FLARE: A New Federated Learning Framework with Adjustable Learning Rates over Resource-Constrained Wireless Networks
arxiv(2024)
摘要
Wireless federated learning (WFL) suffers from heterogeneity prevailing in the data distributions, computing powers, and channel conditions of participating devices. This paper presents a new Federated Learning with Adjusted leaRning ratE (FLARE) framework to mitigate the impact of the heterogeneity. The key idea is to allow the participating devices to adjust their individual learning rates and local training iterations, adapting to their instantaneous computing powers. The convergence upper bound of FLARE is established rigorously under a general setting with non-convex models in the presence of non-i.i.d. datasets and imbalanced computing powers. By minimizing the upper bound, we further optimize the scheduling of FLARE to exploit the channel heterogeneity. A nested problem structure is revealed to facilitate iteratively allocating the bandwidth with binary search and selecting devices with a new greedy method. A linear problem structure is also identified and a low-complexity linear programming scheduling policy is designed when training models have large Lipschitz constants. Experiments demonstrate that FLARE consistently outperforms the baselines in test accuracy, and converges much faster with the proposed scheduling policy.
更多查看译文
AI 理解论文
溯源树
样例
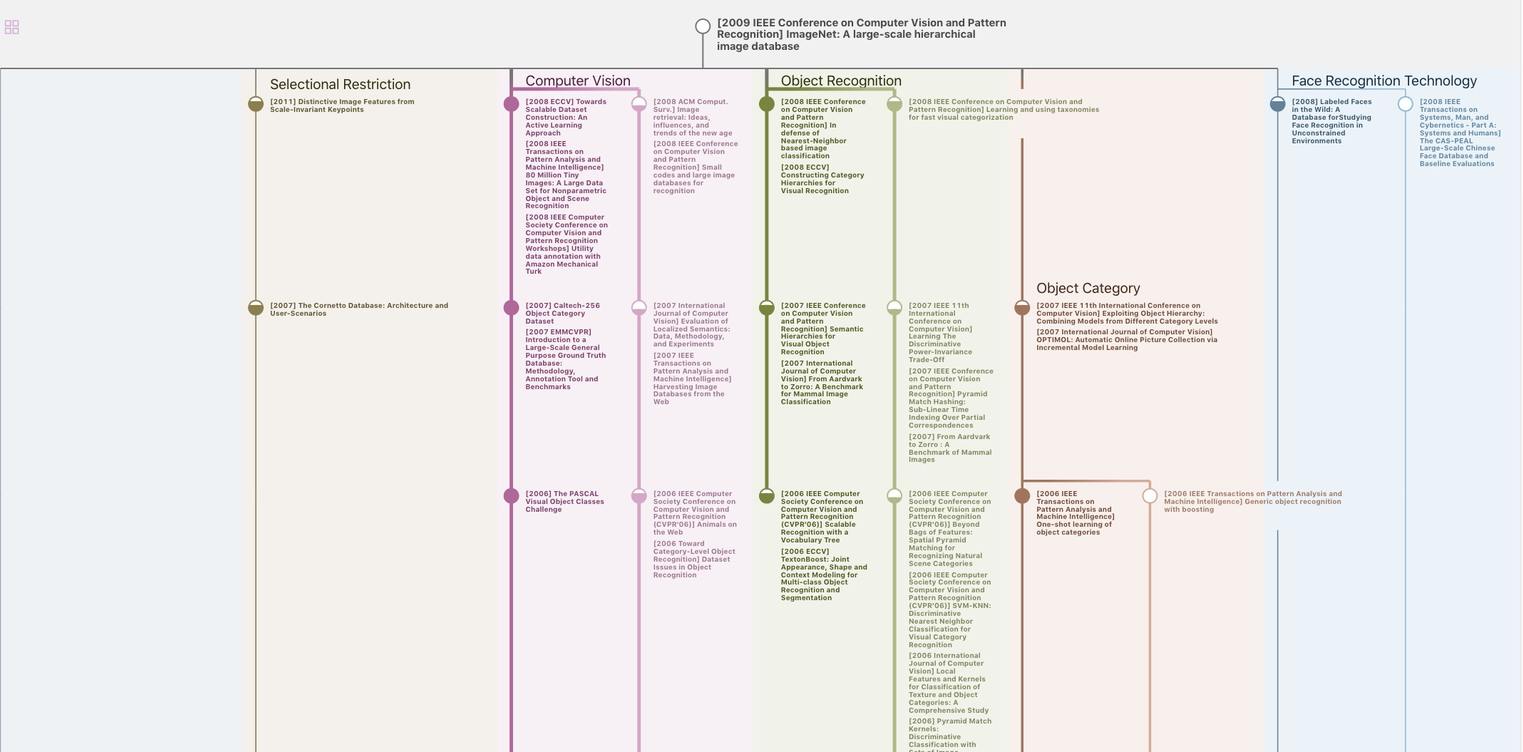
生成溯源树,研究论文发展脉络
Chat Paper
正在生成论文摘要