Enhancing Transparency in Transport Mode Detection: An Interpretable Ensemble Model Classifier
2024 International Conference on Advances in Computing, Communication, Electrical, and Smart Systems (iCACCESS)(2024)
摘要
Transport Mode Detection (TMD) is a crucial component in the field of Intelligent Transportation Systems (ITS), taking advantage of current advancements in Artificial Intelligence and the Internet of Things (IoT). This research undertakes a thorough investigation of transportation modalities, recognizing the crucial role of TMD in increasing road safety. The study evaluates established machine learning methodologies, such as LightGBM, XGBoost, and CatBoost. Furthermore, it introduces a novel ensemble model that capitalizes on the advantages of these techniques, resulting in higher precision when categorizing transportation modes. Notably, the ensemble model provides accuracies of 83%, 93%, and 94% across three independent sensor data collections from the TMD dataset, surpassing the performance of alternative machine learning classifiers. In addition, the incorporation of Shapley Additive Explanations (SHAP) and Local Interpretable Model-Agnostic Explanations (LIME) improves the comprehensibility of the model we propose, providing a valuable understanding of the decision-making procedures. This research not only enhances the current endeavors to improve road safety but also presents a promising method for the advancement of intelligent transportation systems.
更多查看译文
关键词
Machine Learning,Ensemble Model,LIME,SHAP,TMD Dataset
AI 理解论文
溯源树
样例
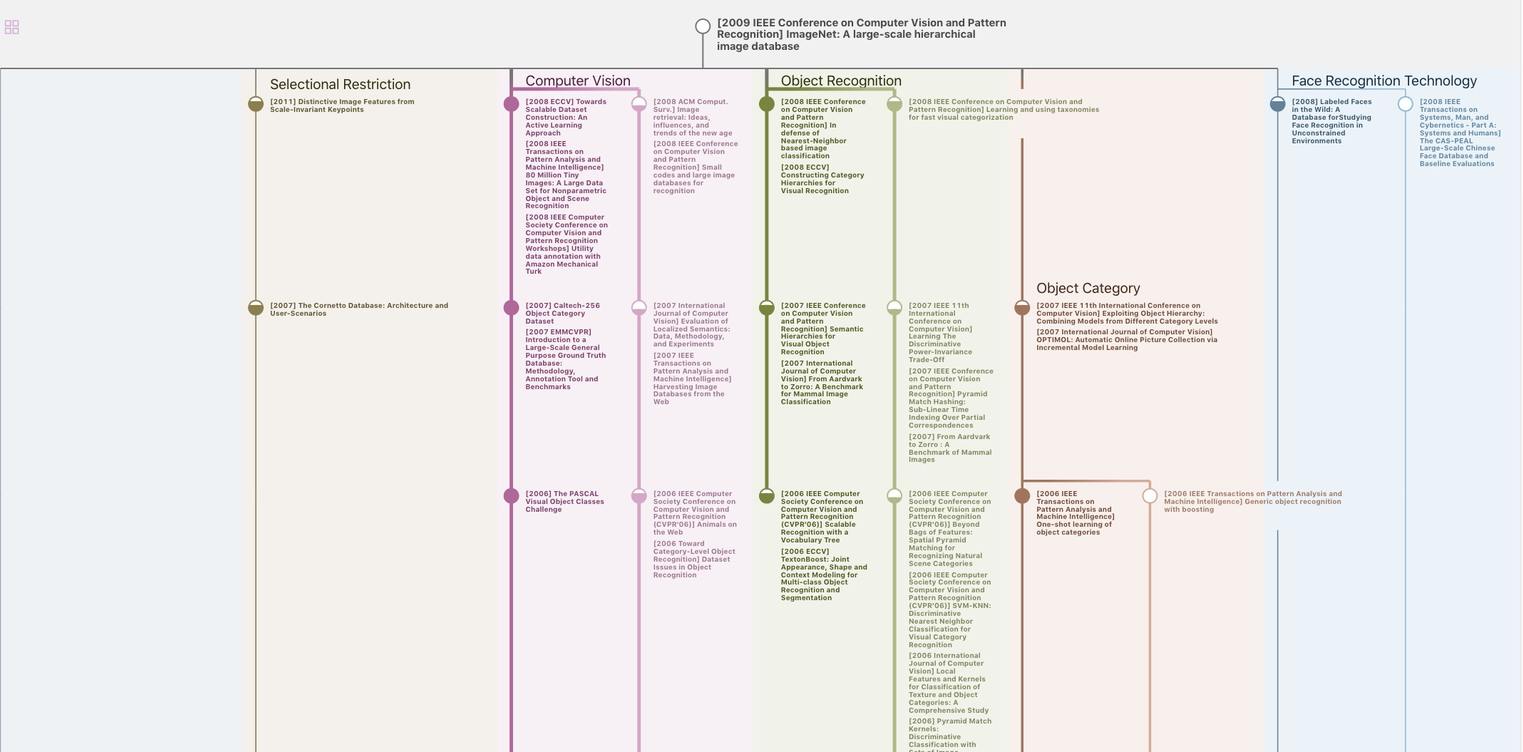
生成溯源树,研究论文发展脉络
Chat Paper
正在生成论文摘要