Toward the Categorical Data Map
arxiv(2024)
摘要
Categorical data does not have an intrinsic definition of distance or order,
and therefore, established visualization techniques for categorical data only
allow for a set-based or frequency-based analysis, e.g., through Euler diagrams
or Parallel Sets, and do not support a similarity-based analysis. We present a
novel dimensionality reduction-based visualization for categorical data, which
is based on defining the distance of two data items as the number of varying
attributes. Our technique enables users to pre-attentively detect groups of
similar data items and observe the properties of the projection, such as
attributes strongly influencing the embedding. Our prototype visually encodes
data properties in an enhanced scatterplot-like visualization, encoding
attributes in the background to show the distribution of categories. In
addition, we propose two graph-based measures to quantify the plot's visual
quality, which rank attributes according to their contribution to cluster
cohesion. To demonstrate the capabilities of our similarity-based approach, we
compare it to Euler diagrams and Parallel Sets regarding visual scalability and
show its benefits through an expert study with five data scientists analyzing
the Titanic and Mushroom datasets with up to 23 attributes and 8124 category
combinations. Our results indicate that the Categorical Data Map offers an
effective analysis method, especially for large datasets with a high number of
category combinations.
更多查看译文
AI 理解论文
溯源树
样例
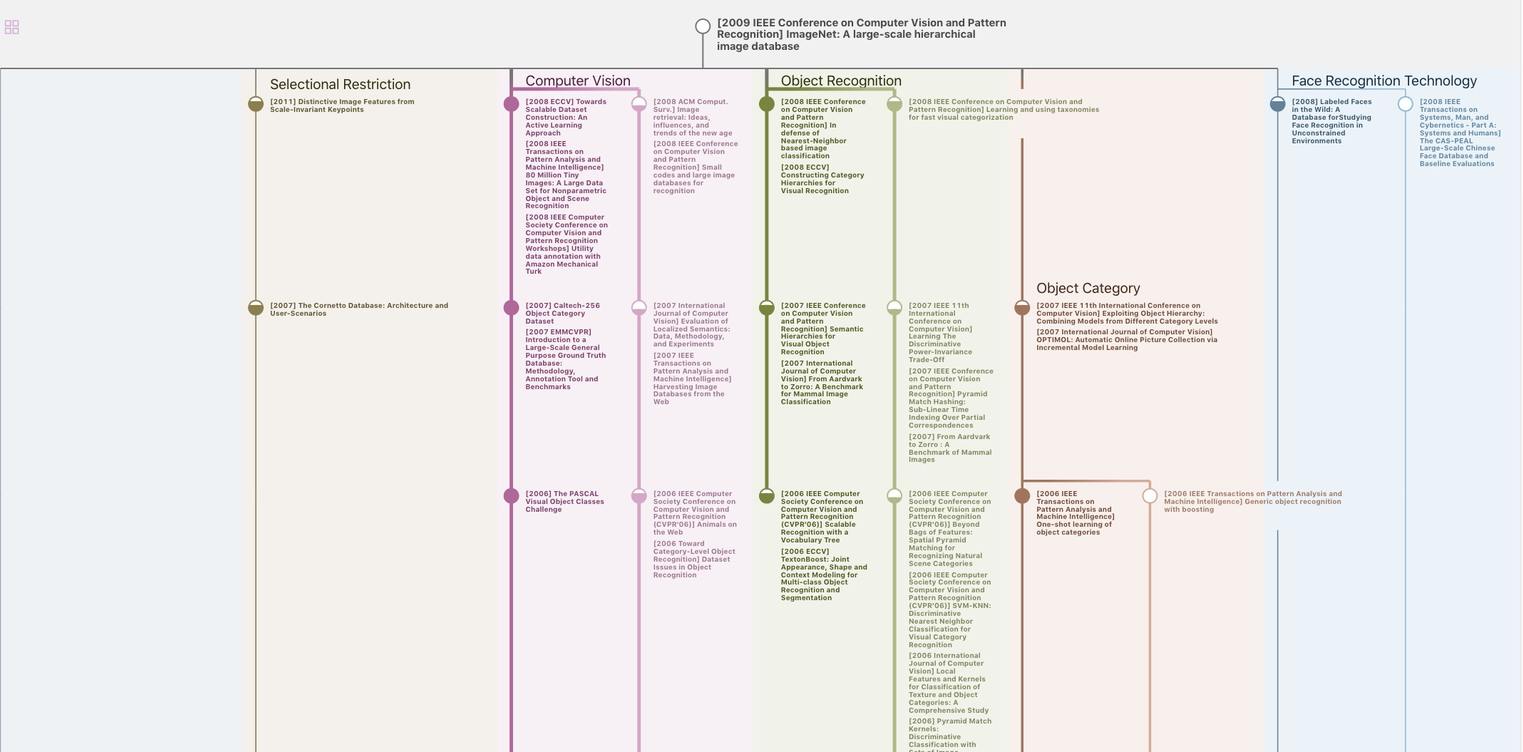
生成溯源树,研究论文发展脉络
Chat Paper
正在生成论文摘要