Improving Representation With Hierarchical Contrastive Learning for Emotion-Cause Pair Extraction
IEEE Transactions on Affective Computing(2024)
摘要
Emotion-cause pair extraction (ECPE) aims to extract emotions and their corresponding cause from a document. The previous works have made great progress. However, there exist two major issues in existing works. First, most existing works mainly focus on the semantic relation between the emotion clause and cause clause, ignoring their inner statistical relation in representation space. Second, the existing works are sensitive to the relative position between the emotion clause and cause clause, which damages the model's robustness. To address the two issues, we propose a hierarchical contrastive learning framework (HCL-ECPE), which hierarchically performs contrastive learning on representation from two levels. The first level is inter-clause contrastive learning (ICCL), which performs between emotion clause and cause clause through mutual information maximization. The second level is intra-pair contrastive learning (IPCL), which performs between clause representation and pair representation through contrastive predictive coding (CPC). HCL-ECPE integrates ICCL and IPCL modules to explore the statistical relations between the emotion clause, cause clause, and their constructed emotion-cause pair from the perspective of mutual information, thereby improving the model performance and robustness. Experimental results on two public datasets, ECPED and RECCON, demonstrate that HCL-ECPE outperforms the most competitive baselines. Furthermore, ICCL and IPCL are orthogonal to the existing model, and introducing them into the current models updates state-of-the-art performance.
更多查看译文
关键词
Contrastive predictive coding,emotion-cause pair extraction,hierarchical contrastive learning,mutual information
AI 理解论文
溯源树
样例
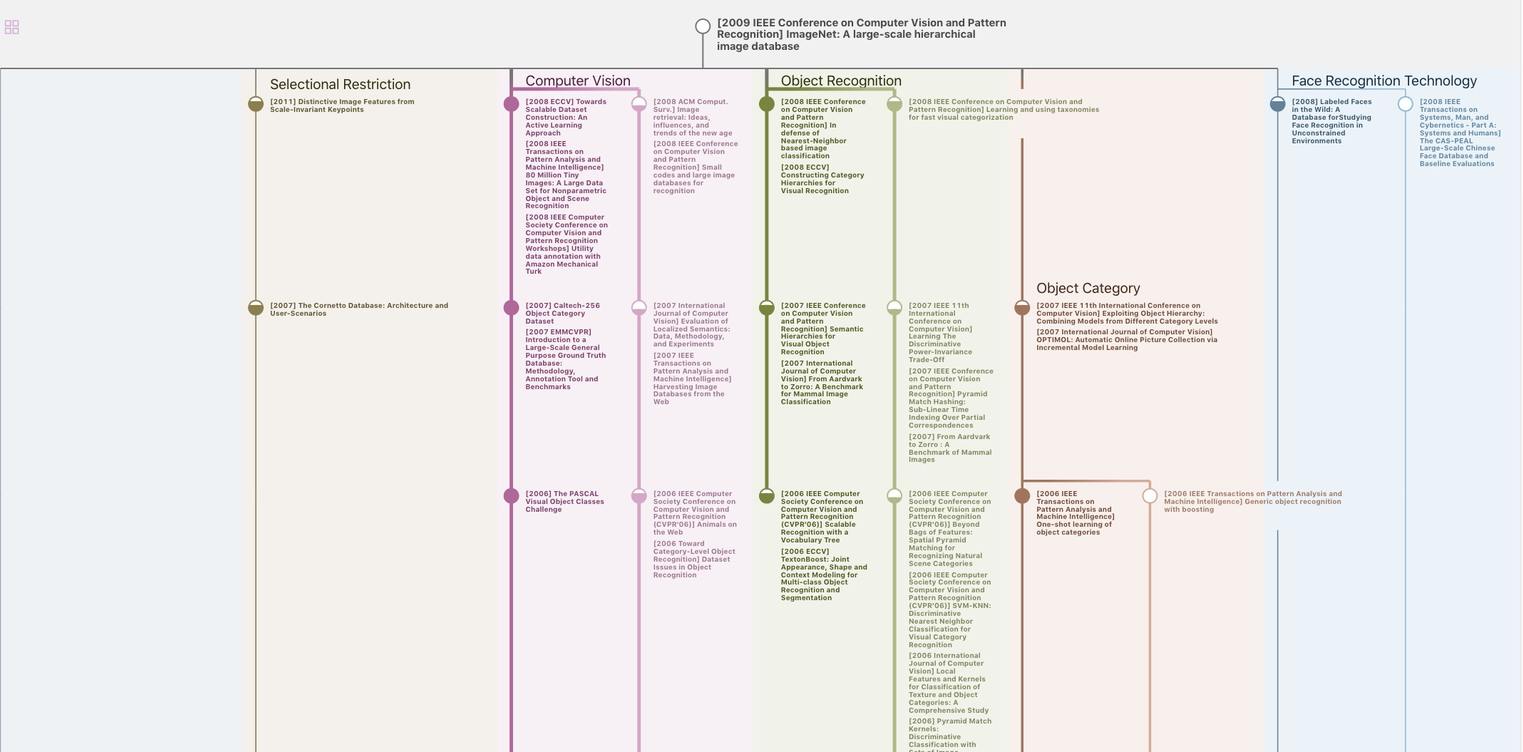
生成溯源树,研究论文发展脉络
Chat Paper
正在生成论文摘要