Weakly Supervised Semantic Segmentation with Consistency-Constrained Multi-Class Attention for Remote Sensing Scenes
IEEE Transactions on Geoscience and Remote Sensing(2024)
摘要
Obtaining image-level class labels for Remote Sensing (RS) images is a relatively straightforward process, sparking significant interest in Weakly Supervised Semantic Segmentation (WSSS). However, RS images present challenges beyond those encountered in generic WSSS, including complex backgrounds, densely distributed small objects, and considerable scale variations. To address above issues, we introduce a COnsistency-COnstrained Multi-Class Attention model, noted as
CocoaNet
. Specifically, CocoaNet endeavors to capture both semantic correlation and class distinctiveness using a Global-Local Adaptive Attention mechanism, which integrates the self-attention to model global correlation, complemented by a Local Perception branch that intensifies focus on local regions. The resulting class-specific attention weights and patch-level pairwise affinity weights are employed to optimize the initial CAMs. This mechanism proves highly effective in mitigating inter-class interference and managing the distribution of densely clustered small objects. Moreover, we invoke a Consistency Constraint to rectify activation inaccuracy. By utilizing a Siamese structure for the mutual supervision of features extracted from images at different scales, we address substantial scale variations in RS scenes. Simultaneously, a Class Contrast Loss is adopted to enhance the discriminativeness of class-specific features. Departing from the conventional CAM optimization, which is rather complex and time-consuming, we harness the prior knowledge from generic Segment Anything model to design a joint optimization strategy that refines target boundaries and further promotes discriminative visual features. We validate the effectiveness of our proposed approach on three benchmark datasets in multi-class RS scenarios, experimental results demonstrate that our model yield promising advancements compared to state-of-the-art methods.
更多查看译文
关键词
Global-Local Adaptive Attention,Consistency Constraint,Weakly Supervised Semantic Segmentation
AI 理解论文
溯源树
样例
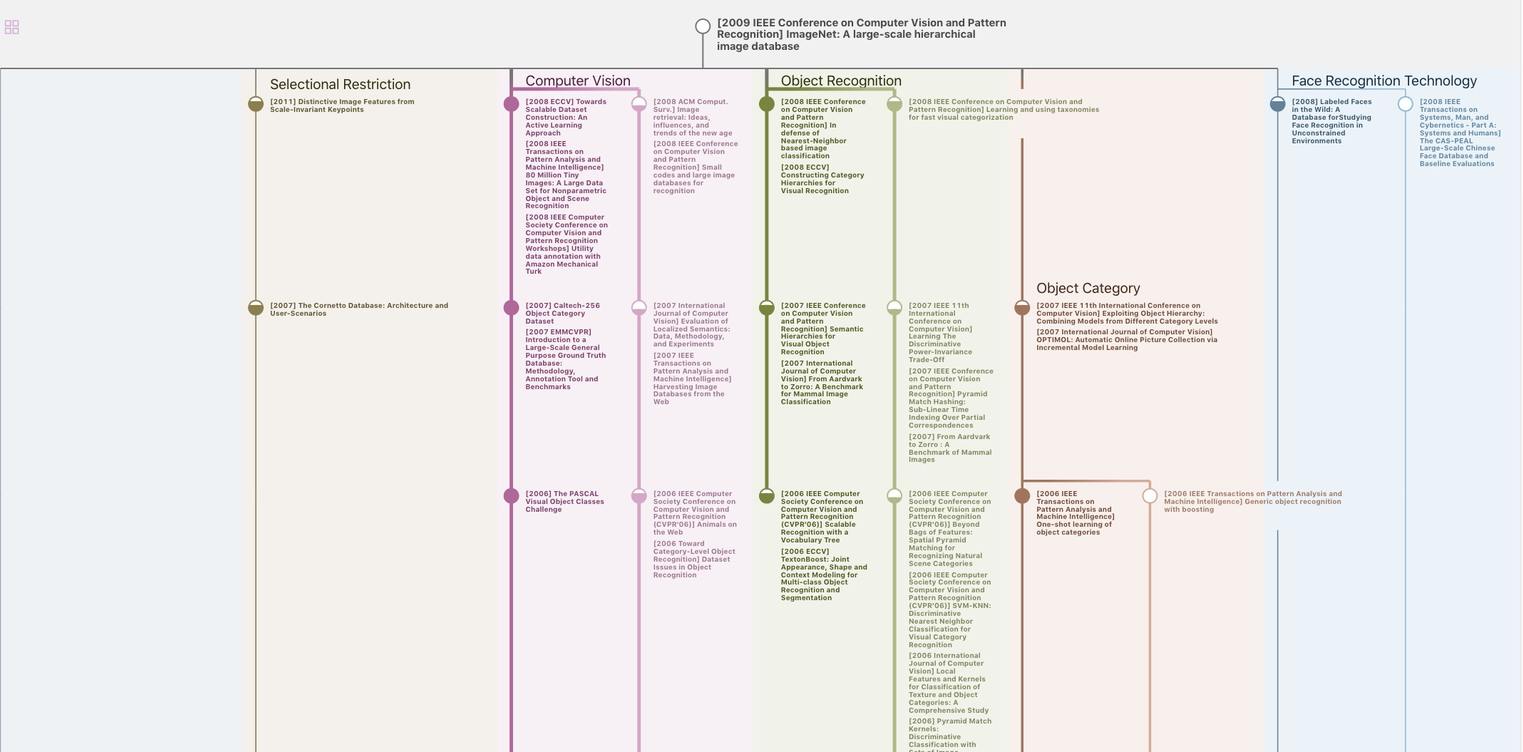
生成溯源树,研究论文发展脉络
Chat Paper
正在生成论文摘要