SECURENET: Advancing Cybersecurity Knowledge Graphs with Multi-Feature Perceptrons and Hierarchical Adversarial W2NER
2023 5th International Academic Exchange Conference on Science and Technology Innovation (IAECST)(2023)
摘要
To meet the demand for improving the construction and analysis efficiency of knowledge graphs in the field of cybersecurity, this paper introduces an innovative system—SECURENET. SECURENET successfully addresses the issue of a lack of annotated text datasets in the field of cybersecurity and effectively tackles the challenges of flat, overlapping, and discontinuous entity recognition in cybersecurity texts. The SECURENET system consists of three core modules: First, the Multifeature Security Averaged Perceptron (MSAP) module combines two types of multi-feature perceptron technologies to deeply analyze and understand complex features in cybersecurity texts, such as semantic and structural properties, and perform corresponding label annotations. Secondly, the entity extraction module uses the annotated dataset to train the Named Entity Recognition (NER) model. We employ the SecBERT pre-trained model combined with the W2NER framework, a unified NER neural network framework. Furthermore, SECURENET introduces an innovative Multi-Level Adversarial Training (MLAT) strategy to enhance the accuracy and robustness in the entity extraction process. Finally, the relationship extraction module defines a security ontology to extract relationships between entities, thereby forming the VulKG knowledge graph. In experiments, each module of the SECURENET system demonstrated excellent performance.
更多查看译文
关键词
automatic labeling,Named Entity Recognition,Knowledge graph,cybersecurity Tasks
AI 理解论文
溯源树
样例
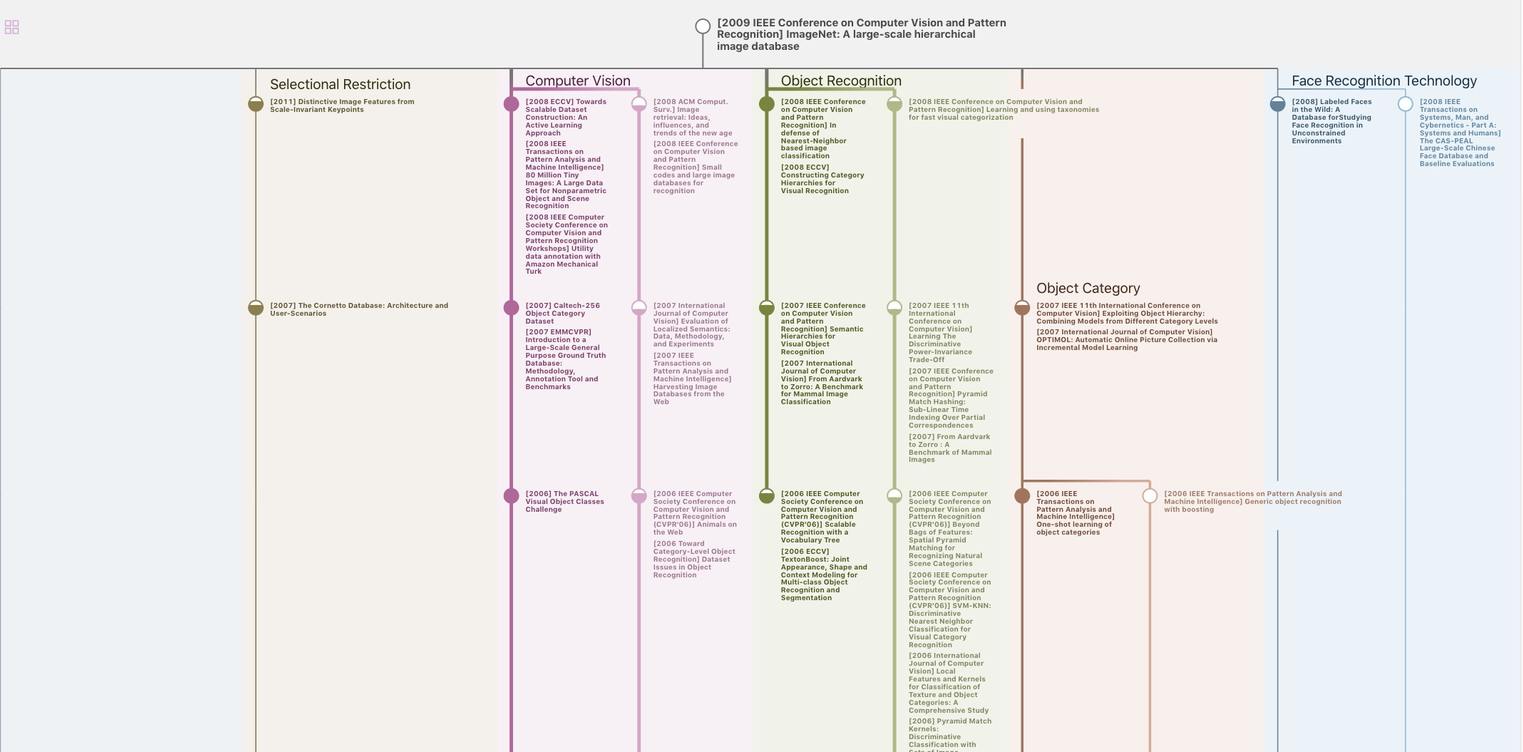
生成溯源树,研究论文发展脉络
Chat Paper
正在生成论文摘要