Multiobjective Evolutionary Learning for Multitask Quality Prediction Problems in Continuous Annealing Process
IEEE TRANSACTIONS ON NEURAL NETWORKS AND LEARNING SYSTEMS(2024)
摘要
In industrial production processes, the mechanical properties of materials will directly determine the stability and consistency of product quality. However, detecting the current mechanical property is time-consuming and labor-intensive, and the material quality cannot be controlled in time. To achieve high-quality steel materials, developing a novel intelligent manufacturing technology that can satisfy multitask predictions for material properties has become a new research trend. This article proposes a multiobjective evolutionary learning method based on a two-stage model with topological sparse autoencoder (TSAE) and ensemble learning. For the structure characteristics of a typical autoencoder (AE), a topology-related constraint is incorporated into the loss function of the AE, thus maintaining the global relationship among multistage input data to improve the data reconstruction quality. Then, a sparse representation of the data is added to the AE to achieve dimensionality reduction. Moreover, the extreme gradient boosting (XGBoost) method is applied to predict the mechanical properties of steel materials through collaboration learning mechanisms. To enhance the model accuracy, a multiobjective evolutionary algorithm (MOEA) with a knee solution strategy is used to optimize the network structure and hyperparameters of the two-stage model. Experiments are conducted using real steel production data from a continuous annealing process (CAP). The results verify that the proposed method obtains a higher prediction accuracy than other state-of-the-art methods and can guide practical production and new material design.
更多查看译文
关键词
Steel,Predictive models,Production,Data models,Analytical models,Optimization,Mechanical factors,Continuous annealing process (CAP),evolutionary learning,multiobjective optimization (MO),multitask quality prediction,topological sparse autoencoder (TSAE)
AI 理解论文
溯源树
样例
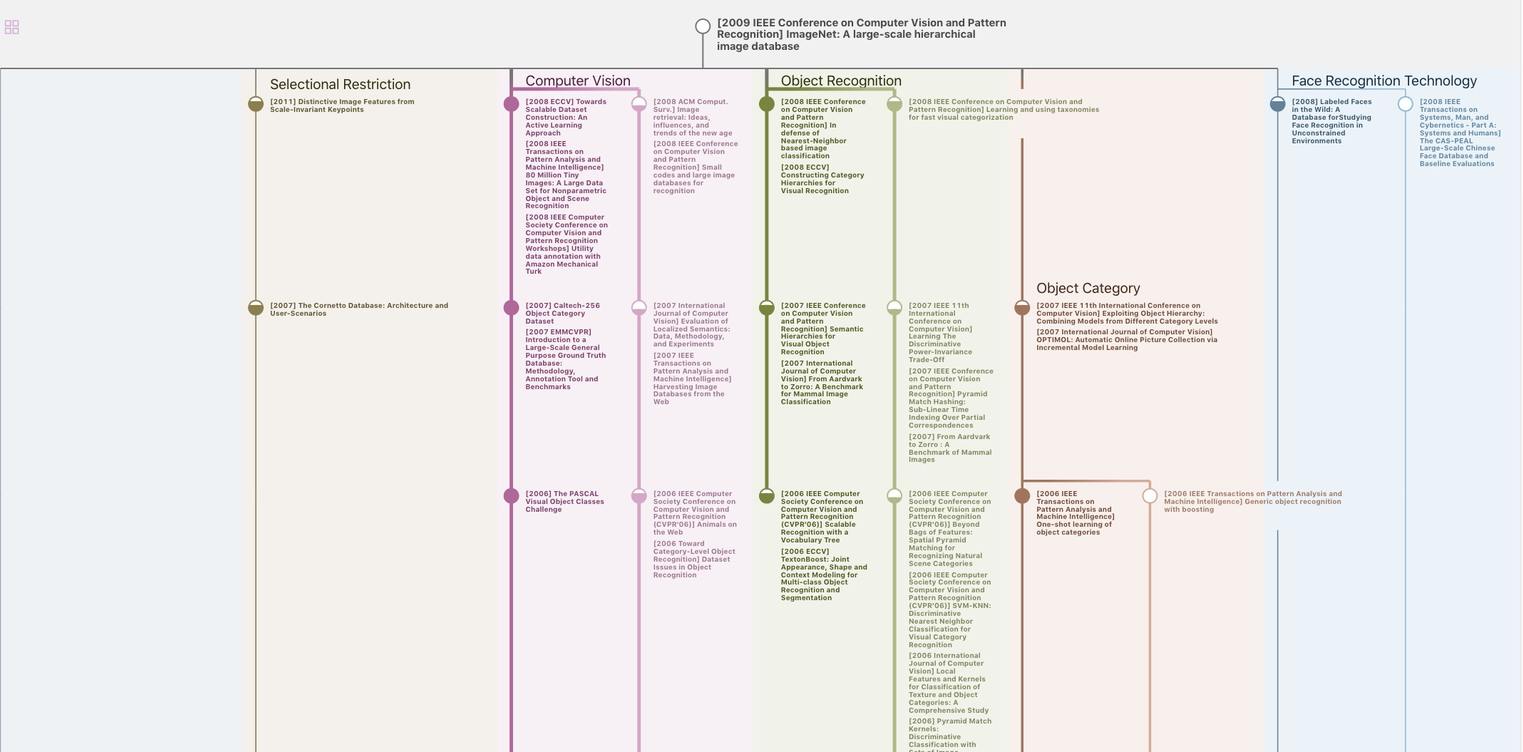
生成溯源树,研究论文发展脉络
Chat Paper
正在生成论文摘要