exclusion constraint (FD-Head-M) module to constrain the scores of all classes, promoting the detector performance. Finally, we replace the original convolution with more powerful feature extraction modules to form the enhanced FD-Head (FD-Head-E). Notably, our method can be used as a universal module and embedded into the existing object detectors to boost their performance. When applying our method to typical object detectors, it experimentally achieves performance gains of 1.2–2.2%, 1.7–2.5% on the KITTI-3, SeaShips datasets respectively. When using ResNet50 as the backbone network, our method gains an accuracy of 45.9% on the MS COCO dataset.
更多查看译文
关键词
Feature degradation,Re-decoupling,Few-class scenes,Object detection,Mutual exclusion constraint
AI 理解论文
溯源树
样例
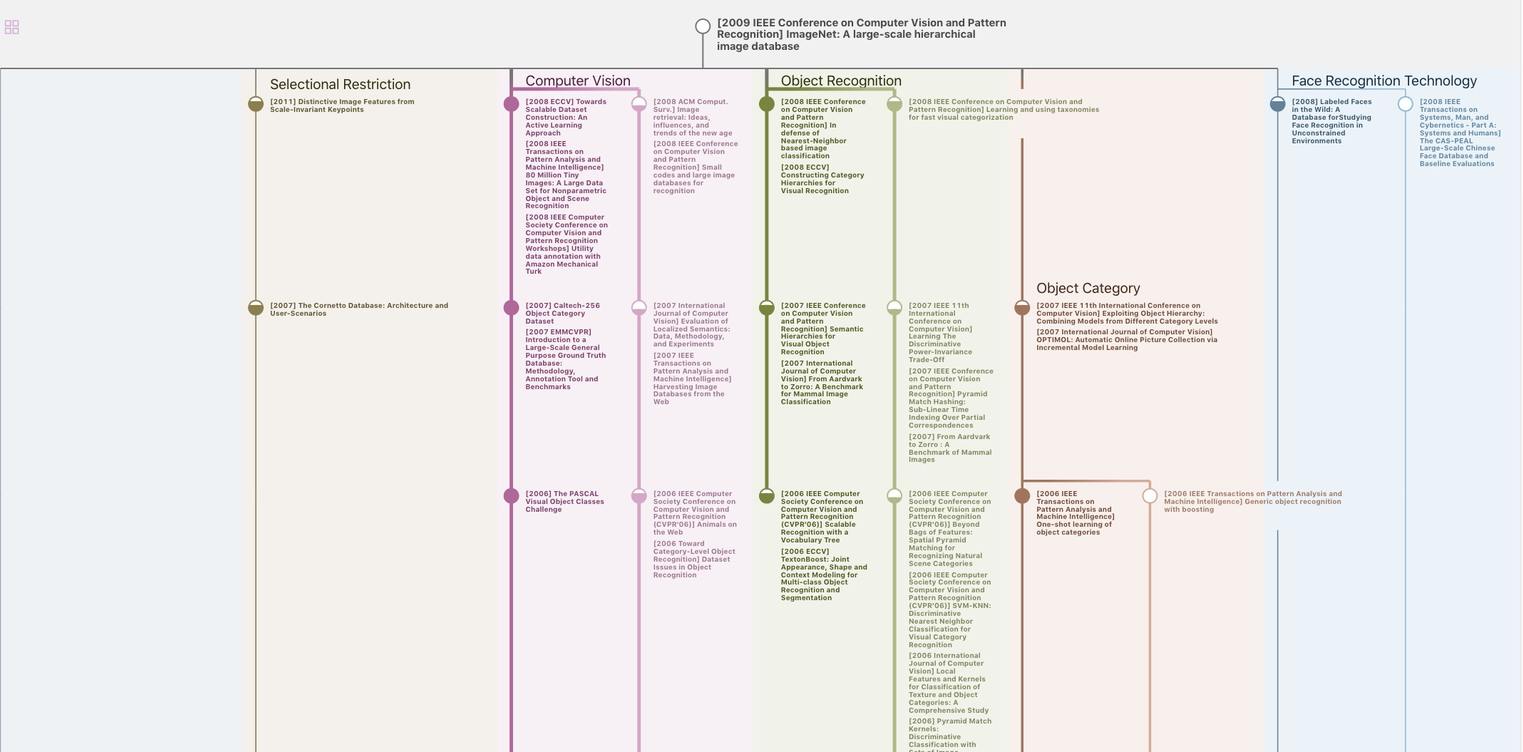
生成溯源树,研究论文发展脉络
Chat Paper
正在生成论文摘要