Bridging Machine Learning and Diagnostics of the ESA LISA Space Mission with Equation Discovery via Explainable Artificial Intelligence
Advances in Space Research(2024)
摘要
The Laser Interferometer Space Antenna (LISA) of the European Space Agency will be the first interferometer for low-frequency (10-4–10-1 Hz) gravitational wave detection in space. LISA will detect spurious accelerations of the test masses, the end mirrors of the interferometer, of the order of femto-g. Amongst spurious signals, Coulomb forces due to stray electric fields coupling with charge deposited on the test masses by galactic and solar particles and the noise associated to the charging process must be monitored during the mission lifetime. Precious clues on spurious forces acting on the test masses have been studied with the LISA Pathfinder mission, meant for the testing of the instrumentation that will be placed on board LISA, in 2016–2017. In this work we present the design and implementation of a workflow leading to equation discovery for the relationship between solar wind speed, galactic cosmic-ray variations and interplanetary magnetic field intensity observations for the diagnostics of LISA. The workflow exploits explainable artificial intelligence tools to build a bridge between the opaque predictions obtained with machine learning (ML) models and data analysis performed by humans with space mission observations. The core step of the workflow is the implementation, tuning and optimisation of an opaque ML regressor explicitly designed for the future LISA mission, based on input observations of the galactic cosmic-ray flux and of the interplanetary magnetic field intensity. The regressor is aimed at reconstructing the solar wind speed, a parameter of fundamental importance, but not available, for the mission environment monitoring. The workflow ends with the application of explainable clustering techniques to the opaque model in order to (i) obtain human-interpretable outputs instead of opaque ones without any noticeable loss in the predictive performance and (ii) discover an equation describing the quantitative relationship between the involved variables, currently missing in the literature. The correlation amongst the transit of interplanetary structures, galactic cosmic-ray variations and LISA test-mass charging is illustrated here.
更多查看译文
关键词
Space interferometers,LISA,Machine learning,Explainable clustering
AI 理解论文
溯源树
样例
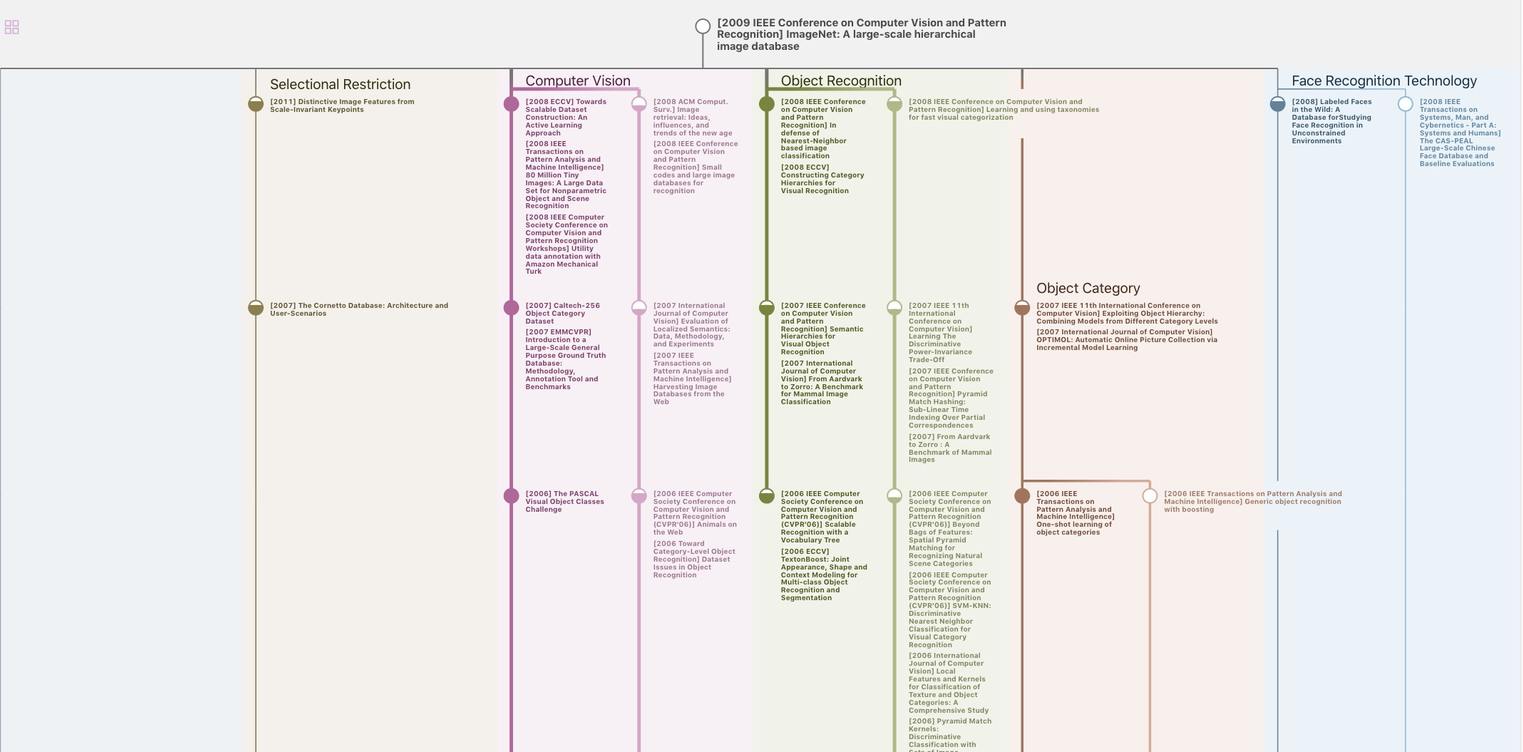
生成溯源树,研究论文发展脉络
Chat Paper
正在生成论文摘要