TANet: Text region attention learning for vehicle re-identification
Engineering Applications of Artificial Intelligence(2024)
摘要
In recent years, the challenge of distinguishing vehicles of the same model has prompted a shift towards leveraging both global appearances and local features, such as lighting and rearview mirrors, for vehicle re-identification (ReID). Despite advancements, accurately identifying vehicles remains complex, particularly due to the underutilization of highly discriminative text regions. This paper introduces the Text Region Attention Network (TANet), a novel approach that integrates global and local information with a specific focus on text regions for improved feature learning. TANet uniquely captures stable and distinctive features across various vehicle views, demonstrating its effectiveness through rigorous evaluation on the VeRi-776, VehicleID, and VERI-Wild datasets. TANet significantly outperforms existing methods, achieving mAP scores of 83.6% on VeRi-776, 84.4% on VehicleID (Large), and 76.6% on VERI-Wild (Large). Statistical tests further validate the superiority of TANet over the baseline, showcasing notable improvements in mAP and Top-1 through Top-15 accuracy metrics.
更多查看译文
关键词
Vehicle re-identification,Part attention,Text region
AI 理解论文
溯源树
样例
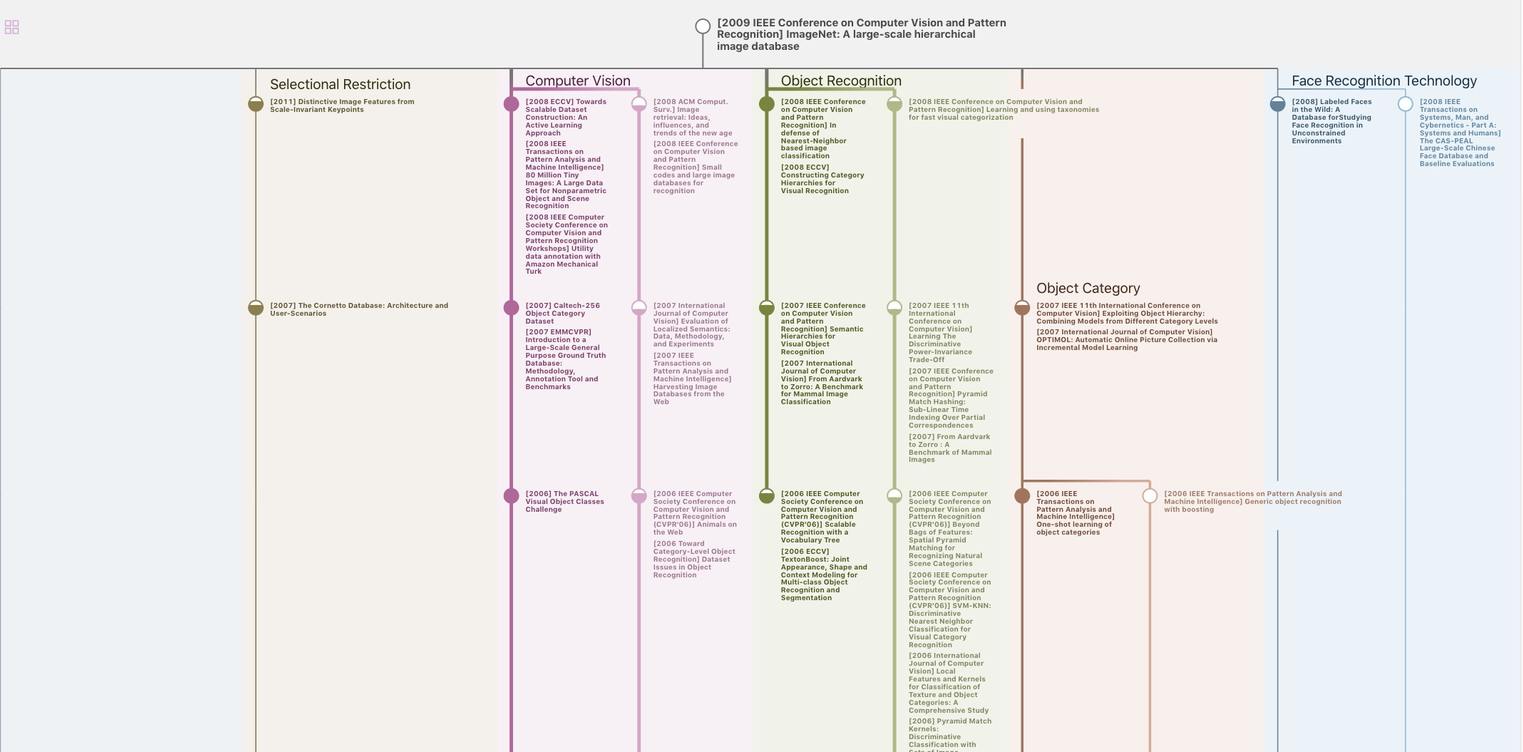
生成溯源树,研究论文发展脉络
Chat Paper
正在生成论文摘要