Reinvestigating the performance of artificial intelligence classification algorithms on COVID-19 X-Ray and CT images.
iScience(2024)
摘要
There are concerns that artificial intelligence (AI) algorithms may create underdiagnosis bias by mislabeling patient individuals with certain attributes (e.g., female and young) as healthy. Addressing this bias is crucial given the urgent need for AI diagnostics facing rapidly spreading infectious diseases like COVID-19. We find the prevalent AI diagnostic models show an underdiagnosis rate among specific patient populations, and the underdiagnosis rate is higher in some intersectional specific patient populations (for example, females aged 20-40 years). Additionally, we find training AI models on heterogeneous datasets (positive and negative samples from different datasets) may lead to poor model generalization. The model's classification performance varies significantly across test sets, with the accuracy of the better performance being over 40% higher than that of the poor performance. In conclusion, we developed an AI bias analysis pipeline to help researchers recognize and address biases that impact medical equality and ethics.
更多查看译文
关键词
Health informatics,Microbiology,Artificial intelligence applications
AI 理解论文
溯源树
样例
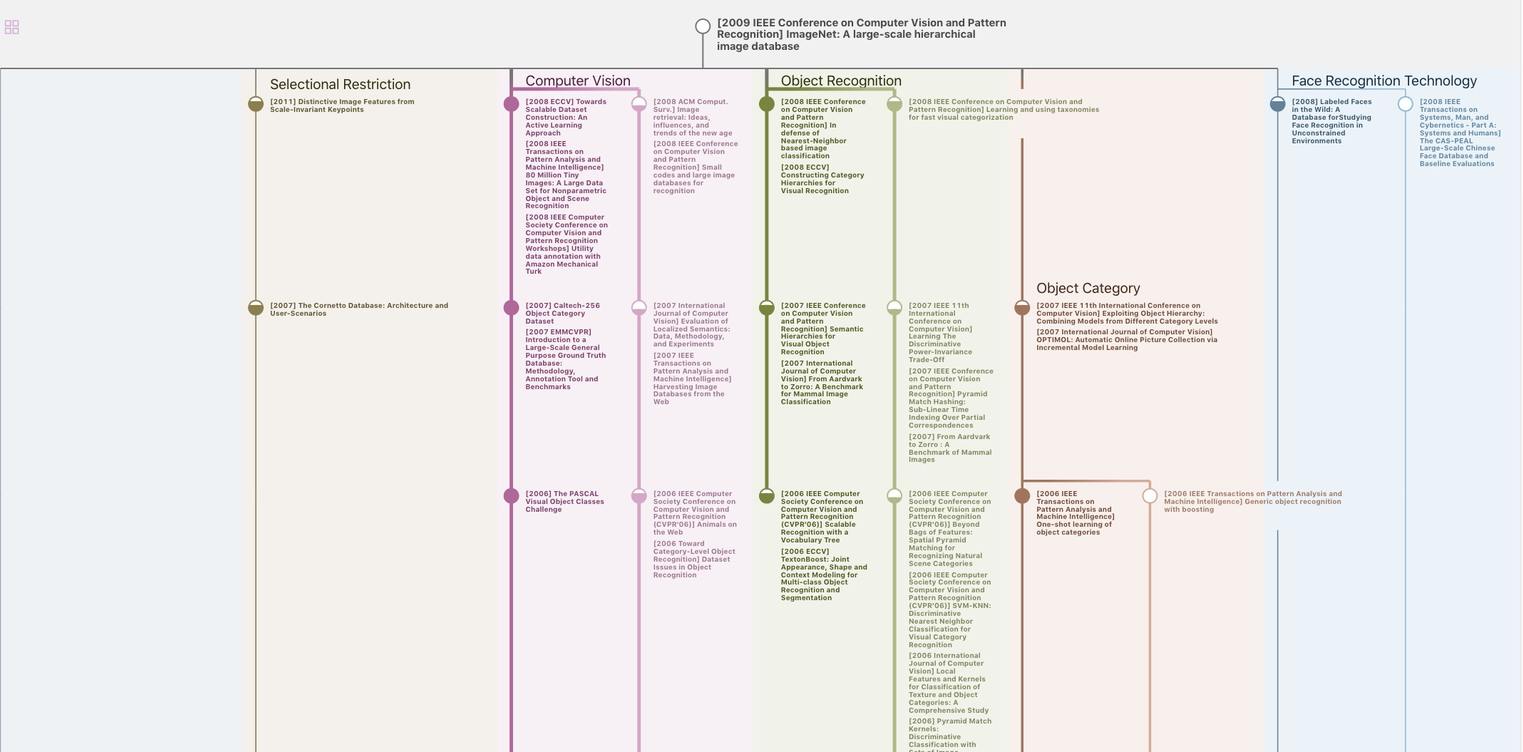
生成溯源树,研究论文发展脉络
Chat Paper
正在生成论文摘要