Perception field based imitation learning for unlabeled multi-agent pathfinding
Science China Information Sciences(2024)
摘要
This paper proposes an imitation learning method to learn a universal agent policy for unlabeled multi-agent pathfinding (unlabeled MAPF) in grid environments. The method transforms the unlabeled MAPF problem into a series of temporal-independent homogeneous classification problems for each agent. Based on this transformation, a neural network is designed to imitate a distance-optimal expert algorithm. The neural network consists of two successive modules: perception field learner and field integrating classifier. The former refines and encodes the current system state into a perception field for each agent by combining a set of learnable field-generating functions. The latter takes an agent’s perception field as input and decides the agent’s next action based on a triplet cross-attention mechanism. We evaluate our method on a diverse set of unlabeled MAPF tasks. Compared with state-of-the-art counterparts, the experimental results manifest the superiority of the proposed method in both generalization ability and scalability.
更多查看译文
关键词
unlabeled multi-agent pathfinding,perception field,triplet cross-attention,multi-agent imitation learning,learning-based planning
AI 理解论文
溯源树
样例
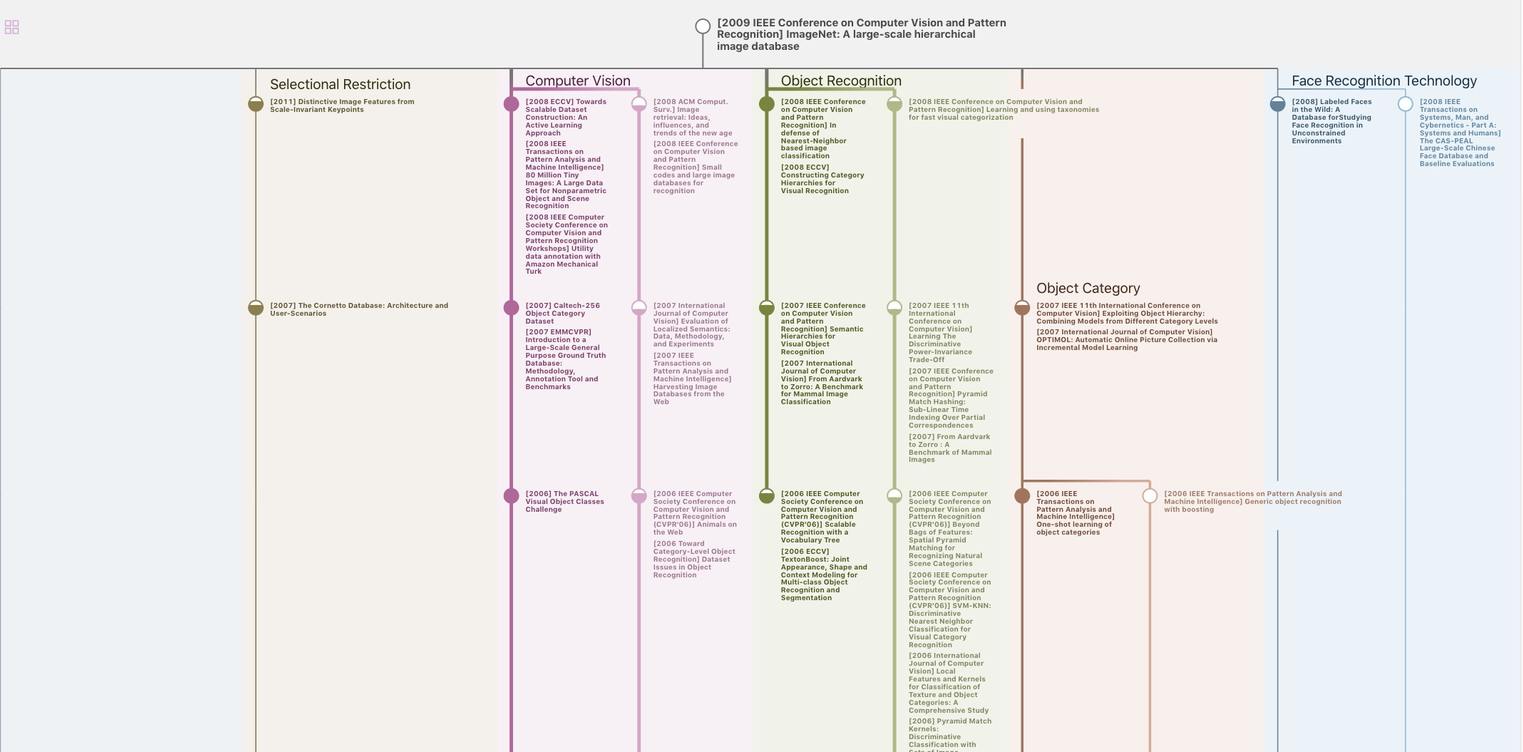
生成溯源树,研究论文发展脉络
Chat Paper
正在生成论文摘要