Spatiotemporal synchronous dynamic graph attention network for traffic flow forecasting
Neural Computing and Applications(2024)
摘要
Traffic flow forecasting (TFF) is crucial for effective urban planning and traffic management. Most modeling approaches in TFF ignore the dynamic characteristics of the transportation network topology, which results in an inability to accurately capture the hidden spatiotemporal correlations. To this end, we investigate a Spatiotemporal Synchronous Dynamic Graph Attention Network (STS-DGAT) for TFF. STS-DGAT is composed of three parts: the dynamic feature enhancement (DFE) module, the spatiotemporal coupling (STC) module, and the temporal position embedding (TPE) module. Specifically, we discover the impact of traffic data features on TFF based on the DFE module, assign dynamic weights of various features for time steps, and adjust the intrinsic relevance of traffic data. Then, we propose the STC module to characterize the complex coupling relationships of road network nodes in spatial and temporal dimensions and the dynamic intrinsic interactions of spatiotemporal correlations. The STC module comprises a dynamic graph attention network (DGAT) and an adaptive gated temporal convolutional network (AGTCN). Deep characterization for the dynamic topology of road networks is mined by DGAT, which captures real-time dynamic spatial correlations, and hidden features in the nonlinear temporal dimension are extracted by AGTCN to learn long-term temporal dependency. Finally, we put forward a TPE module to incorporate temporal position information into spatiotemporal relationships and adaptively learn hidden features of individual nodes to understand spatiotemporal variation features effectively. Experimental results from four real-world datasets demonstrate that the STS-DGAT model outperforms other baseline models.
更多查看译文
关键词
Big data analytics,Traffic flow forecasting,Dynamic graph neural network,Multidimensional self-attention
AI 理解论文
溯源树
样例
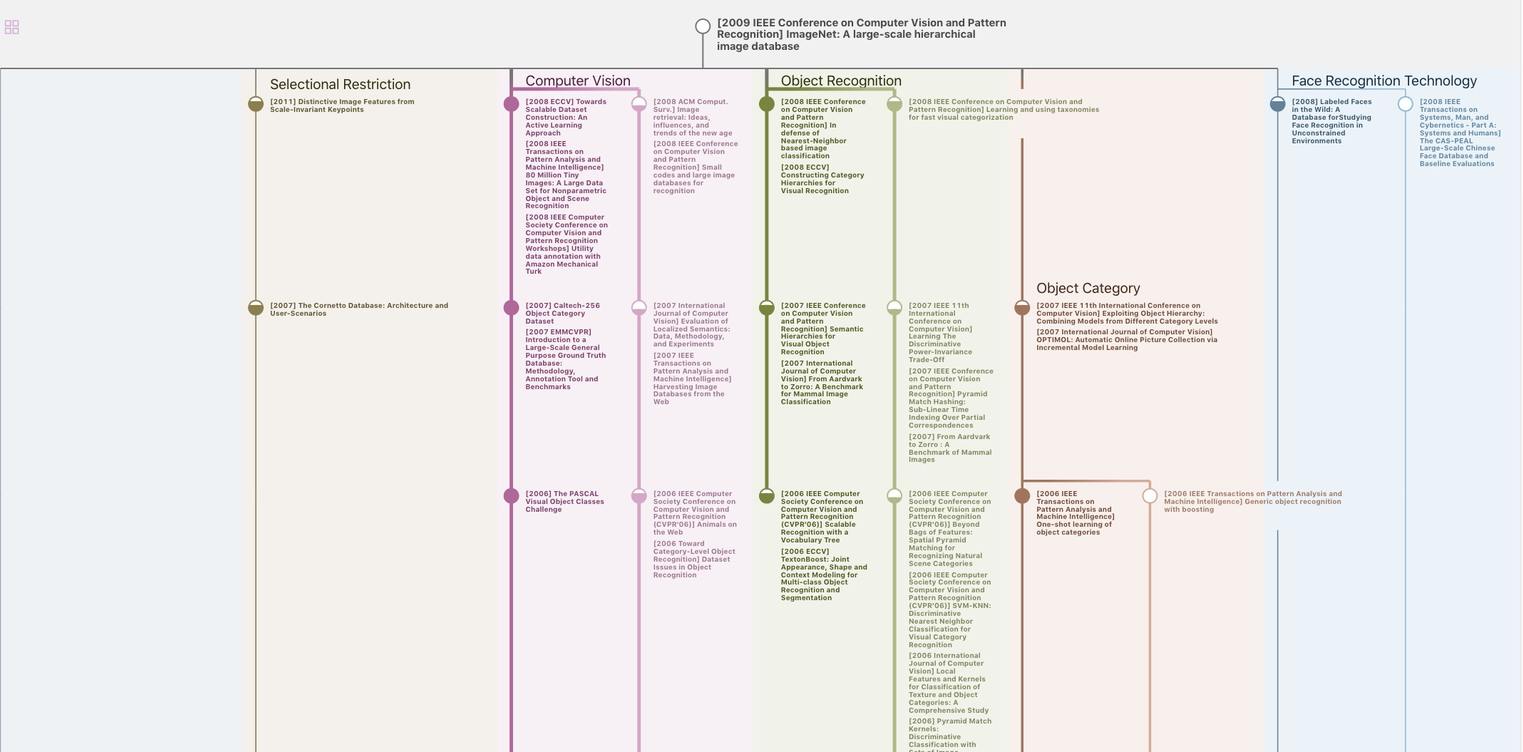
生成溯源树,研究论文发展脉络
Chat Paper
正在生成论文摘要