The Landscape of Unfolding with Machine Learning
arxiv(2024)
摘要
Recent innovations from machine learning allow for data unfolding, without
binning and including correlations across many dimensions. We describe a set of
known, upgraded, and new methods for ML-based unfolding. The performance of
these approaches are evaluated on the same two datasets. We find that all
techniques are capable of accurately reproducing the particle-level spectra
across complex observables. Given that these approaches are conceptually
diverse, they offer an exciting toolkit for a new class of measurements that
can probe the Standard Model with an unprecedented level of detail and may
enable sensitivity to new phenomena.
更多查看译文
AI 理解论文
溯源树
样例
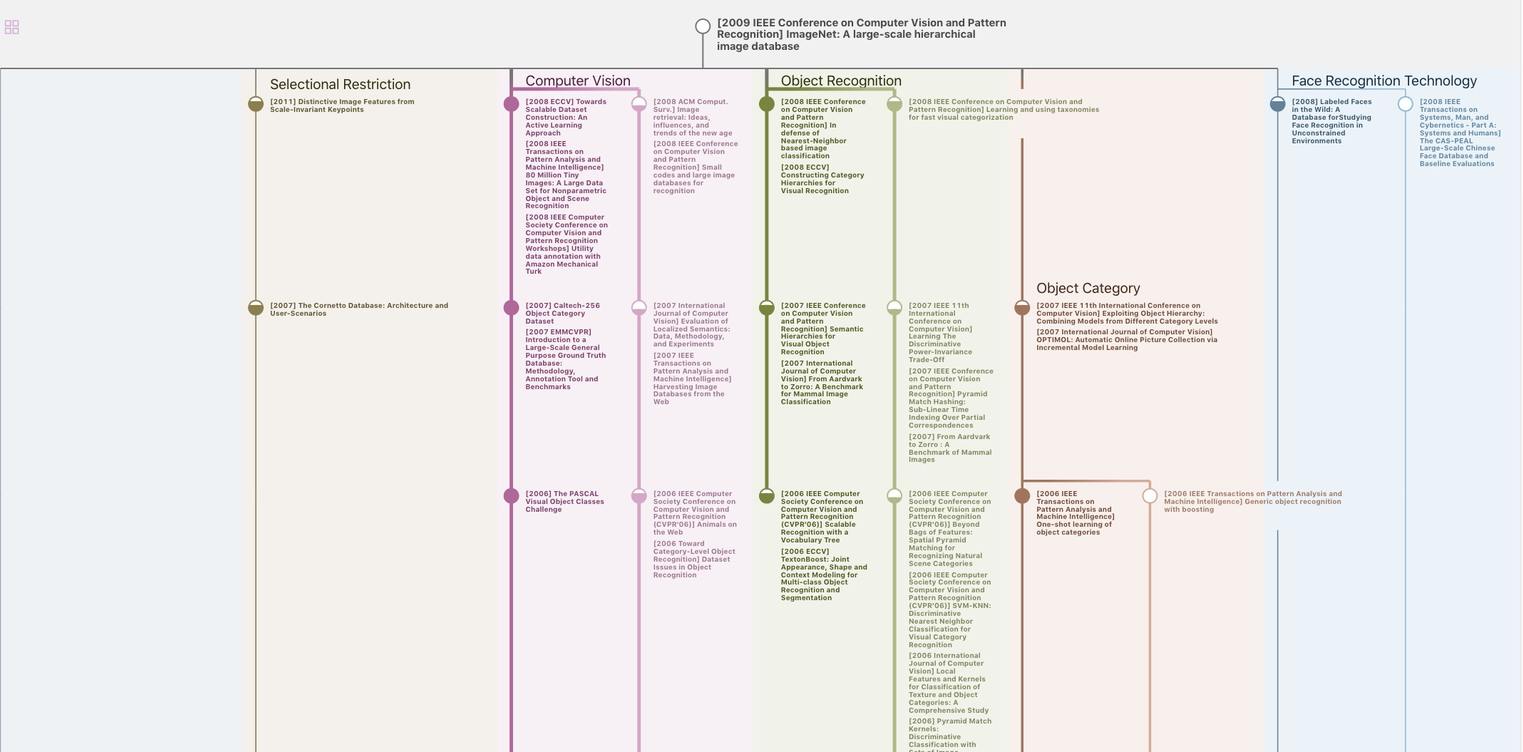
生成溯源树,研究论文发展脉络
Chat Paper
正在生成论文摘要