Semantic features analysis for biomedical lexical answer type prediction using ensemble learning approach
Knowledge and Information Systems(2024)
摘要
Lexical answer type prediction is integral to biomedical question–answering systems. LAT prediction aims to predict the expected answer’s semantic type of a factoid or list-type biomedical question. It also aids in the answer processing stage of a QA system to assign a high score to the most relevant answers. Although considerable research efforts exist for LAT prediction in diverse domains, it remains a challenging biomedical problem. LAT prediction for the biomedical field is a multi-label classification problem, as one biomedical question might have more than one expected answer type. Achieving high performance on this task is challenging as biomedical questions have limited lexical features. One biomedical question must be assigned multiple labels given these limited lexical features. In this paper, we develop a novel feature set (lexical, noun concepts, verb concepts, protein–protein interactions, and biomedical entities) from these lexical features. Using ensemble learning with bagging, we use the label power set transformation technique to classify multi-label. We evaluate the integrity of our proposed methodology on the publicly available multi-label biomedical questions dataset (MLBioMedLAT) and compare it with twelve state-of-the-art multi-label classification algorithms. Our proposed method attains a micro-F1 score of 77
更多查看译文
关键词
Multi-label text classification,Biomedical question classification,Feature engineering,Machine learning,Natural language processing (NLP),Lexical answer (LAT),Ensemble learning
AI 理解论文
溯源树
样例
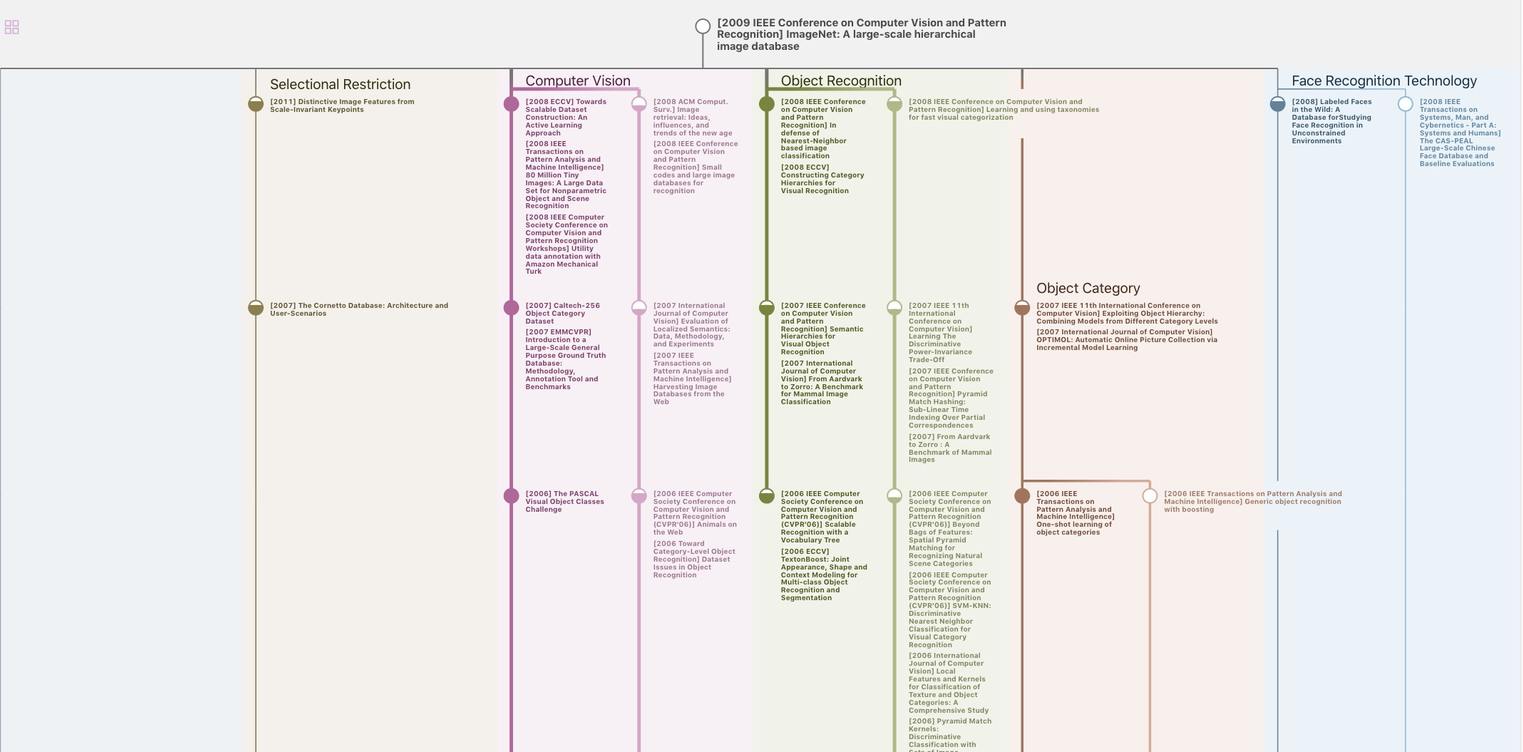
生成溯源树,研究论文发展脉络
Chat Paper
正在生成论文摘要