Interpretable machine learning of SPAC system via a mechanism-assisted gaussian process group: Representation of the system mechanism by data
Computers and Electronics in Agriculture(2024)
摘要
Traditional models of the soil-plant-atmosphere continuum (SPAC) system are physics-based and their practical application has been hindered by issues of high parameterization, prior structural bias, and costly running. In this paper, we developed a machine learning modelling method based on the Gaussian process (GP) to avoid these difficulties of traditional modelling. At the same time, shortcomings of conventional machine learning in terms of interpretability and mechanism representation were addressed. The innovative method consisted of structuring a local GP group framework and improving kernel functions used in each local GP. The resultant model was endowed with advanced capacities, including representing interplays between subprocesses within the complex SPAC system, representing nonstationary subprocess dynamics caused by crop growth stage shifts, as well as automatically interpreting key low-order input interactions and dominant input variables for each subprocess. The performance of our model was examined on synthetic SPAC system datasets that covered three different soil conditions. Results demonstrated that its interpretations regarding subprocess mechanisms were robust across different soil conditions and consistent with domain knowledge. Compared to a conventional global GP model and two deep learning models (DNN and LSTM), our model performed significantly better not only in regular prediction experiments but also in generalization experiments that required models to be transferred across different conditions. Our study suggested that it is promising to enable a machine learning model interpretable by improving its feature representation function. For machine learning modelling of complex systems like the SPAC, it may be more important to enhance the model’s mechanism representation capability than simply using deeper networks.
更多查看译文
关键词
Machine learning,Interpretability,Mechanism representation,Gaussian process,SPAC system
AI 理解论文
溯源树
样例
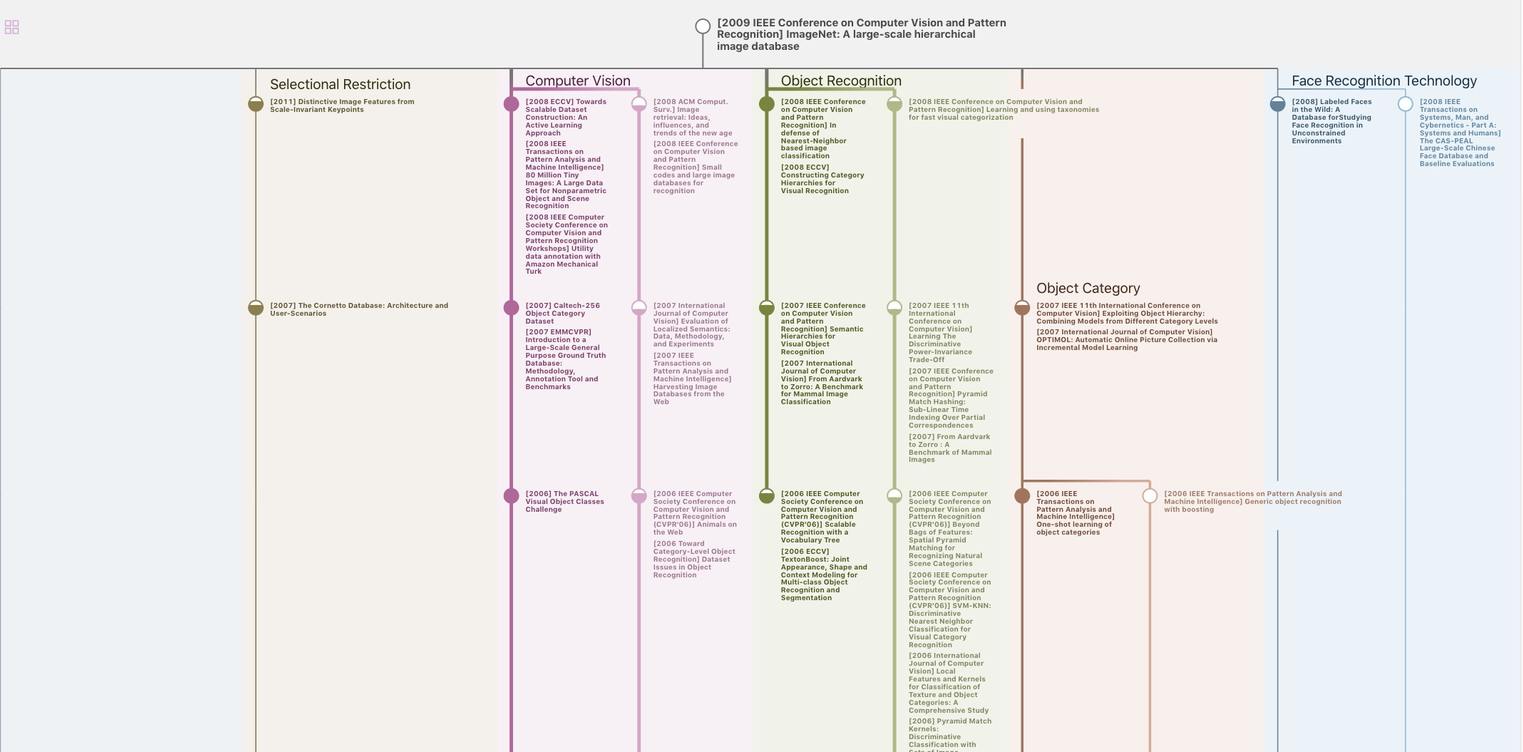
生成溯源树,研究论文发展脉络
Chat Paper
正在生成论文摘要