Extreme pressure coefficients: modelling a hydraulic jump using deep-learning based methods
Sādhanā(2024)
摘要
Hydraulic jump pressure field analysis is essential to assess slab stability in dissipation basins. This study is aimed to modelling non-dimensional extreme pressure coefficients of the minimum pressure fluctuation (CP−) and maximum pressure fluctuation (CP+) using experimental data with three deep learning (DL) techniques. Pressure coefficients were estimated using Convolutional Neural Network (CNN), Gated Recurrent Unit (GRU), and Long Short-Term Memory (LSTM) methods. For the CP− coefficient related to the tested dataset, the correlation coefficient (CC)=0.894 and Willmott’s index of agreement (WI)=0.944 are achieved using the LSTM model, compared with 0.892 and 0.936 for CNN; and 0.892 and 0.943 for GRU. For the CP+ coefficient, CC=0.910 and WI=0.950 are attained using the LSTM model, compared with 0.903 and 0.941 with CNN; and 0.905 and 0.945 with GRU. The results indicate the excellent performance of the LSTM model for estimating pressure coefficients. With laboratory conditions and the experimental data domain framework used in this study, it is shown that the DL models have been successful for modelling very complex systems. Therefore, DL techniques are recommended for estimating extreme pressure coefficients with a reduction in the need to perform expensive experiments.
更多查看译文
关键词
Hydraulic jump,C +,C −,CNN,GRU,LSTM
AI 理解论文
溯源树
样例
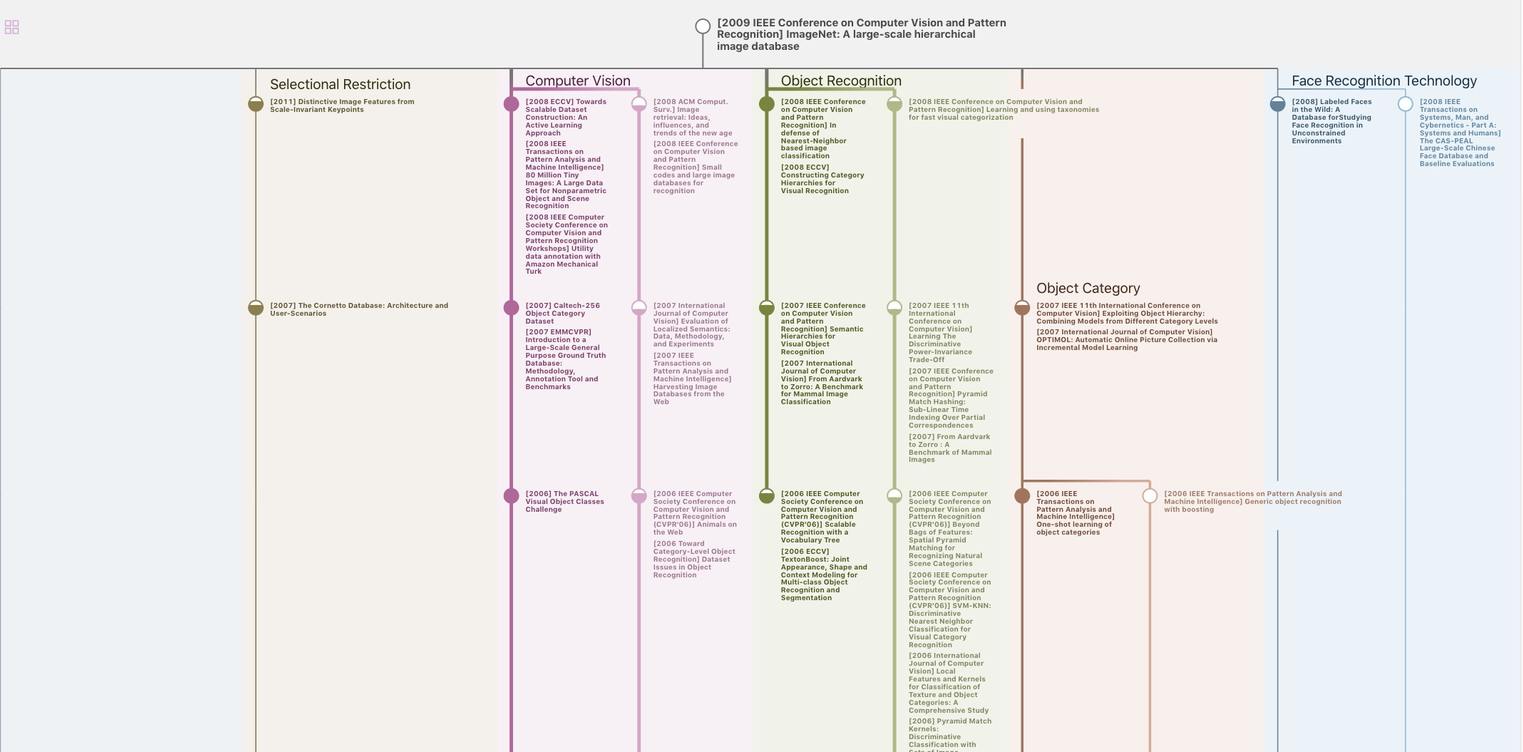
生成溯源树,研究论文发展脉络
Chat Paper
正在生成论文摘要