ESIE-BERT: Enriching sub-words information explicitly with BERT for intent classification and slot filling
Neurocomputing(2024)
摘要
Natural language understanding (NLU) has two core tasks: intent classification and slot filling. The success of pre-training language models resulted in a significant breakthrough in the two tasks. The architecture based on autoencoding (BERT-based model) can optimize the two tasks jointly. However, we note that BERT-based models convert each complex token into multiple sub-tokens by the Wordpiece algorithm, which generates an out-of-alignment between the lengths of the tokens and the labels. This leads to BERT-based models not performing well in label prediction, which limits the improvement of model performance. Many existing models can address this issue, but some hidden semantic information is discarded during the fine-tuning process. We addressed the problem by introducing a novel joint method on top of BERT. This method explicitly models multiple sub-token features after the Wordpiece tokenization, thereby contributing to both tasks. Our proposed method effectively extracts contextual features from complex tokens using the Sub-words Attention Adapter (SAA), preserving overall utterance information. Additionally, we propose an Intent Attention Adapter (IAA) to acquire comprehensive sentence features, assisting users in predicting intent. Experimental results confirm that our proposed model exhibits significant improvements on two public benchmark datasets. Specifically, the slot-filling F1 score improves from 96.5 to 98.2 (an absolute increase of 1.7%) on the Airline Travel Information Systems (ATIS) dataset.
更多查看译文
关键词
Wordpiece,Intent classification,Slot filling,BERT,Attention mechanisms
AI 理解论文
溯源树
样例
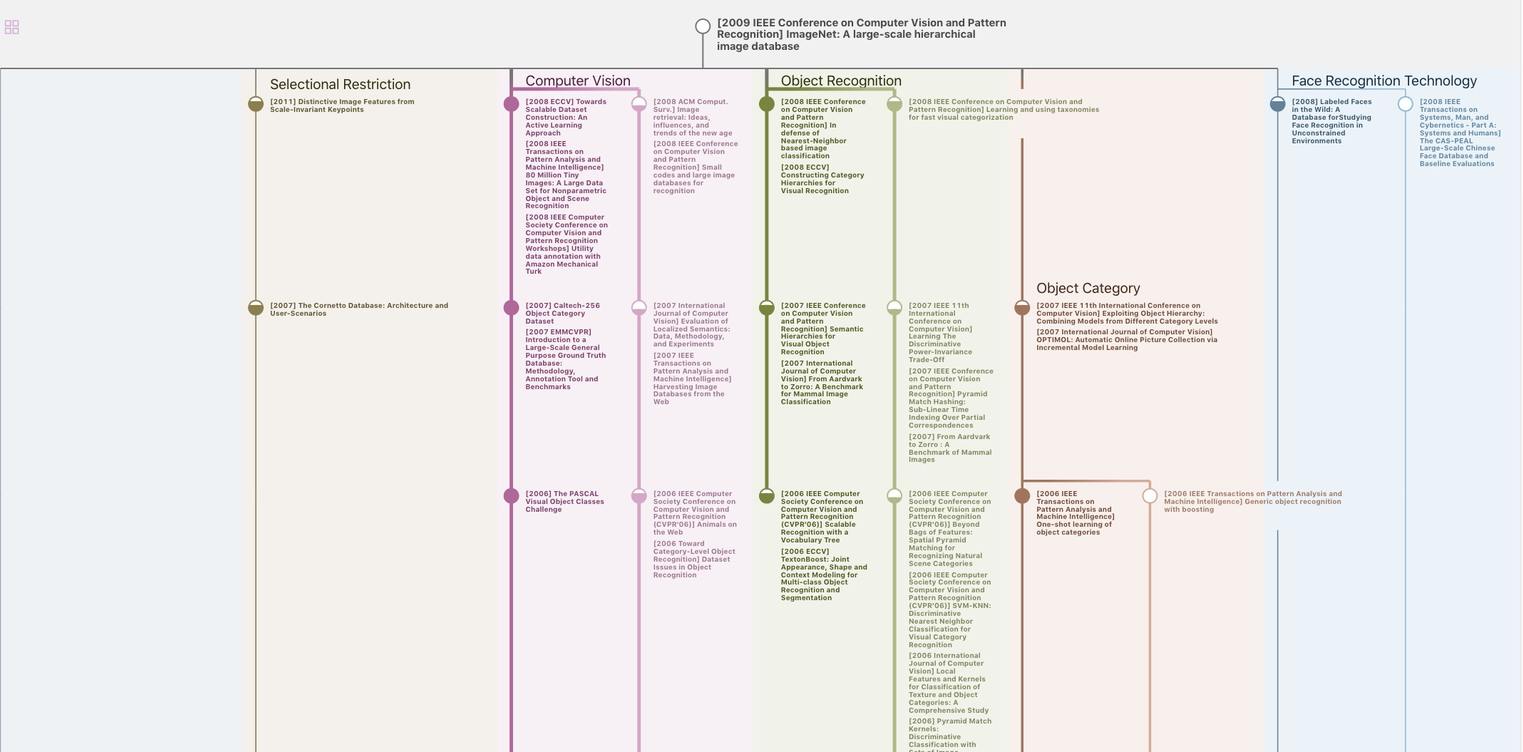
生成溯源树,研究论文发展脉络
Chat Paper
正在生成论文摘要