Consistency Framework For Zero-Shot Image Captioning
2024 4th International Conference on Neural Networks, Information and Communication (NNICE)(2024)
摘要
Recently, Large-scale pre-trained Visual Language Models (VLMs) have shown great zero-shot capability in various downstream tasks. Nonetheless, they are not capable of generating caption given an image. To explore the adaptation to zero-shot image-captioning, recent works follow the paradigm that utilize a pre-trained Large Language Models (LLMs) as the language decoder, then using the text embedding encoded by VLMs as a substitute for image. However, when predicting on images, model usually fails to correctly comprehend visual content, leading to the wrong prediction of objects that do not actually exist in the images, i.e., object hallucination. The cause of this phenomenon is that, during the training process, the model fails to adequately receive and integrate information from the image modality. This results in the crucial image-related information being missing during training. To address the above issue, in this work, we propose our Visual Augment Decoding Network (VAD) for zero-shot image captioning. We initially use retrieval model to search the relevant image from unpaired dataset, thus visual information is introduced during the training phase. Additionally, we employ an entity-aware textual prompt, guiding the LLMs to better comprehend image content. Experimental results demonstrate competitive performance across both in-domain and cross-domain captioning in multiple datasets, validating its generalization capabilities and superiority.
更多查看译文
关键词
component,Image Captioning,Zero-Shot,prompt engineersing
AI 理解论文
溯源树
样例
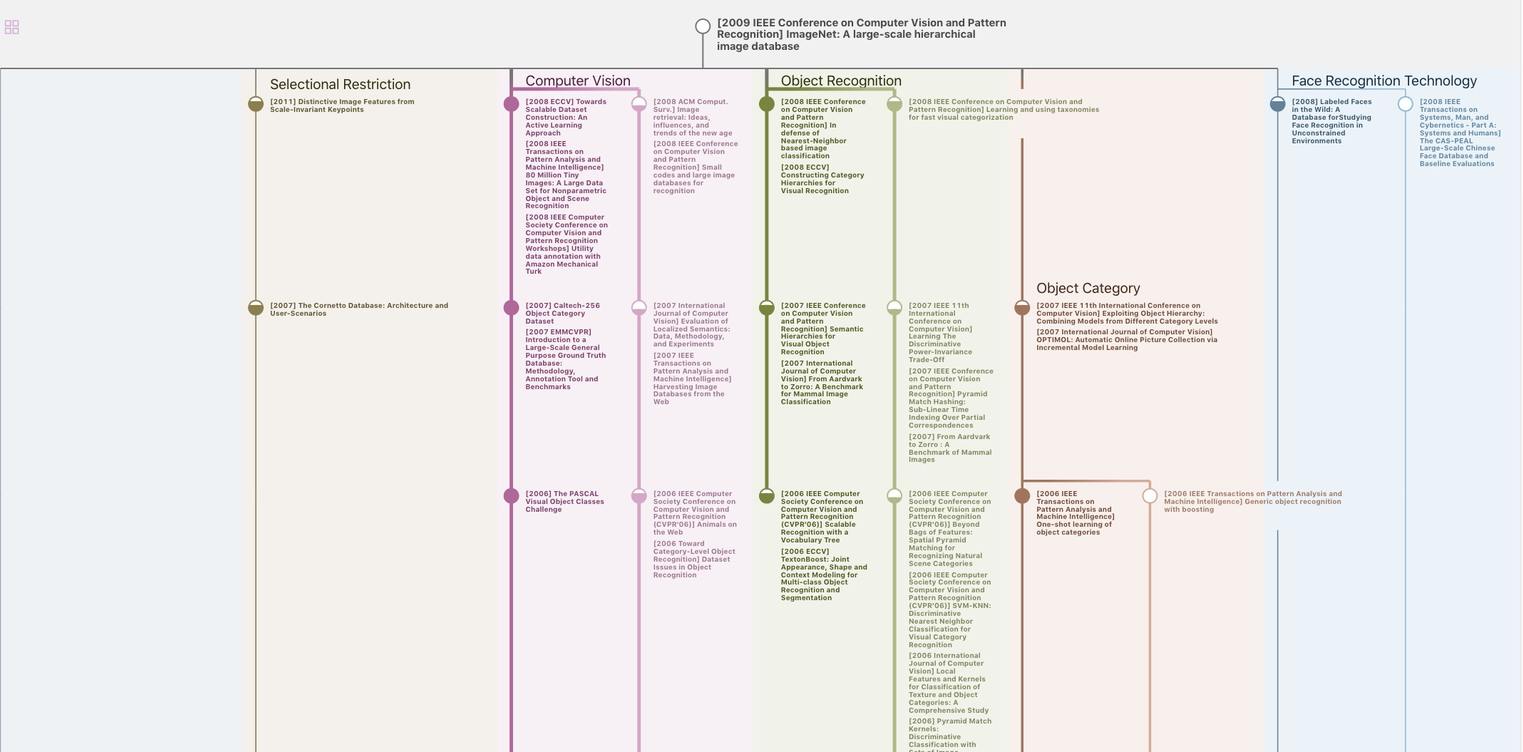
生成溯源树,研究论文发展脉络
Chat Paper
正在生成论文摘要