Investigating the potential of EMA-embedded feature selection method for ESVR and LSTM to enhance the robustness of monthly streamflow forecasting from local meteorological information
Journal of Hydrology(2024)
摘要
Accurate forecast of monthly streamflow is helpful to improve the social capability in risk management. Diverse input features are crucial to the accuracy of machine learning-based monthly streamflow forecast models. The embedded feature selection (EFS) method based on binary-coded metaheuristic algorithm is adept at parallel optimization of feature selection and model hyperparameter. It has been rarely studied coupled with deep learning models in monthly streamflow forecasting. This study further investigated the potential of EFS method using the well-known Long short-term memory network (LSTM) and a state-of-the-art model named Extreme support vector regression (ESVR), with the Evolutionary mating algorithm (EMA) as the search algorithm. The benchmark models were established using EMA for hyperparameter optimization without feature selection. Ten basins with distinct hydroclimate characteristics minimally impacted by human activities from the Catchment Attributes and Meteorology for Large-sample Studies (CAMELS) data set were used to comprehensively examine the proposed models. Nonparametric approaches including the Friedman test and Wilcoxon signed ranks test were utilized to rank the models and detect the significance of the performance variation brought by EFS. The kernel ESVR model coupled with EFS-EMA (EFS-KESVREMA) ranked the first in the 1-month-ahead experiment with NSE = 0.734–0.944. EFS-ESVREMA and EFS-LSTMEMA ranked second and third, with NSE = 0.746–0.94 and NSE = 0.701–0.94 respectively. The results also demonstrated that EFS-EMA helps the benchmark models improve prediction accuracy at a confidence level of 95 % but it has no obvious effect on the standard SVR. As the forecast horizon increased to 3- and 5-month-ahead, EFS-ESVREMA maintained a reliable performance. Whereas EFS-LSTMEMA experienced a significant accuracy decrease in the 5-month-ahead experiment. The proposed models and relevant findings in this study have certain reference value for researchers in monthly streamflow forecasting.
更多查看译文
关键词
Monthly streamflow forecasting,Embedded feature selection,Evolutionary mating algorithm,Extreme support vector regression,LSTM,CAMELS
AI 理解论文
溯源树
样例
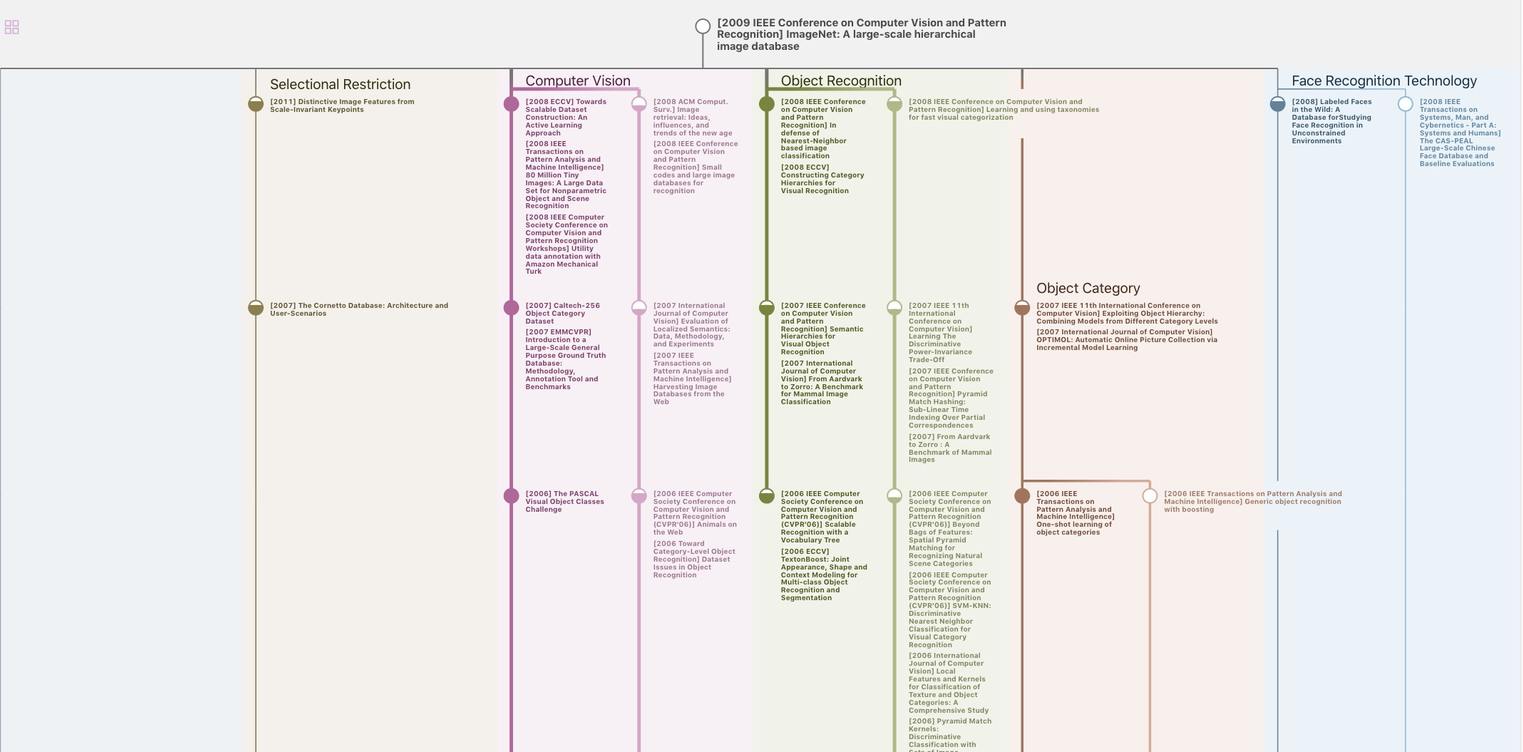
生成溯源树,研究论文发展脉络
Chat Paper
正在生成论文摘要