Reducing Voltage Losses in Organic Photovoltaics Requires Interfacial Disorder Management
ADVANCED ENERGY MATERIALS(2024)
摘要
Thanks to the introduction of non-fullerene acceptors, efficiencies of organic photovoltaics are now approaching 20%. Closing the gap with inorganic photovoltaics requires minimizing voltage losses without penalizing charge extraction, for which microstructure control is crucial. However, the complex interplay between microstructure and charge generation, recombination, and extraction has so far not been unraveled. Here, a systematic study linking device performance to distinct microstructural features via machine learning is presented. Building bi-layer devices allows to separately study the influence of aggregation and disorder on the energies and lifetimes of bulk and interfacial states. Unambiguous assignments of specific structural motifs to the device photophysics are thus possible. It is found that the control of aggregation-caused quenching is decisive for the exciton splitting efficiency and thus the carrier generation. Furthermore, the static disorder at the donor-acceptor interface controls the nonradiative recombination by shifting the excited state population from the bulk toward the interface. Finally, the amount of disorder in the bulk is found decisive for charge extraction. The finding that charge generation, recombination, and extraction are controlled by distinct structural features, is the key to optimizing these motifs independently, which will pave the way for organic photovoltaics toward the detailed balance limit. In this work, the aggregation-related photophysical properties of bulk and interfacial states are disentangled through the construction of bilayer organic solar cells. Machine learning is used to enable the assignment of specific structural motifs to elementary device photophysics. The results highlight those different aspects of structural features control loss in voltage, current, and fill factor. image
更多查看译文
关键词
interfacial energy states,machine learning,microstructure,organic solar cells,voltage loss
AI 理解论文
溯源树
样例
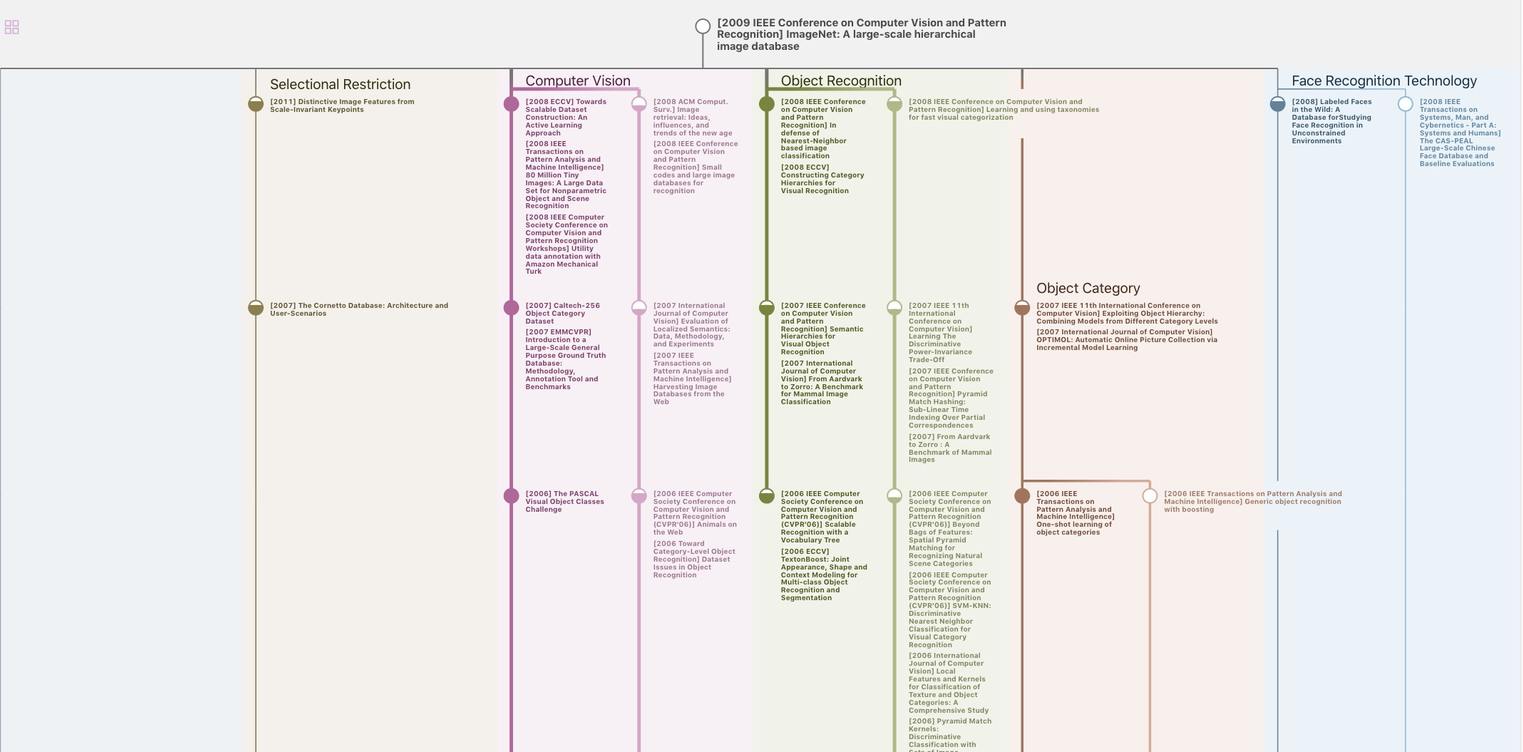
生成溯源树,研究论文发展脉络
Chat Paper
正在生成论文摘要