WCAM: Wavelet Convolutional Attention Module
SoutheastCon 2024(2024)
摘要
Convolutional neural networks (CNNs) have demon-strated remarkable ability in extracting and comprehending complex features from inputs, such as images. However, the conventional CNN approach of uniformly processing the entire image may not always be efficient, as not all input regions are equally significant for prediction. This aligns with the human visual system, which naturally prioritizes specific portions of a scene over processing its entirety simultaneously. Similarly, the attention mechanism emphasizes the most crucial aspects of a feature while suppressing less significant elements. This work introduces the Wavelet Convolutional Attention Module ((WCAM), which leverages the intrinsic properties of wavelets, such as multiresolution and sparsity, to direct the CNN towards more efficient and discriminative feature extraction and learning. The lossless property of wavelets enables efficient downsam- pling while preserving the details. WCAM is a versatile and lightweight module, easily integrated into any CNN without adding computational complexity. Extensive image classification and object detection experiments have been conducted using various datasets, including CIFAR-10, CIFAR-100, ImageNet-1k, and MS COCO. The results from these experiments have validated the effectiveness of WCAM over existing convolutional attention modules. The code will be released soon.
更多查看译文
关键词
Attention mechanism,Convolution attention,Discrete wavelet transform,Inverse wavelet transform,Feature extraction,Object recognition
AI 理解论文
溯源树
样例
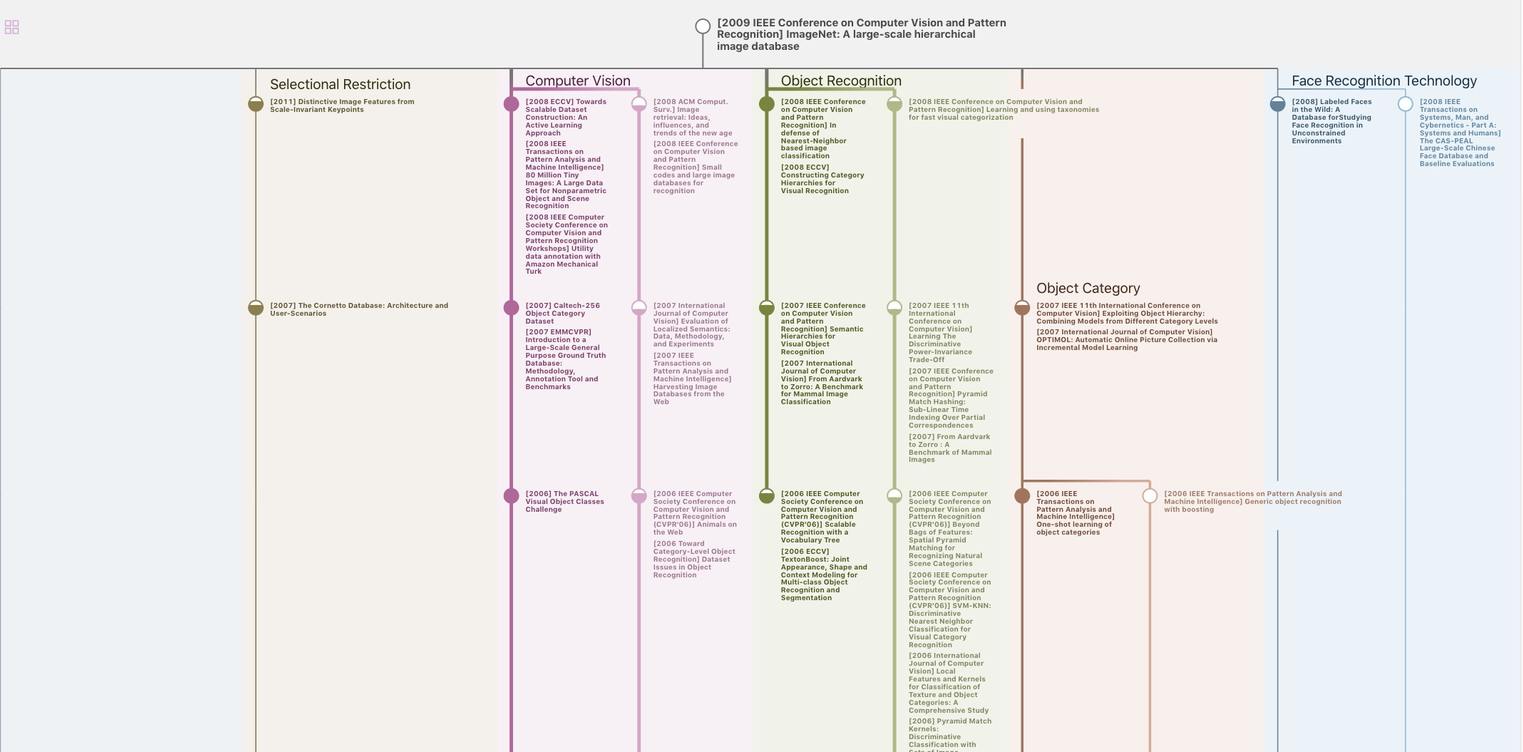
生成溯源树,研究论文发展脉络
Chat Paper
正在生成论文摘要