A Joint Learning Framework for Optimal Feature Extraction and Multi-class SVM
Information Sciences(2024)
摘要
In high-dimensional data classification, effectively extracting discriminative features while eliminating redundancy is crucial for enhancing the performances of classifiers, such as Support Vector Machine (SVM). However, previous studies have decoupled the process of feature extraction from the development of SVM, leading to suboptimal classification accuracy. To address this problem, we propose a novel joint learning framework that combines optimal feature extraction and multi-class SVM, incorporating a generalized regression form to learn a discriminative latent subspace. The projected data in this subspace are more likely to have a larger margin between different classes and align with the properties of the SVM classification mechanism, enhancing the overall classification performance. Three iterative algorithms were presented to obtain optimal solutions with guaranteed convergence, and theoretical analyses were also conducted to reveal their fundamental nature. The optimal linear projection subspace is equivalent to that obtained from Linear Discriminant Analysis (LDA) in some special cases. We conducted extensive experiments using diverse datasets to evaluate the performances of the proposed algorithms. Our algorithms achieved an accuracy improvement of up to 7.55% compared to other conventional methods.
更多查看译文
关键词
Feature extraction and selection,Pattern recognition,Linear discriminant analysis,SVM classification
AI 理解论文
溯源树
样例
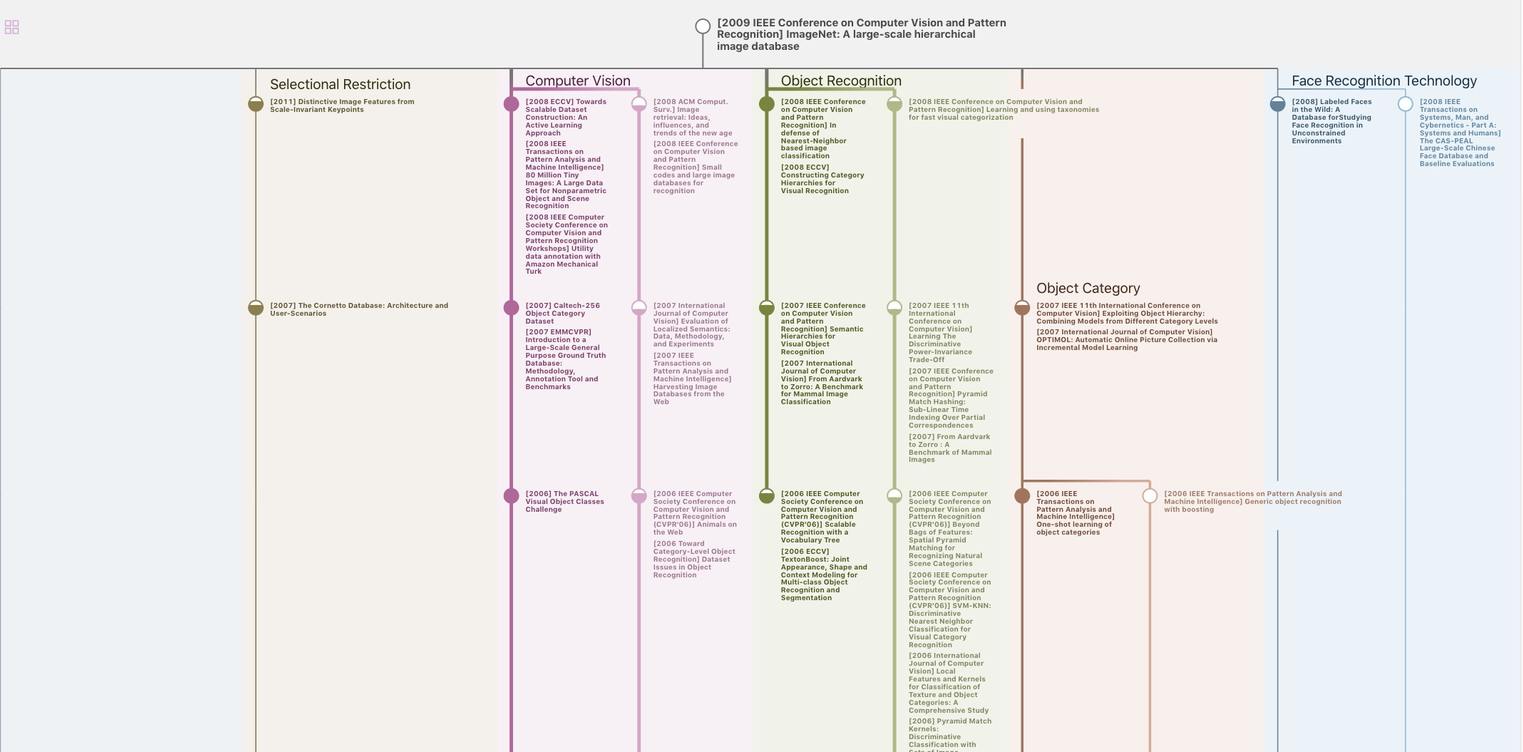
生成溯源树,研究论文发展脉络
Chat Paper
正在生成论文摘要