Transformer For Low-frequency Extrapolating of Seismic Data
arxiv(2024)
摘要
Full waveform inversion (FWI) is used to reconstruct the physical properties
of subsurface media which plays an important role in seismic exploration.
However, the precision of FWI is seriously affected by the absence or
inaccuracy of low-frequency information. Therefore, reconstructing the
low-frequency signals accurately is highly significant in seismic data
processing. Low-frequency extrapolation of seismic records can be approached as
a deep learning regression problem. Thus, to obtain low-frequency information
from band-limited seismic records, a novel network structure called
low-frequency extrapolation transformer (LFET) is proposed to construct the
nonlinear mapping relationship between the data missing low-frequency and
low-frequency data in a supervised learning approach, which is inspired by the
transformer model widely used in natural language processing (NLP). We apply
multi-head self-attention (MSA) modules to model the remote dependencies of
seismic data. Based on this, we introduce a shifted window partitioning
approach to reduce the calculating amount. Due to the field data are not
suitable for supervised learning, we generate synthetic seismic records using
submodels selected from the benchmark Marmousi model as training data whose
characteristics are similar to that of the field data. A single trace of
synthetic band-limited seismic data in the time domain is used as the input
data, and the parameters of LFET are updated based on the errors between the
predicted trace and the corresponding label. The experimental results on the
data generated by different models, different wavelets, and different kinds of
field marine data demonstrate the feasibility and generalization of the
proposed method. Furthermore, the proposed method achieves higher accuracy with
lower computational expense than the traditional CNN method.
更多查看译文
AI 理解论文
溯源树
样例
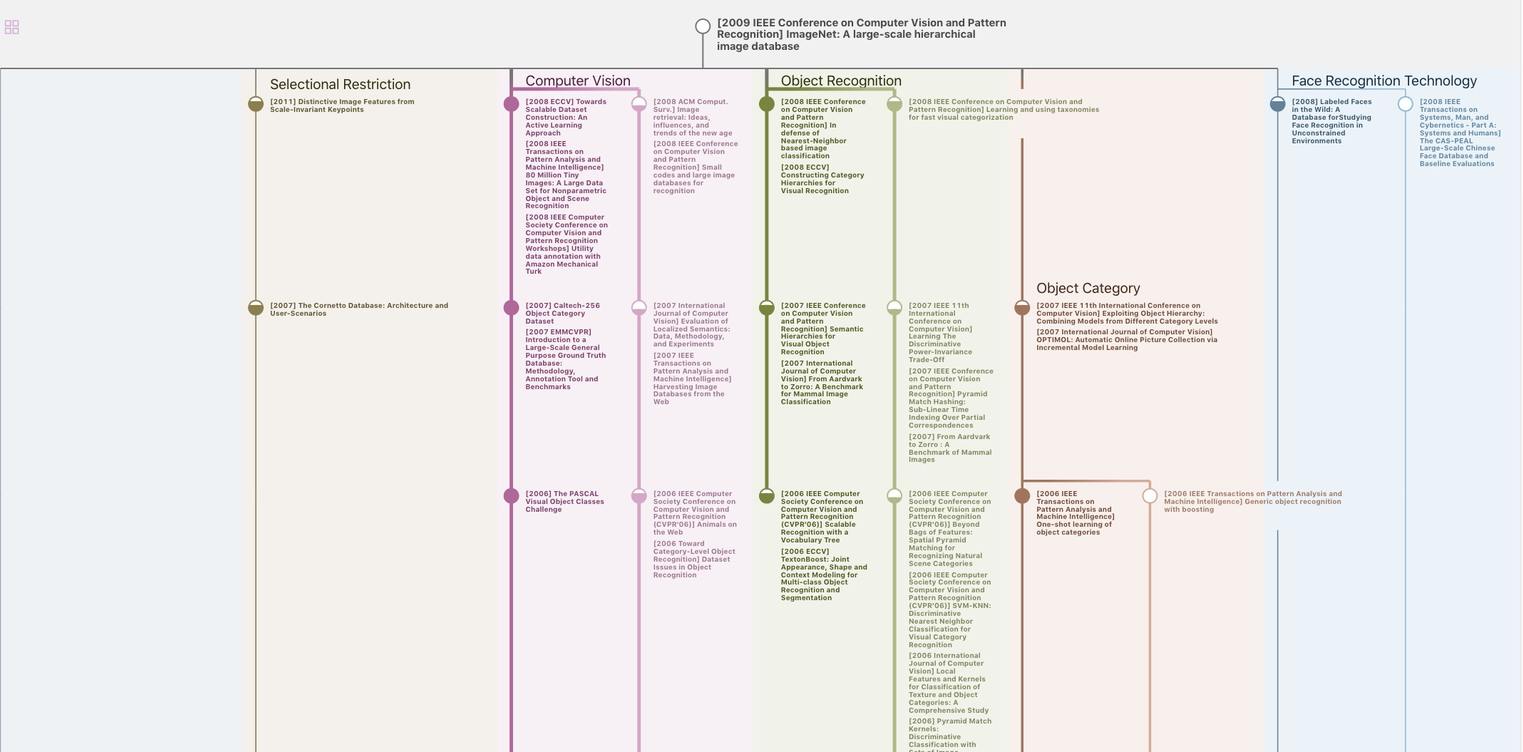
生成溯源树,研究论文发展脉络
Chat Paper
正在生成论文摘要