Capabilities of Gemini Models in Medicine
arxiv(2024)
摘要
Excellence in a wide variety of medical applications poses considerable
challenges for AI, requiring advanced reasoning, access to up-to-date medical
knowledge and understanding of complex multimodal data. Gemini models, with
strong general capabilities in multimodal and long-context reasoning, offer
exciting possibilities in medicine. Building on these core strengths of Gemini,
we introduce Med-Gemini, a family of highly capable multimodal models that are
specialized in medicine with the ability to seamlessly use web search, and that
can be efficiently tailored to novel modalities using custom encoders. We
evaluate Med-Gemini on 14 medical benchmarks, establishing new state-of-the-art
(SoTA) performance on 10 of them, and surpass the GPT-4 model family on every
benchmark where a direct comparison is viable, often by a wide margin. On the
popular MedQA (USMLE) benchmark, our best-performing Med-Gemini model achieves
SoTA performance of 91.1
strategy. On 7 multimodal benchmarks including NEJM Image Challenges and MMMU
(health medicine), Med-Gemini improves over GPT-4V by an average relative
margin of 44.5
capabilities through SoTA performance on a needle-in-a-haystack retrieval task
from long de-identified health records and medical video question answering,
surpassing prior bespoke methods using only in-context learning. Finally,
Med-Gemini's performance suggests real-world utility by surpassing human
experts on tasks such as medical text summarization, alongside demonstrations
of promising potential for multimodal medical dialogue, medical research and
education. Taken together, our results offer compelling evidence for
Med-Gemini's potential, although further rigorous evaluation will be crucial
before real-world deployment in this safety-critical domain.
更多查看译文
AI 理解论文
溯源树
样例
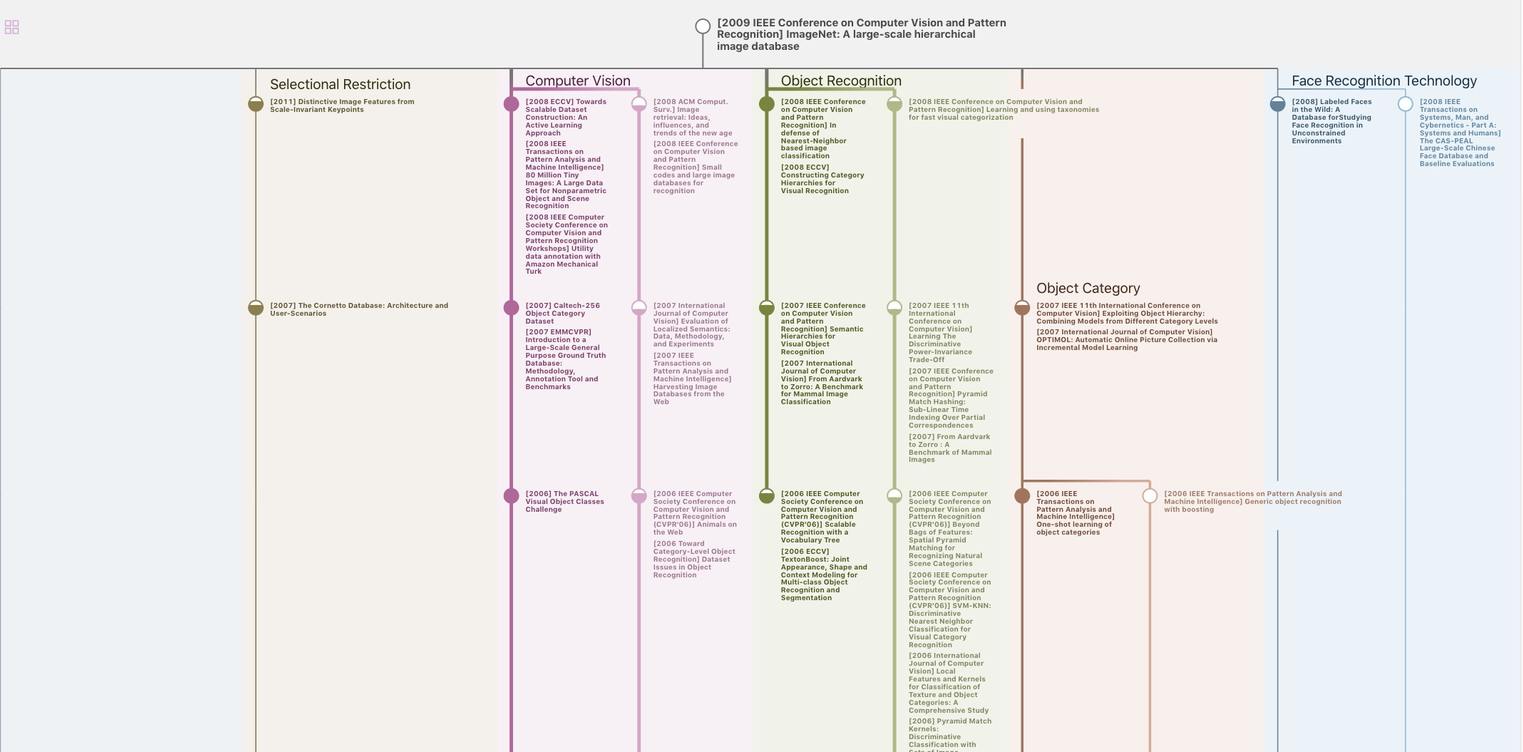
生成溯源树,研究论文发展脉络
Chat Paper
正在生成论文摘要