FVFL: A Flexible and Verifiable Privacy-Preserving Federated Learning Scheme
IEEE Internet of Things Journal(2024)
摘要
With the development of deep learning, people are more and more concerned about the security of data. Federated learning can solve the problem of data island, but it also brings more serious data privacy problems. Furthermore, in the process of multi-source data collaboration, the efficiency of the whole federated learning system is usually not high. In this paper, we introduce a scheme named FVFL, which ensure the local data security and resistance to collusive attacks, more importantly it can well support client flexible participate federated learning. we adopt Paillier encryption and Secret sharing to guaranttee client’s data security and resistance to collusive attacks; Moreover, our encryption mechanism allows client to participate in federated learning flexibly, and the correctness of the encryption algorithm is not affected by client’s drop out. The super-increasing sequence is introduced to reduce the communication overhead of the whole system, the simulation result shows that the result is significant; the Lagrange interpolation polynomial and secret Sharing is introduced to implement verification mechansim, to prevent malicious forgery of aggregation results in the cloud.The verification mechanism ensures the clients to obtain real and reliable aggregation results in the cloud. Moreover, our verification mechanism allows client to participate in federated learning flexibly, and the correctness of the verification algorithm is not affected by client’s drop out. And the experimental results show that FVFL has high accuracy and efficiency.
更多查看译文
关键词
Privacy-preserving,Federated learning,Verifiable,Deep Learning
AI 理解论文
溯源树
样例
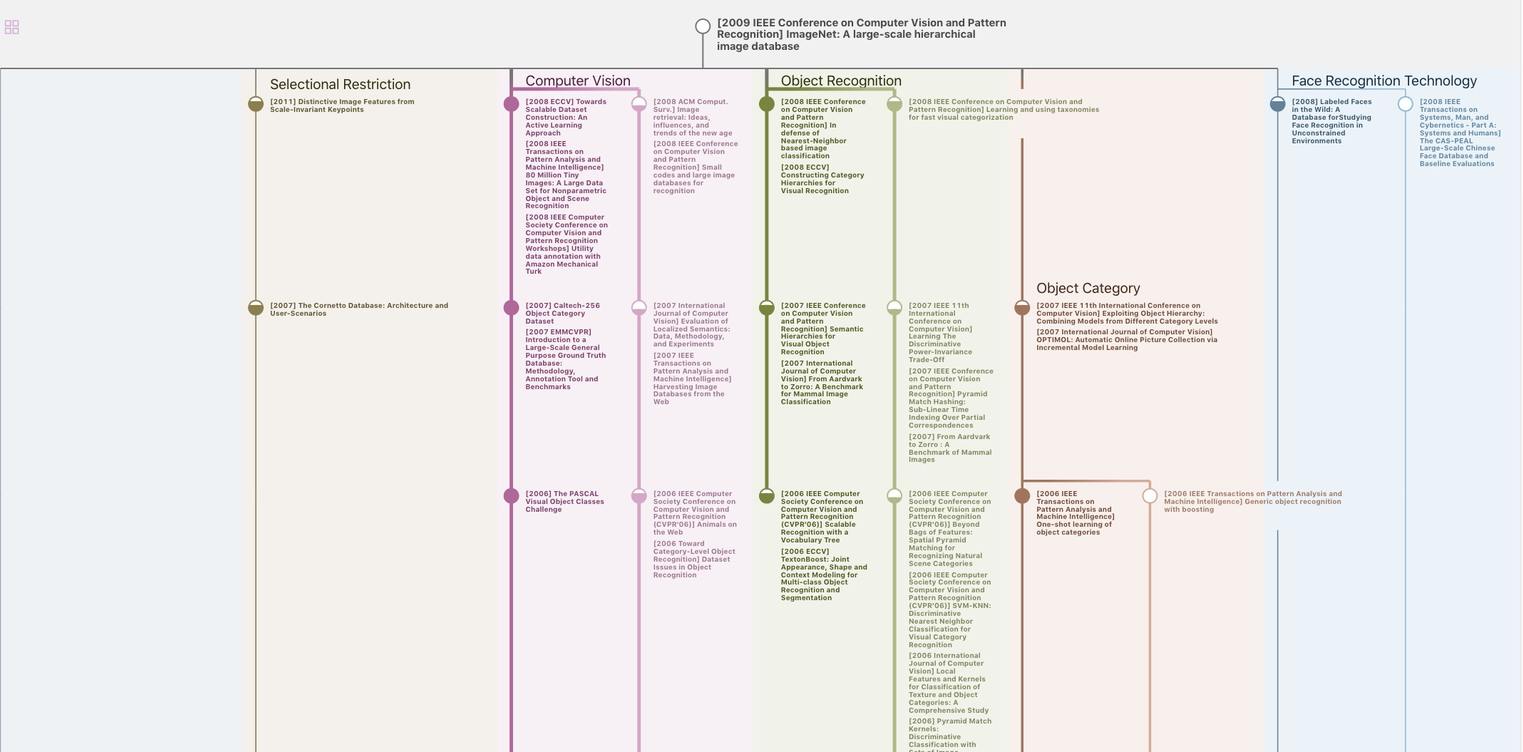
生成溯源树,研究论文发展脉络
Chat Paper
正在生成论文摘要