SeNAS-Net: Self-supervised Noise and Artifact Suppression Network for Material Decomposition in Spectral CT
IEEE Transactions on Computational Imaging(2024)
摘要
For material decomposition in spectral computed tomography, the x-ray attenuation coefficient of an unknown material can be decomposed as a combination of a group of basis materials, in order to analyze its material properties. Material decomposition generally leads to amplification of image noise and artifacts. Meanwhile, it is often difficult to acquire the ground truth values of the material basis images, preventing the application of supervised learning-based noise reduction methods. To resolve such problem, we proposed a self-supervised noise and artifact suppression network for spectral computed tomography. The proposed method consists of a projection domain self-supervised denoising network along with physics driven constraints to mitigate the secondary artifacts, including a noise modulation item to incorporate the anisotropic noise amplitudes in the projection domain, a sinogram mask image to suppress streaky artifacts and a data fidelity loss item to further mitigate noise and to improve signal accuracy. The performance of the proposed method was evaluated based on both numerical experiment tests and laboratory experiment tests. Results demonstrated that the proposed method has promising performance in noise and artifact suppression for material decomposition in spectral computed tomography. Comprehensive ablation studies were performed to demonstrate the function of each physical constraint.
更多查看译文
关键词
Spectral CT,Material decomposition,Neural network,Self-supervised Learning,Photon Counting CT
AI 理解论文
溯源树
样例
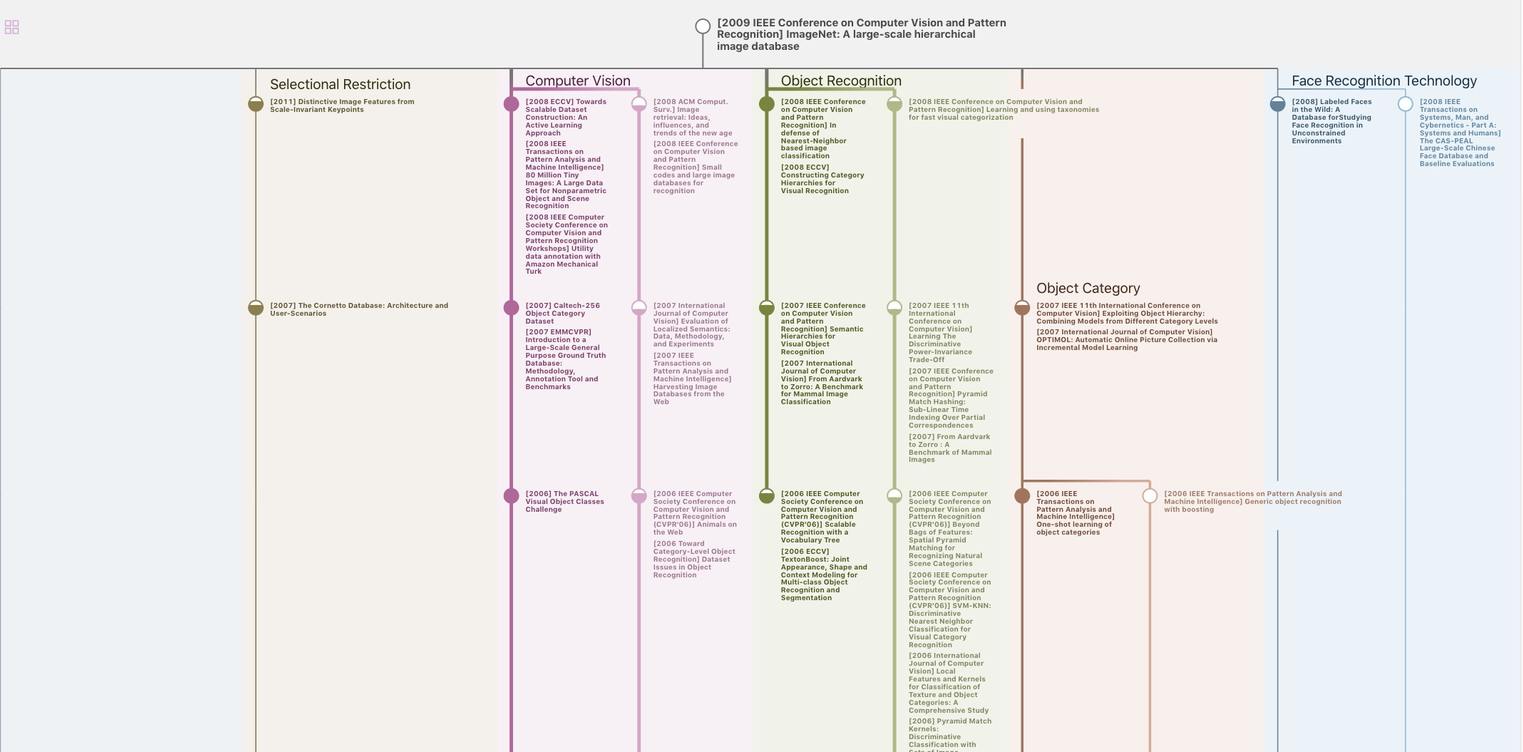
生成溯源树,研究论文发展脉络
Chat Paper
正在生成论文摘要