Feature Selection in High-Dimensional Space with Applications to Gene Expression Data
SoutheastCon 2024(2024)
摘要
Recent years have seen rapid growth in high-dimensional datasets. Most existing machine learning (ML) algorithms fail in high-dimensional settings where many features could be redundant. A critical process of feature selection is thus applied in such a setting that helps in identifying the most relevant features while removing redundant ones. With the increase in high dimensionality, one is also faced with problems of efficiency and interpretation in performing such selection methods. Therefore, this paper proposes a “novel” feature selection framework that uses an ensemble of interpretable ML algorithms to perform feature selection and the ranking of final features. Finally, this framework is applied to a gene expression dataset obtained through collaboration with the National Aeronautics and Space Administration (NASA)'s Biological and Physical Sciences (BPS) team and helps identify important and relevant genes contributing to specific target attributes through classification tasks.
更多查看译文
AI 理解论文
溯源树
样例
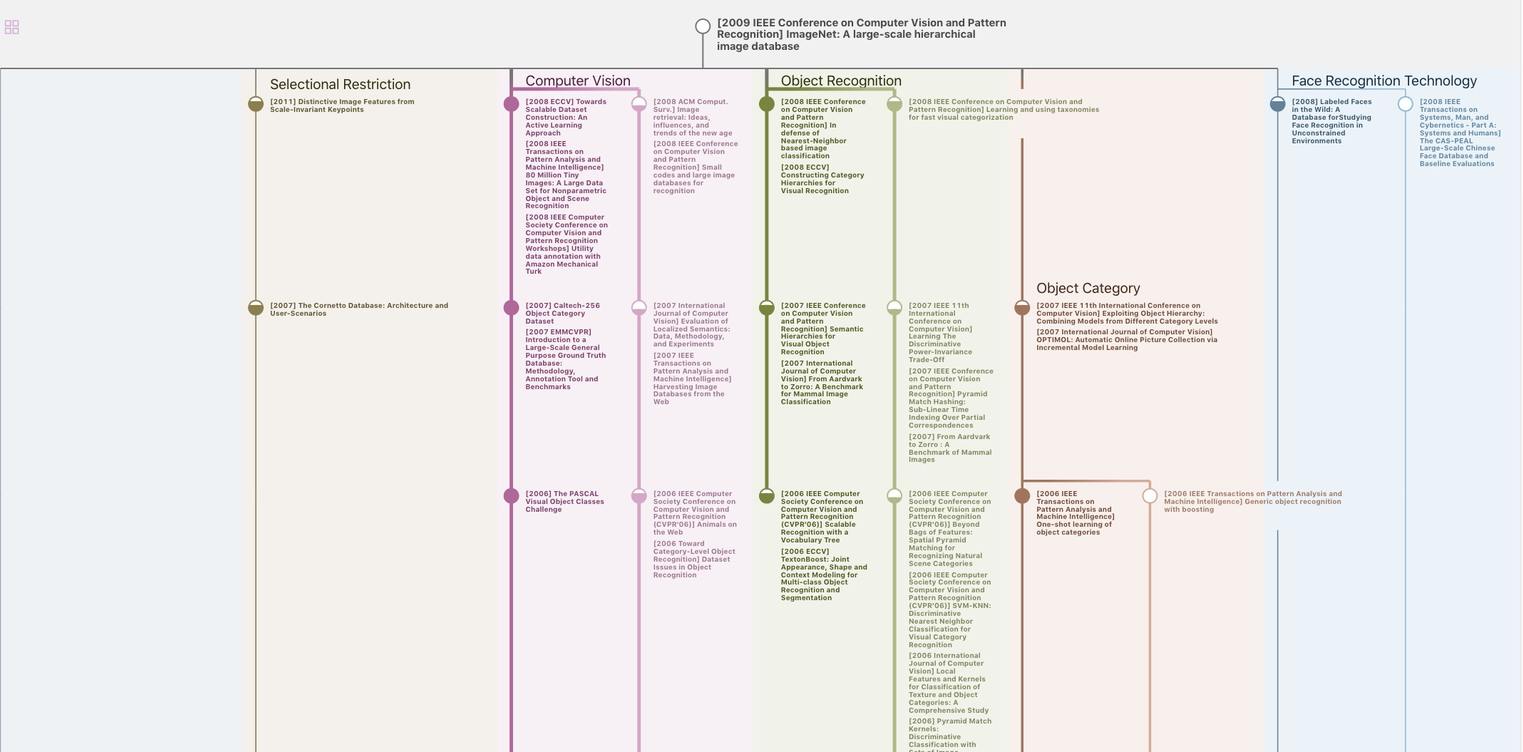
生成溯源树,研究论文发展脉络
Chat Paper
正在生成论文摘要