Trajectory Planning for UAV-Assisted Data Collection in IoT Network: A Double Deep Q Network Approach
Electronics(2024)
摘要
Unmanned aerial vehicles (UAVs) are becoming increasingly valuable as a new type of mobile communication device and autonomous decision-making device in many application areas, including the Internet of Things (IoT). UAVs have advantages over other stationary devices in terms of high flexibility. However, a UAV, as a mobile device, still faces some challenges in optimizing its trajectory for data collection. Firstly, the high complexity of the movement action and state space of the UAV’s 3D trajectory is not negligible. Secondly, in unknown urban environments, a UAV must avoid obstacles accurately in order to ensure a safe flight. Furthermore, without a priori wireless channel characterization and ground device locations, a UAV must reliably and safely complete the data collection from the ground devices under the threat of unknown interference. All of these require the proposing of intelligent and automatic onboard trajectory optimization techniques. This paper transforms the trajectory optimization problem into a Markov decision process (MDP), and deep reinforcement learning (DRL) is applied to the data collection scenario. Specifically, the double deep Q-network (DDQN) algorithm is designed to address intelligent UAV trajectory planning that enables energy-efficient and safe data collection. Compared with the traditional algorithm, the DDQN algorithm is much better than the traditional Q-Learning algorithm, and the training time of the network is shorter than that of the deep Q-network (DQN) algorithm.
更多查看译文
关键词
UAV,trajectory planning,deep reinforcement learning,double deep Q-network (DDQN)
AI 理解论文
溯源树
样例
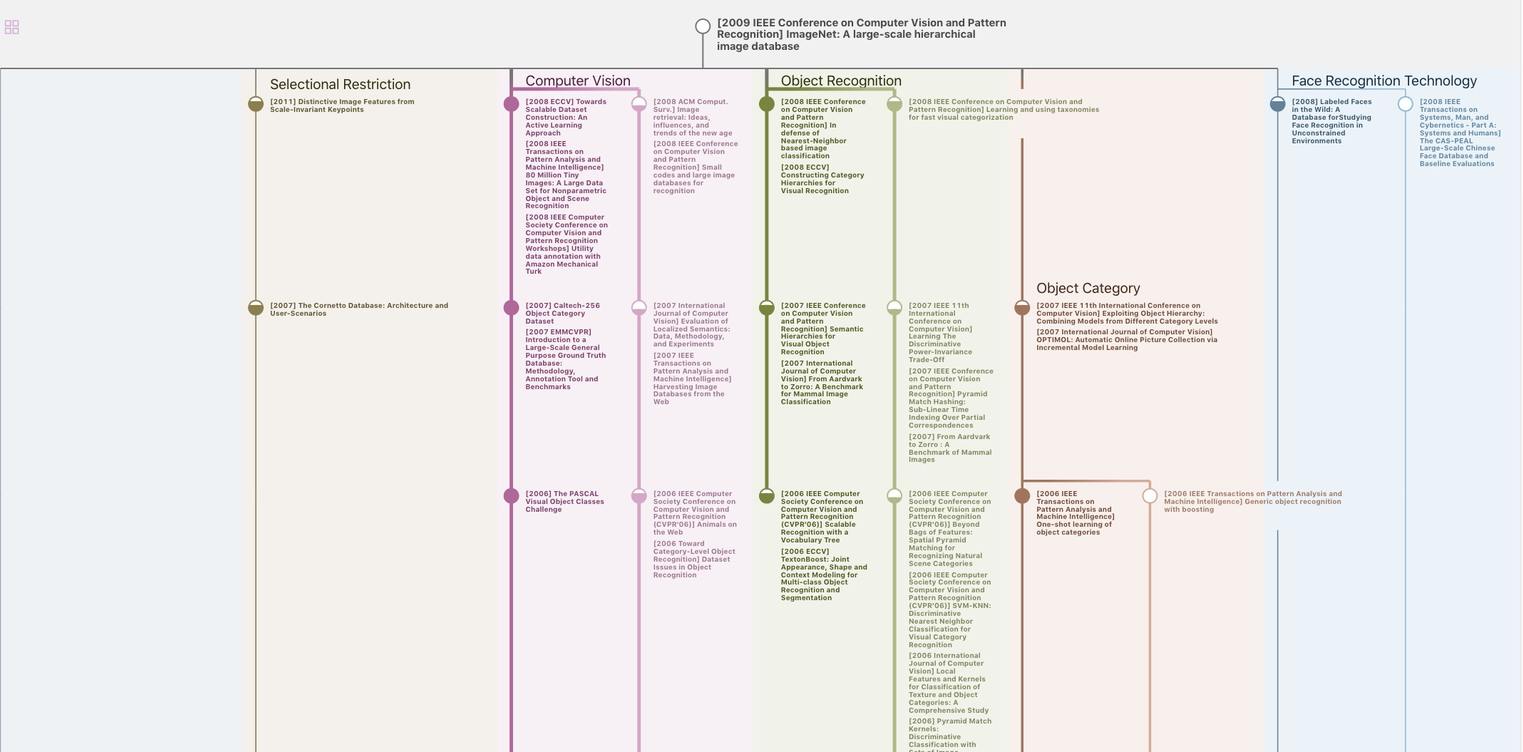
生成溯源树,研究论文发展脉络
Chat Paper
正在生成论文摘要