Localization-aware logit mimicking for object detection in adverse weather conditions
Image and Vision Computing(2024)
摘要
Adverse weather conditions would decrease the image quality, leading to a sharp decline in detection accuracy. Most of the researches focus on object detection in fine weather conditions, rather than in adverse weather conditions. Recently, some methods attempt to reduce the gap between degraded images and clean images to improve the detection accuracy in adverse weather conditions. Specifically, these methods usually conduct image restoration and object detection in a sequential way or by joint learning. While these methods can improve detection accuracy to a certain extent, image restoration models may introduce noise or artifacts and increase computational burden, limiting the accuracy and efficiency of object detection in adverse weather conditions. In this paper, we propose a knowledge distillation-based method, Localization-aware Logit Mimicking (LLM), to improve detection accuracy in adverse weather conditions by reducing the gap between degraded images and clean images at the prediction level, rather than the image level. Moreover, the localization quality is designed as the mimicking target to make the knowledge distillation more effective. Experiments conducted on three popular benchmarks (i.e., RTTS, ExDark, and RID) demonstrate that our LLM can achieve the state-of-the-art detection accuracy and inference speed in foggy, rainy, and low-light conditions.
更多查看译文
关键词
Object detection,Adverse weather conditions,Knowledge distillation
AI 理解论文
溯源树
样例
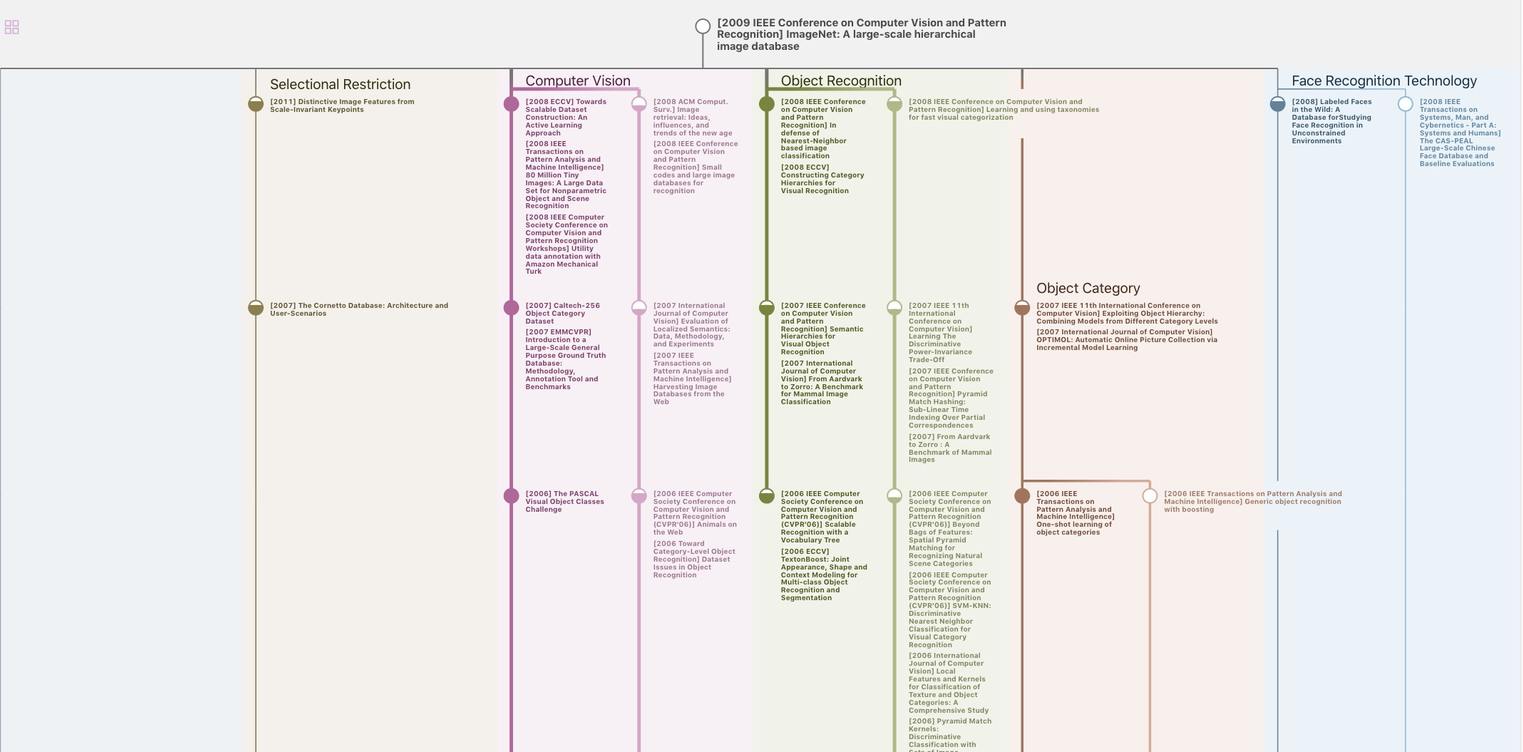
生成溯源树,研究论文发展脉络
Chat Paper
正在生成论文摘要