A flow-based multi-scale learning network for single image stochastic super-resolution
Signal Processing: Image Communication(2024)
摘要
Single image super-resolution (SISR) is still an important while challenging task. Existing methods usually ignore the diversity of generated Super-Resolution (SR) images. The fine details of the corresponding high-resolution (HR) images cannot be confidently recovered due to the degradation of detail in low-resolution (LR) images. To address the above issue, this paper presents a flow-based multi-scale learning network (FMLnet) to explore the diverse mapping spaces for SR. First, we propose a multi-scale learning block (MLB) to extract the underlying features of the LR image. Second, the introduced pixel-wise multi-head attention allows our model to map multiple representation subspaces simultaneously. Third, by employing a normalizing flow module for a given LR input, our approach generates various stochastic SR outputs with high visual quality. The trade-off between fidelity and perceptual quality can be controlled. Finally, the experimental results on five datasets demonstrate that the proposed network outperforms the existing methods in terms of diversity, and achieves competitive PSNR/SSIM results. Code is available at https://github.com/qianyuwu/FMLnet.
更多查看译文
关键词
Single image super-resolution,Attention mechanism,Normalizing flow,Stochastic super-resolution
AI 理解论文
溯源树
样例
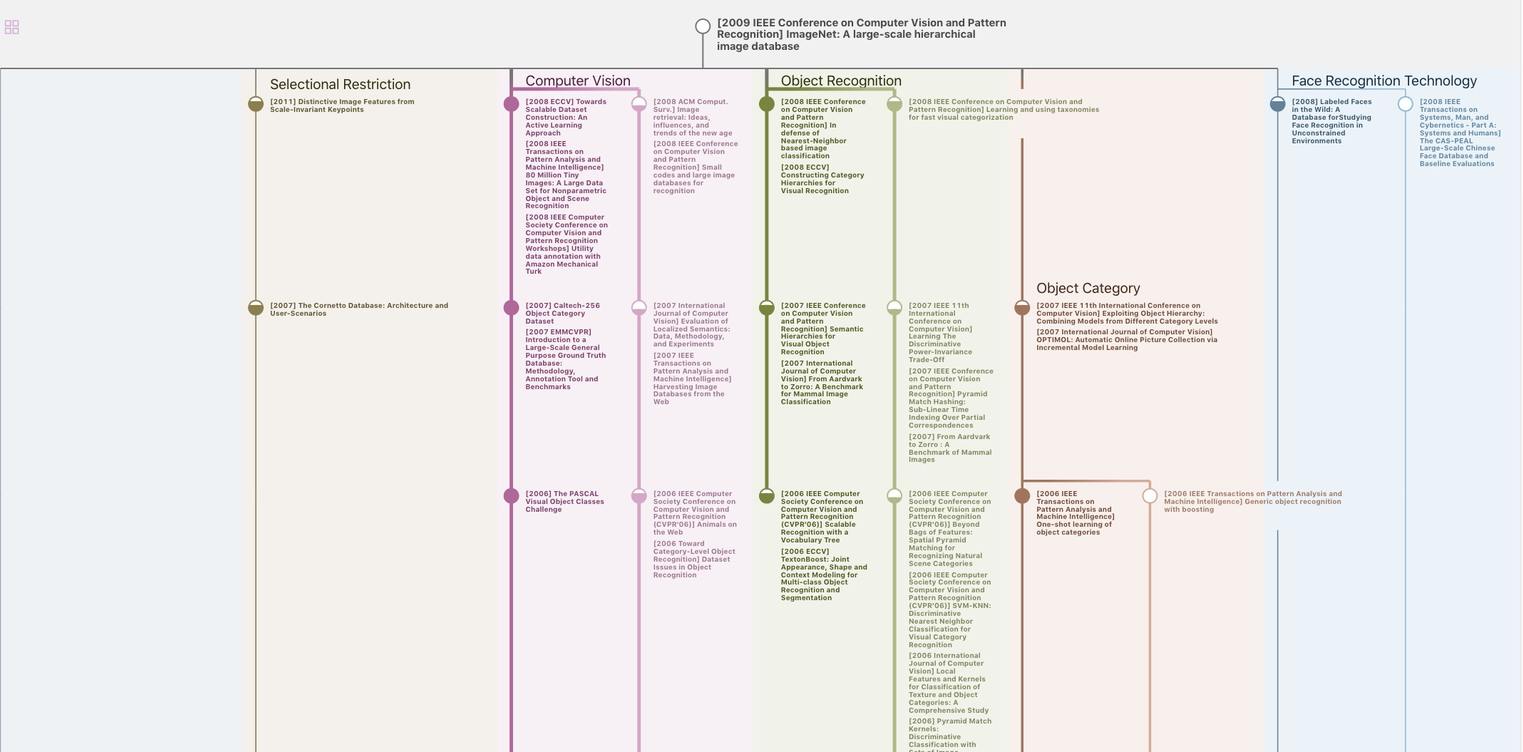
生成溯源树,研究论文发展脉络
Chat Paper
正在生成论文摘要